Machine Learning Empowered Well Placement in a Large Unconventional Tight Gas Field in China
Day 2 Tue, February 22, 2022(2022)
摘要
Abstract An undisclosed tight-gas field produces gas from a large heterogenous fluvial reservoir with limited well control in margins and appraisal areas, as well as sparse advanced logs (formation micro-imaging, magnetic resonance, or sonic tools, etc). The principal goal of this study is to provide a novel solution using machine-learning (ML) techniques to predict sandstone distribution and to some extent automate the process of optimizing well placement. This workflow overcomes low data quality, scaling, and inconsistency, and builds the bridge between geoscience and artificial intelligence (AI) software platforms. This newly proposed solution provides a combination of ML and geological studies to optimize well placement. The key steps of this technology are: Use unsupervised seismic waveform classification together with well logs to define sand body distribution and generate a reservoir risk map to support well planning. Construct a 3D grid with multiple variables to extract and integrate well logs and seismic attributes from existing 2D seismic lines for data organization and analysis. Set up the data transform mechanism to unlock restrictions of data analysis between subsurface domain and AI software platforms. Test multiple ML algorithms by carrying out a deep search to find seismic attributes that are the most sensitive to reservoir parameters and form the decision trees for quantitative analysis. Evaluate and select the high-performance results, export them into the subsurface software platform, and make the final property model available for integration and visualization to support well placement. Multiple tests have been completed to date in this gas field, and the results showed that the technique can improve well placement, add potential reserve and also improve the efficiency of the workflow by reducing the execution time by 240 times. The results provide solid support to achieve more reliable and accurate well placement. This new solution generates an improved reservoir risk map with flexibility of using many secondary variables or their combinations. It produces results with higher resolution and focuses on improving efficiency. It also reduces turnaround time and increases collaboration over conventional approaches by improving the accuracy of fast predictions as the data flow enriches the database rapidly, which unleashes big data potential. This innovative solution can be applied to other projects especially ML empowered well placements in complex geological settings.
更多查看译文
AI 理解论文
溯源树
样例
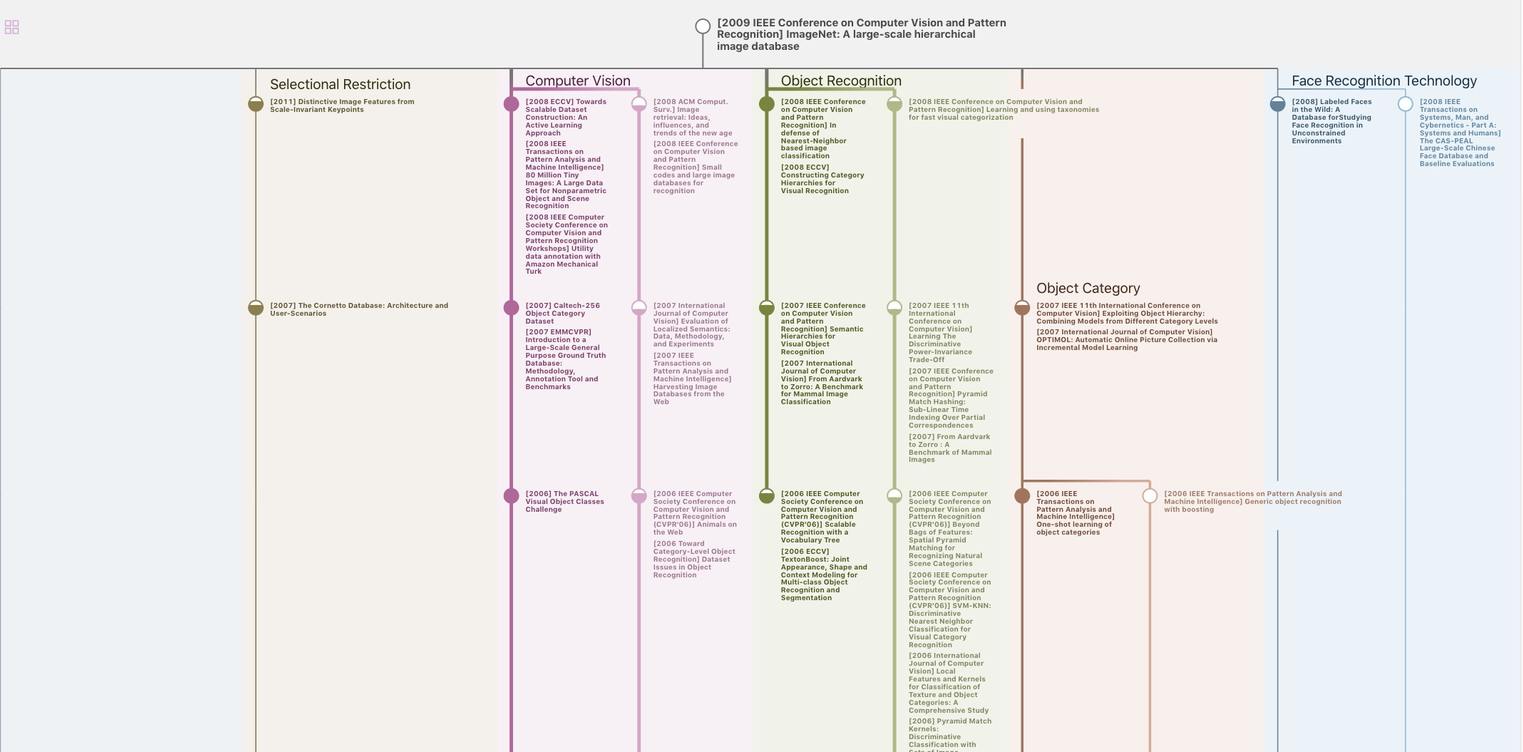
生成溯源树,研究论文发展脉络
Chat Paper
正在生成论文摘要