On reliability of reinforcement learning based production scheduling systems: a comparative survey
Journal of Intelligent Manufacturing(2022)
摘要
The deep reinforcement learning (DRL) community has published remarkable results on complex strategic planning problems, most famously in virtual scenarios for board and video games. However, the application to real-world scenarios such as production scheduling (PS) problems remains a challenge for current research. This is because real-world application fields typically show specific requirement profiles that are often not considered by state-of-the-art DRL research. This survey addresses questions raised in the domain of industrial engineering regarding the reliability of production schedules obtained through DRL-based scheduling approaches. We review definitions and evaluation measures of reliability both, in the classical numerical optimization domain with focus on PS problems and more broadly in the DRL domain. Furthermore, we define common ground and terminology and present a collection of quantifiable reliability definitions for use in this interdisciplinary domain. Concludingly, we identify promising directions of current DRL research as a basis for tackling different aspects of reliability in PS applications in the future.
更多查看译文
关键词
Reinforcement learning,Production scheduling,Reliability,Robustness,Machine learning
AI 理解论文
溯源树
样例
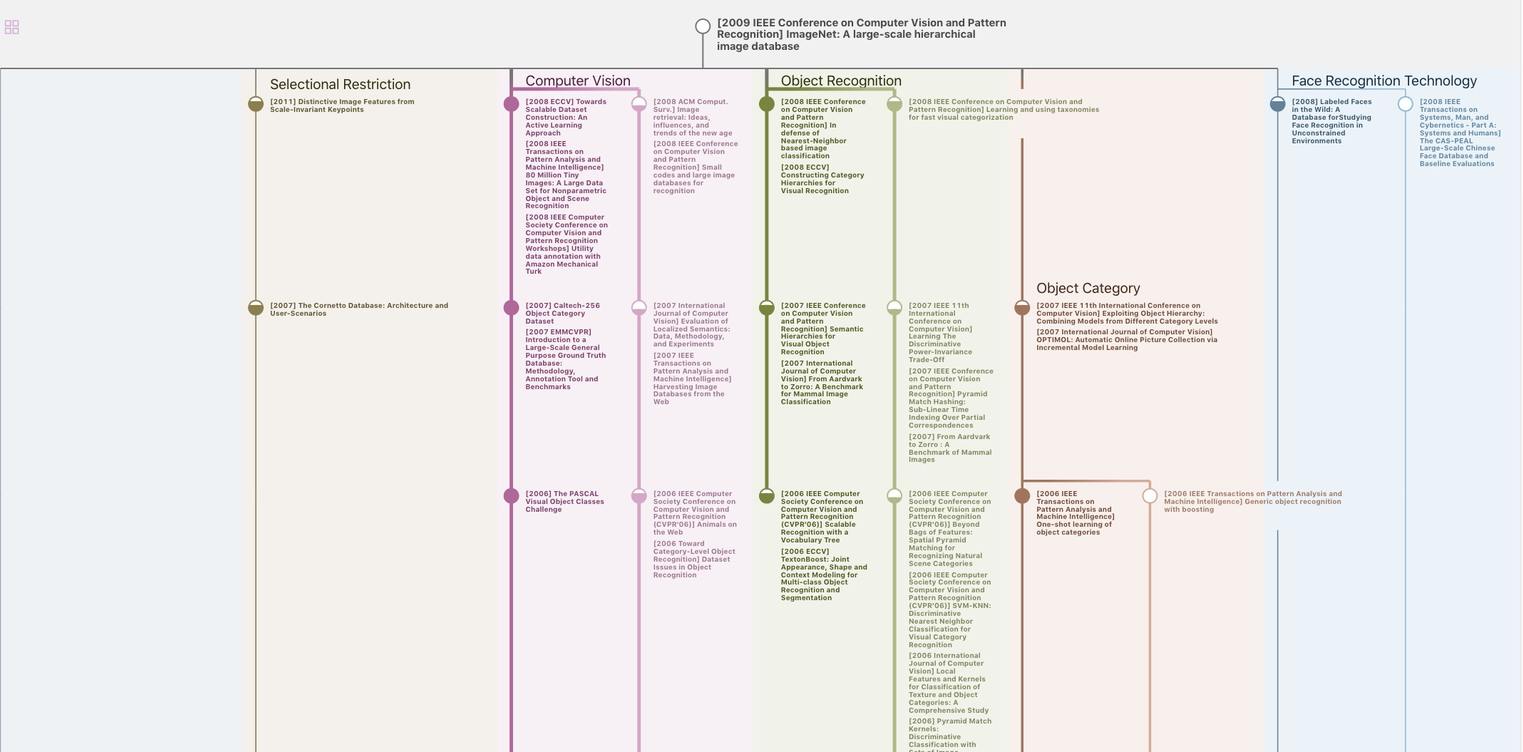
生成溯源树,研究论文发展脉络
Chat Paper
正在生成论文摘要