Semi-Supervised Ensemble Learning for Expanding the Low Sample Size of Microarray Dataset
2021 International Conference on Electrical, Computer and Energy Technologies (ICECET)(2021)
摘要
Cancer is considered one of the most common causes of death in the world. Using a microarray dataset for cancer classification can provide insight into possible treatment strategies. However, it is hard and expensive to collect a full labeled dataset. Self-training can solve this issue by train the classifier on limited labeled data, then adding unlabeled data incrementally for classification to select the most confidence between them and adding it to the original labeled data. The current study aims to propose a framework that can reduce the high dimensionality by using aggregation ranker-filters and particle swarm intelligence with the ensemble method (PSO-ensemble). Afterward, An Adaptive Self-Training Method (ASTM) can boost the labeled set by repeatedly incrementing it with the most confident samples from the unlabeled datasets to solve the low sample size issue. Empirical results demonstrate that ASTM can effectively improve classification performance.
更多查看译文
关键词
Semi-Supervised learning,Ensemble Learning,Feature Selection,cancer classification,Self-labeling
AI 理解论文
溯源树
样例
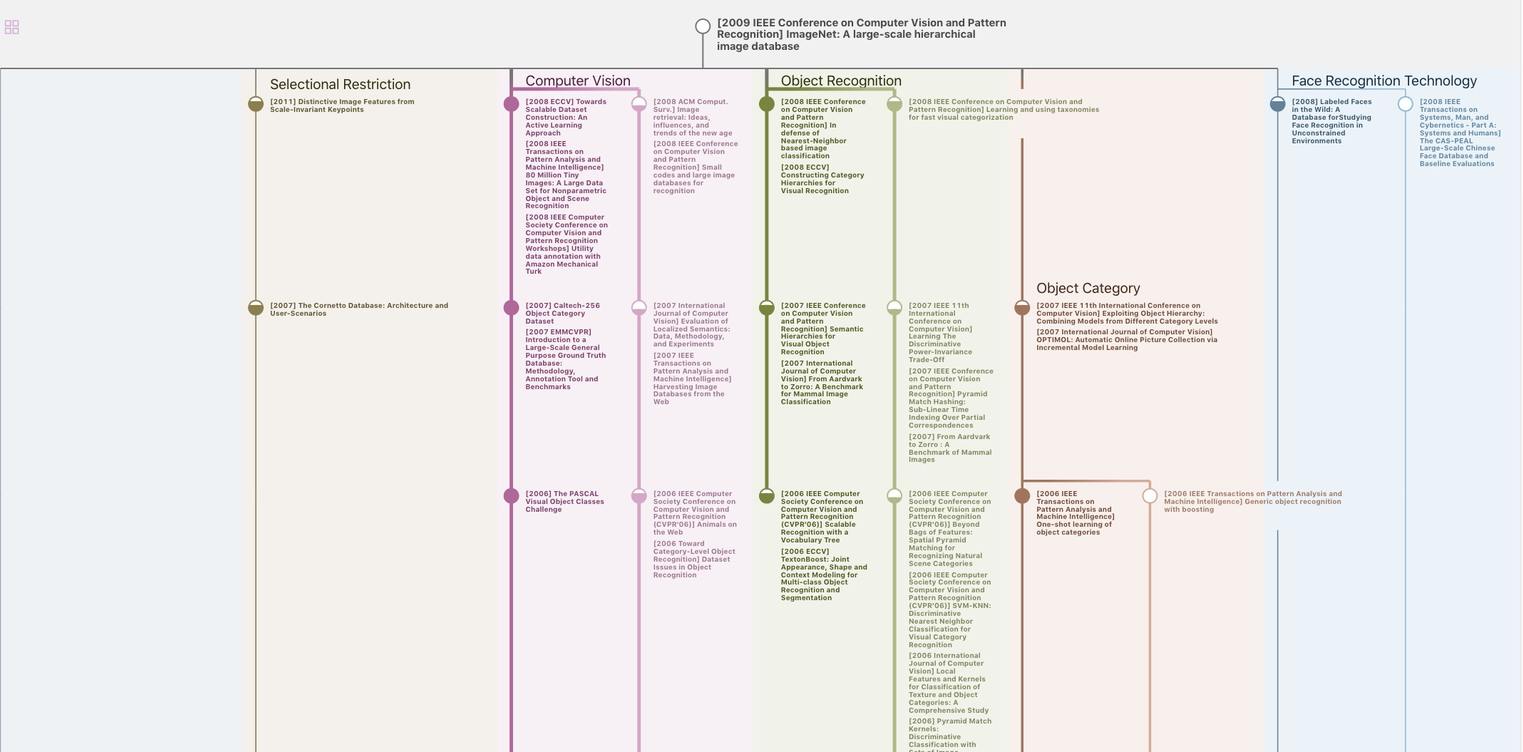
生成溯源树,研究论文发展脉络
Chat Paper
正在生成论文摘要