SGCNet: Scale-aware and global contextual network for crowd counting
Applied Intelligence(2022)
摘要
Recently, visual attention mechanisms have been employed in CNN-based crowd counting methods to overcome the interference of background noise and have achieved good performance. However, the existing methods usually focus on designing complex attention structures and extracting pixel-level contextual information, while ignoring global contextual information extraction at different scales. In this paper, to overcome scale variation and complex background noise, we propose a novel scale-aware and global contextual network (SGCNet) that employs multi-scale attention mechanisms to selectively strengthen features with different network scales. The key component of SGCNet is a multi-scale global contextual block that consists of multi-scale feature selection and global contextual information extraction, where global contextual information is adopted as guidance to weight features at different scales. Compared with the previous methods that ignore scale information injected into the attention mechanism, SGCNet achieves better counting performance via multi-scale contextual information extraction. Extensive experiments on four crowd counting datasets (ShanghaiTech, UCF_CC_50, UCF-QNRF, UCSD) demonstrate the effectiveness and superiority of the proposed method in highly congested noisy crowd scenes.
更多查看译文
关键词
Crowd counting,Global contextual information,Multi-scale contextual information,Scale variation,Background interference
AI 理解论文
溯源树
样例
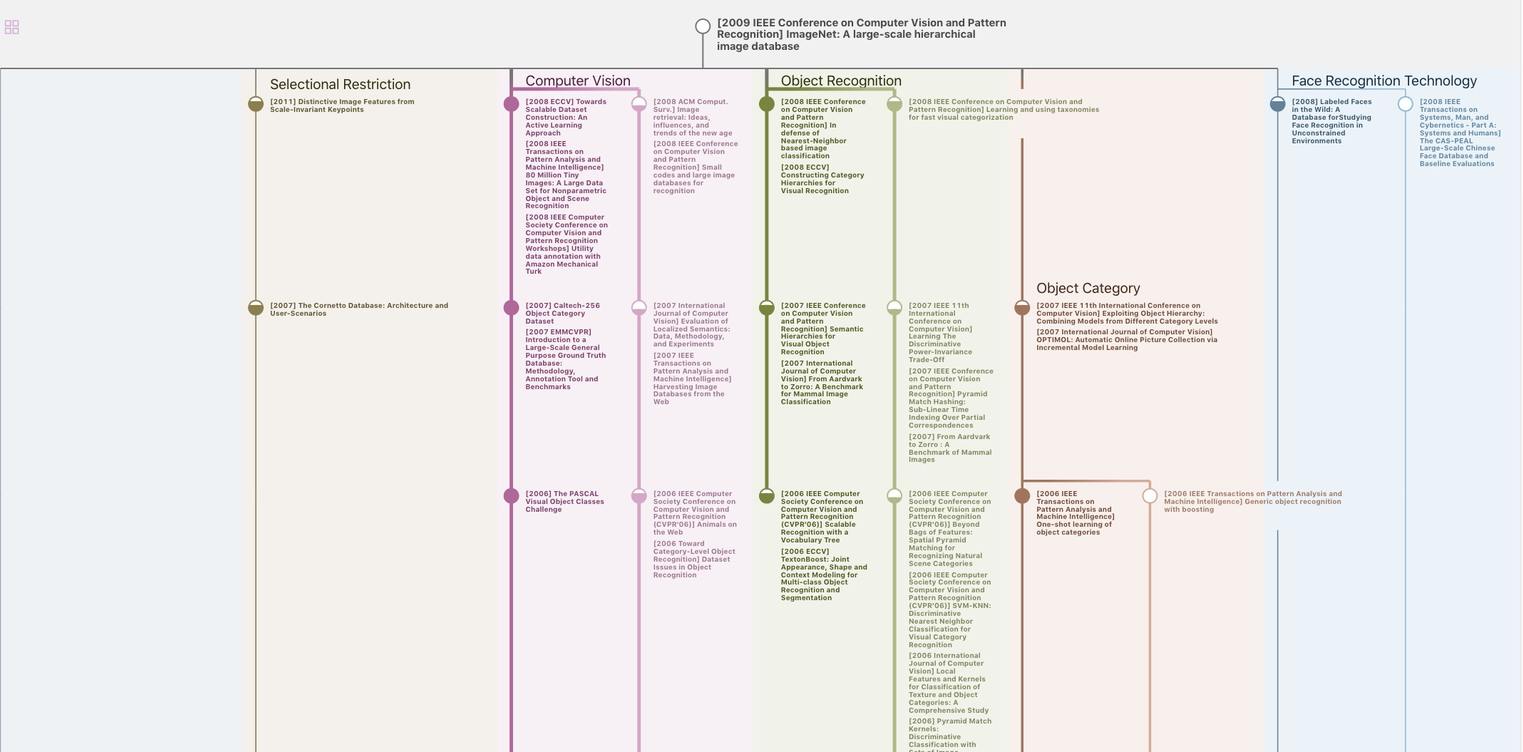
生成溯源树,研究论文发展脉络
Chat Paper
正在生成论文摘要