Low-Rank Softmax Can Have Unargmaxable Classes in Theory but Rarely in Practice
PROCEEDINGS OF THE 60TH ANNUAL MEETING OF THE ASSOCIATION FOR COMPUTATIONAL LINGUISTICS (ACL 2022), VOL 1: (LONG PAPERS)(2022)
摘要
Classifiers in natural language processing (NLP) often have a large number of output classes. For example, neural language models (LMs) and machine translation (MT) models both predict tokens from a vocabulary of thousands. The Softmax output layer of these models typically receives as input a dense feature representation, which has much lower dimensionality than the output. In theory, the result is some words may be impossible to be predicted via argmax, irrespective of input features, and empirically, there is evidence this happens in small language models (Demeter et al., 2020). In this paper we ask whether it can happen in practical large language models and translation models. To do so, we develop algorithms to detect such unargmaxable tokens in public models. We find that 13 out of 150 models do indeed have such tokens; however, they are very infrequent and unlikely to impact model quality. We release our algorithms and code so that others can test their models.(1)
更多查看译文
关键词
unargmaxable classes,low-rank
AI 理解论文
溯源树
样例
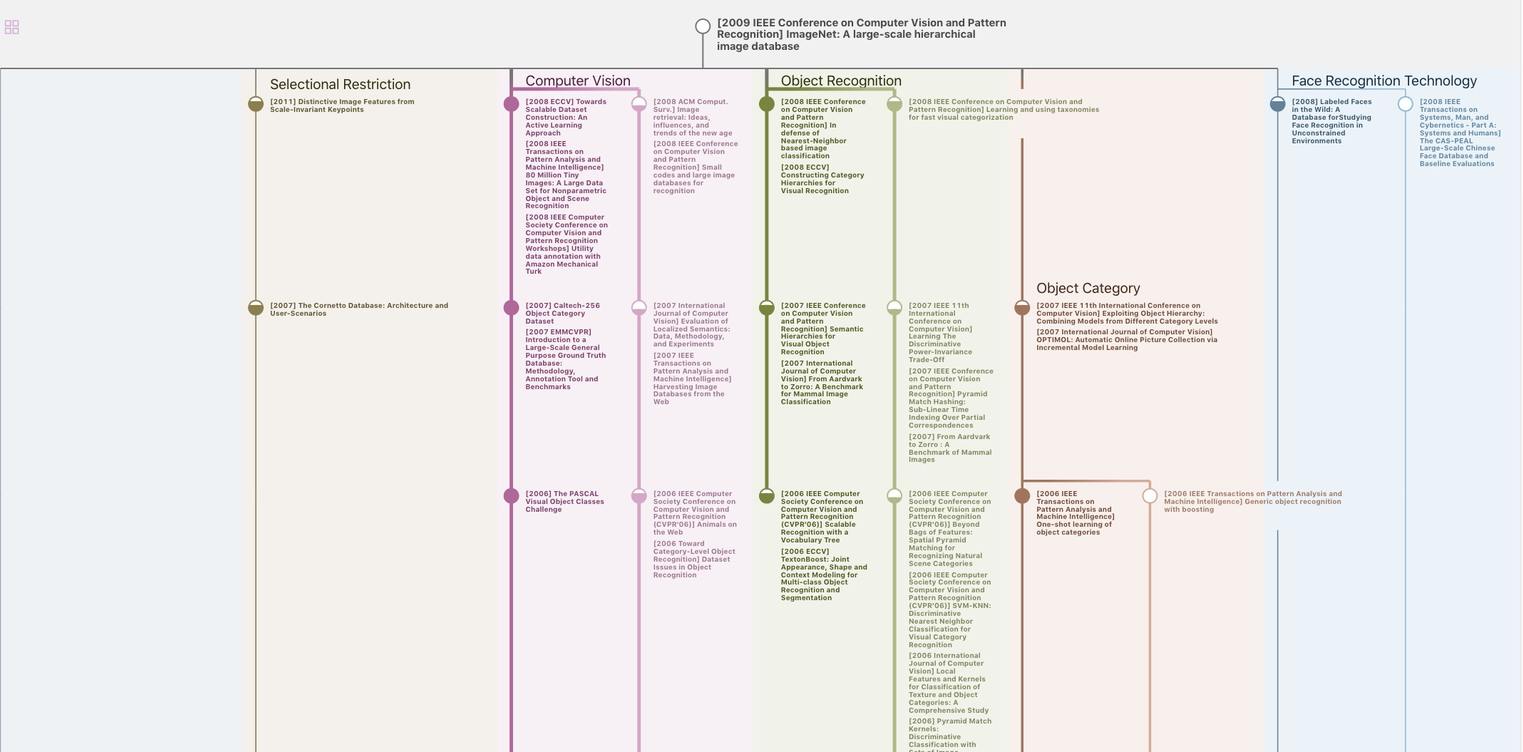
生成溯源树,研究论文发展脉络
Chat Paper
正在生成论文摘要