Identifying optimised speaker identification model using hybrid GRU-CNN feature extraction technique
International Journal of Computational Vision and Robotics(2022)
摘要
Extracting vigorous and discriminative features and selecting an appropriate classifier model to identify speakers from voice clips are challenging tasks. Thus, we considered signal processing techniques and deep neural networks for feature extraction along with state-of-art machine-learning models as classifiers. Also, we introduced a hybrid gated recurrent unit (GRU) and convolutional neural network (CNN) as a novel feature extractor for optimising the subspace loss to extract the best feature vector. Additionally, space-time is contemplated as a computational parameter for finding the optimal speaker identification pipeline. Later, we have inspected the pipeline in a large-scale VoxCeleb dataset comprising 6,000 real world speakers with multiple voices achieving GRU-CNN + R-CNN for the highest accuracy and F1-score as well as GRU-CNN + CNN for maximum precision and LPC + KNN for the highest recall. Also, LPCC + R-CNN and MFCC + R-CNN are accomplished as optimal in terms of memory usage and time respectively.
更多查看译文
关键词
computational complexity,deep learning,feature extraction,speaker identification,VoxCeleb dataset
AI 理解论文
溯源树
样例
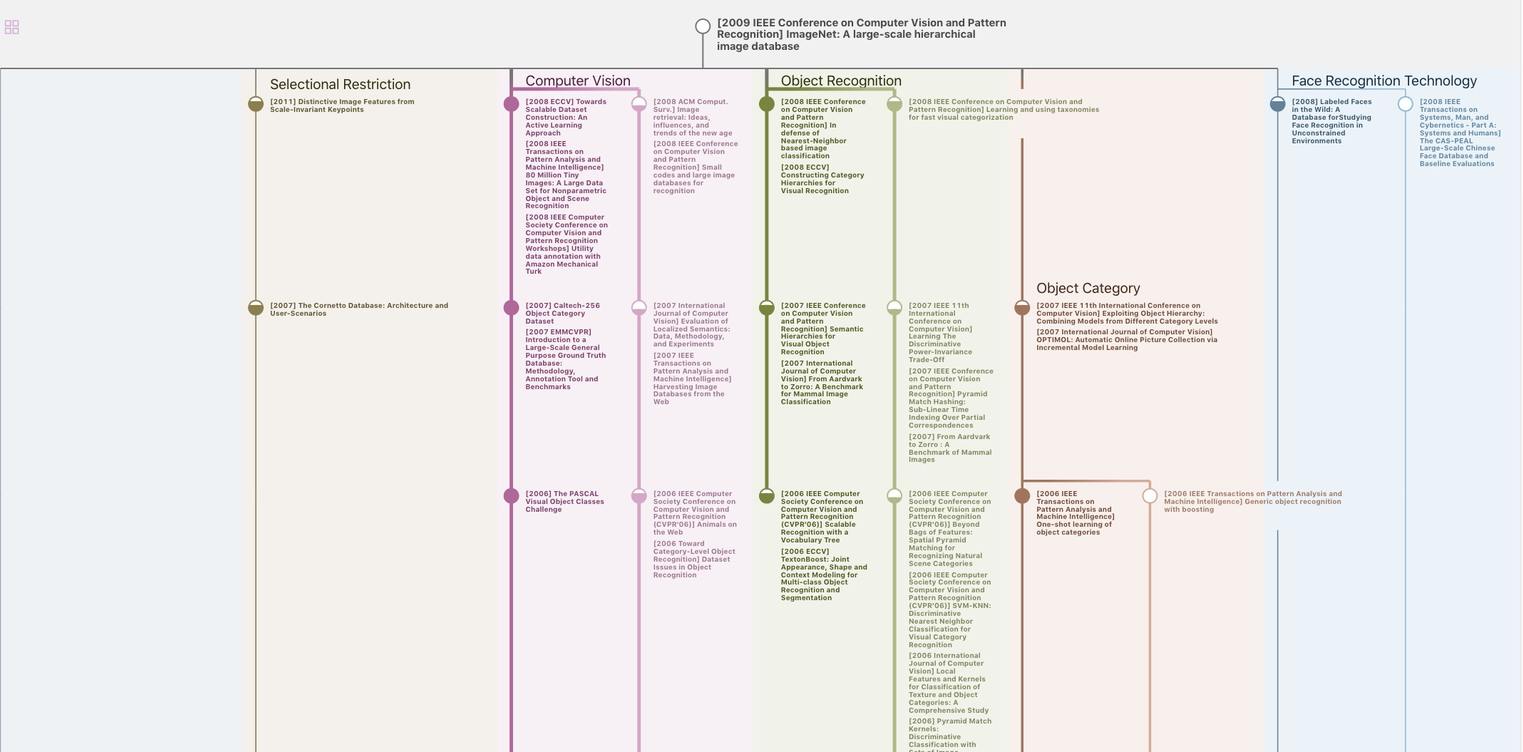
生成溯源树,研究论文发展脉络
Chat Paper
正在生成论文摘要