PNM: Pixel Null Model for General Image Segmentation
arxiv(2022)
摘要
A major challenge in image segmentation is classifying object boundaries. Recent efforts propose to refine the segmentation result with boundary masks. However, models are still prone to misclassifying boundary pixels even when they correctly capture the object contours. In such cases, even a perfect boundary map is unhelpful for segmentation refinement. In this paper, we argue that assigning proper prior weights to error-prone pixels such as object boundaries can significantly improve the segmentation quality. Specifically, we present the \textit{pixel null model} (PNM), a prior model that weights each pixel according to its probability of being correctly classified by a random segmenter. Empirical analysis shows that PNM captures the misclassification distribution of different state-of-the-art (SOTA) segmenters. Extensive experiments on semantic, instance, and panoptic segmentation tasks over three datasets (Cityscapes, ADE20K, MS COCO) confirm that PNM consistently improves the segmentation quality of most SOTA methods (including the vision transformers) and outperforms boundary-based methods by a large margin. We also observe that the widely-used mean IoU (mIoU) metric is insensitive to boundaries of different sharpness. As a byproduct, we propose a new metric, \textit{PNM IoU}, which perceives the boundary sharpness and better reflects the model segmentation performance in error-prone regions.
更多查看译文
关键词
pixel null model,segmentation,image
AI 理解论文
溯源树
样例
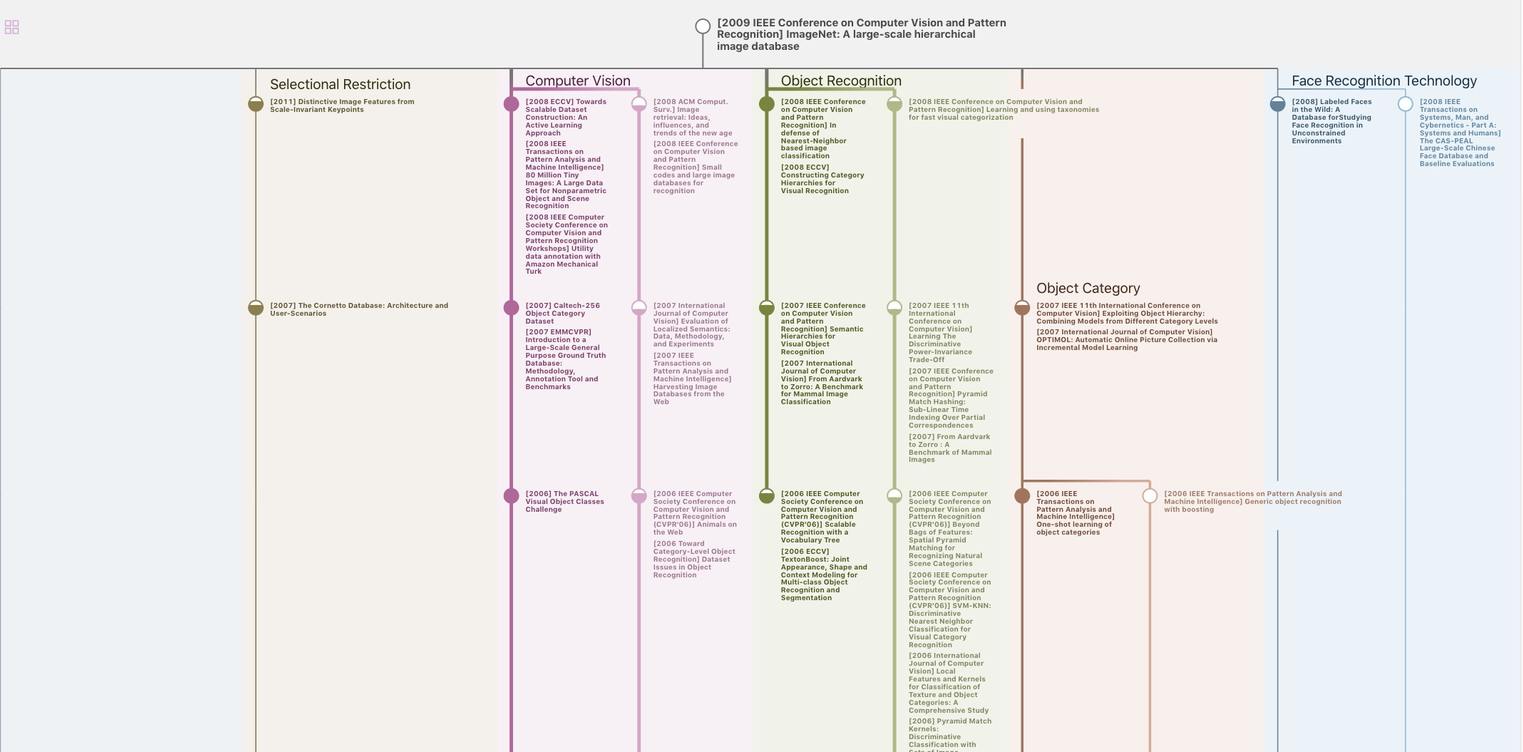
生成溯源树,研究论文发展脉络
Chat Paper
正在生成论文摘要