Lengthy Early Morning Instant Messages Reveal More than You Think: Analysing Interpersonal Relationships Using Mobile Communication Metadata
SSRN Electronic Journal(2022)
摘要
Privacy policies are one of the key factors that determine user's privacy exposure. Quite often such policies request users' consent to "only" collect their metadata. In this paper, we investigate the information that communication metadata can reveal, specifically, in regards to users' social circles. To this end, we developed an application and conducted a longitudinal field study with 25 participants, who installed our application on their personal smartphones. Over a period of four weeks, our application collected the metadata of the participants' communication with their social contacts over four channels, i.e., calls, SMS, e-mails, and Instant Messages (IMs). The content of the communication were not collected and the identities of the participants' social contacts were encrypted, so that only the users could get access to them. We leverage the collected metadata to examine whether and to what extend it is possible to exploit them in order to classify the participants' social contacts into four social categories, namely, family members, friends, acquaintances, and colleagues using Machine Learning (ML) techniques. By doing so, we do not only reproduce and replicate an existing study, but also extend it by further considering the metadata about IMs. In our user study, friends and family members call each other and exchange SMS more than acquaintances and colleagues. Moreover, as expected, IMs are exchanged more with friends followed by family, whereas e-mails more between acquaintances and colleagues. In addition, to validate the role of instant messaging channel, we show against our expectations that considering metadata about IMs only slightly improve the prediction of users' social ties. We also examine the most important features that lead to such predictions. We show that the prediction of social ties can be further enhanced by considering the aforementioned communication channels. Our study results in the f-measure score of 79.4% for a fine-grained classification of four considered social categories, achieving better results than related approaches, and 89.6% when considering the family category against all the others.
更多查看译文
关键词
Interpersonal relationships prediction,Privacy,Android,Machine learning,Metadata
AI 理解论文
溯源树
样例
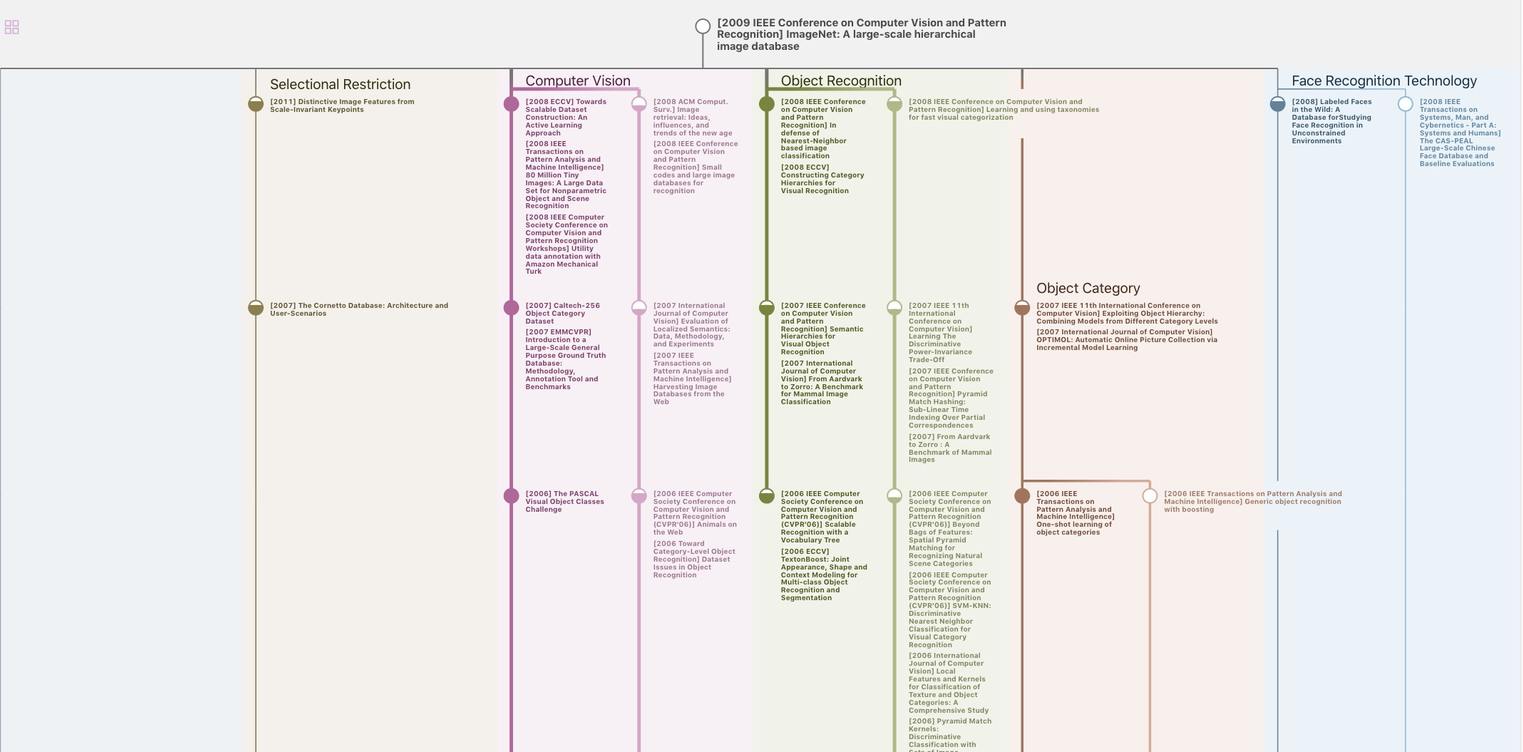
生成溯源树,研究论文发展脉络
Chat Paper
正在生成论文摘要