An Automated, Electronic Health Record-Based Algorithm to Classify Ischemic Stroke Etiology
STROKE(2022)
摘要
Introduction: Determining acute ischemic stroke (AIS) etiology is central to secondary stroke prevention, but can be diagnostically challenging. We built a stroke etiology classification model using electronic health record (EHR) data from non-cryptogenic AIS hospitalization encounters. Methods: We studied discharge summaries of non-cryptogenic AIS patients hospitalized at 2 academic, Comprehensive Stroke Centers from 2015-2020. The outcome was stroke etiology encoded in each institution’s stroke registry, abstracted from the discharge attending’s impression. We engineered variables including 1) clinical features from Unified Medical Language System concepts identified by a text mining tool, MetaMap , and 2) neuroimaging, echocardiographic, and laboratory features by regular expression operations. XGBoost classifier models were generated (80% training set, 20% testing set). A grid search of 36 model parameter value combinations and 10-fold cross validation were performed. We assessed variable importance in the optimized algorithm by calculating each feature’s Shapley value, the absolute value of the variable’s mean impact in predicting each specific stroke etiology. Results: The cohort was comprised of 1,993 patients (mean age 69 years, 55% male). We engineered a total of 2,004 features. Mean cross-validated accuracies of the best model in the training and validation sets were: 73.0% and 65.5%, respectively. Validation precision, recall, and F1 weighted scores were 66.2%, 65.5%, and 64.1% (mean cross-validated). The top 20 features contributing to etiology prediction are in Figure 1. Conclusion: We developed an EHR-based, automated tool to replicate a discharging attending’s stroke etiology diagnosis in non-cyptogenic patients. With further training in representative cohorts of non-cryptogenic and cryptogenic patients with an eventually diagnosed etiology, this algorithm may aide stroke etiology diagnosis before hospital discharge.
更多查看译文
AI 理解论文
溯源树
样例
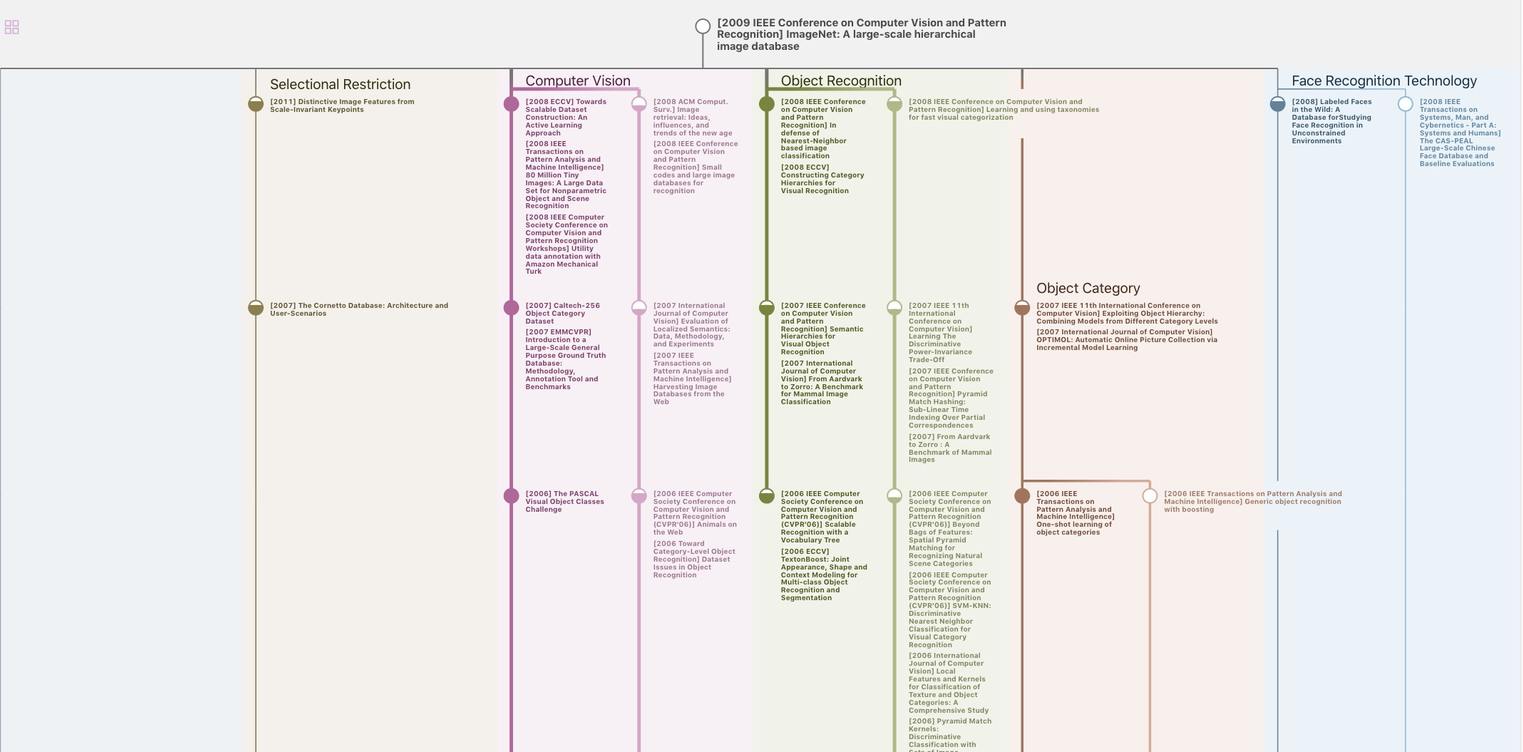
生成溯源树,研究论文发展脉络
Chat Paper
正在生成论文摘要