GANsformer: A Detection Network for Aerial Images with High Performance Combining Convolutional Network and Transformer.
Remote sensing(2022)
摘要
There has been substantial progress in small object detection in aerial images in recent years, due to the extensive applications and improved performances of convolutional neural networks (CNNs). Typically, traditional machine learning algorithms tend to prioritize inference speed over accuracy. Insufficient samples can cause problems for convolutional neural networks, such as instability, non-convergence, and overfitting. Additionally, detecting aerial images has inherent challenges, such as varying altitudes and illuminance situations, and blurred and dense objects, resulting in low detection accuracy. As a result, this paper adds a transformer backbone attention mechanism as a branch network, using the region-wide feature information. This paper also employs a generative model to expand the input aerial images ahead of the backbone. The respective advantages of the generative model and transformer network are incorporated. On the dataset presented in this study, the model achieves 96.77% precision, 98.83% recall, and 97.91% mAP by adding the Multi-GANs module to the one-stage detection network. These three indices are enhanced by 13.9%, 20.54%, and 10.27%, respectively, when compared to the other detection networks. Furthermore, this study provides an auto-pruning technique that may achieve 32.2 FPS inference speed with a minor performance loss while responding to the real-time detection task’s usage environment. This research also develops a macOS application for the proposed algorithm using Swift development technology.
更多查看译文
关键词
object detection,transformer,deep learning,aerial image,generative model,GANsformer detection network
AI 理解论文
溯源树
样例
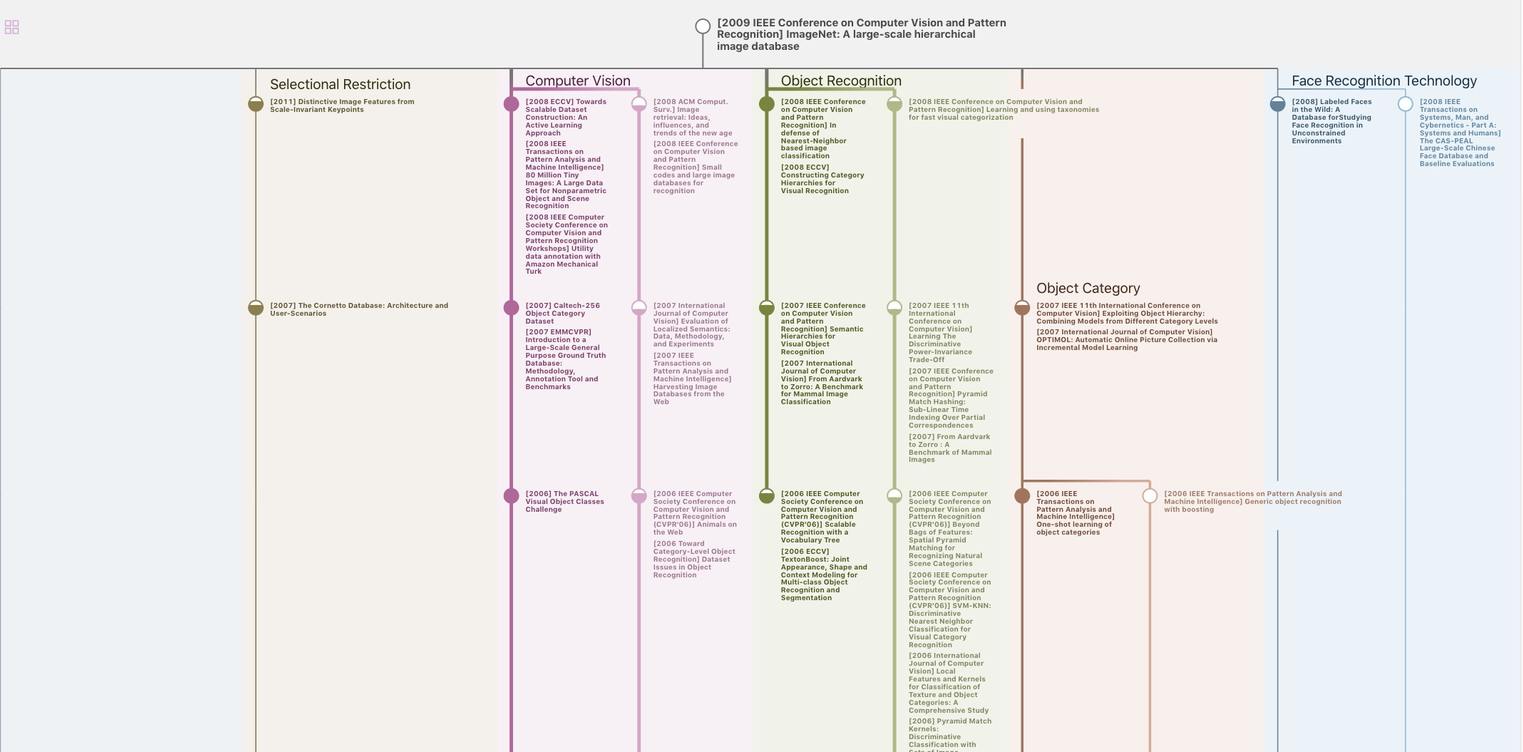
生成溯源树,研究论文发展脉络
Chat Paper
正在生成论文摘要