Learning from Attacks: Attacking Variational Autoencoder for Improving Image Classification
arxiv(2022)
摘要
Adversarial attacks are often considered as threats to the robustness of Deep Neural Networks (DNNs). Various defending techniques have been developed to mitigate the potential negative impact of adversarial attacks against task predictions. This work analyzes adversarial attacks from a different perspective. Namely, adversarial examples contain implicit information that is useful to the predictions i.e., image classification, and treat the adversarial attacks against DNNs for data self-expression as extracted abstract representations that are capable of facilitating specific learning tasks. We propose an algorithmic framework that leverages the advantages of the DNNs for data self-expression and task-specific predictions, to improve image classification. The framework jointly learns a DNN for attacking Variational Autoencoder (VAE) networks and a DNN for classification, coined as Attacking VAE for Improve Classification (AVIC). The experiment results show that AVIC can achieve higher accuracy on standard datasets compared to the training with clean examples and the traditional adversarial training.
更多查看译文
AI 理解论文
溯源树
样例
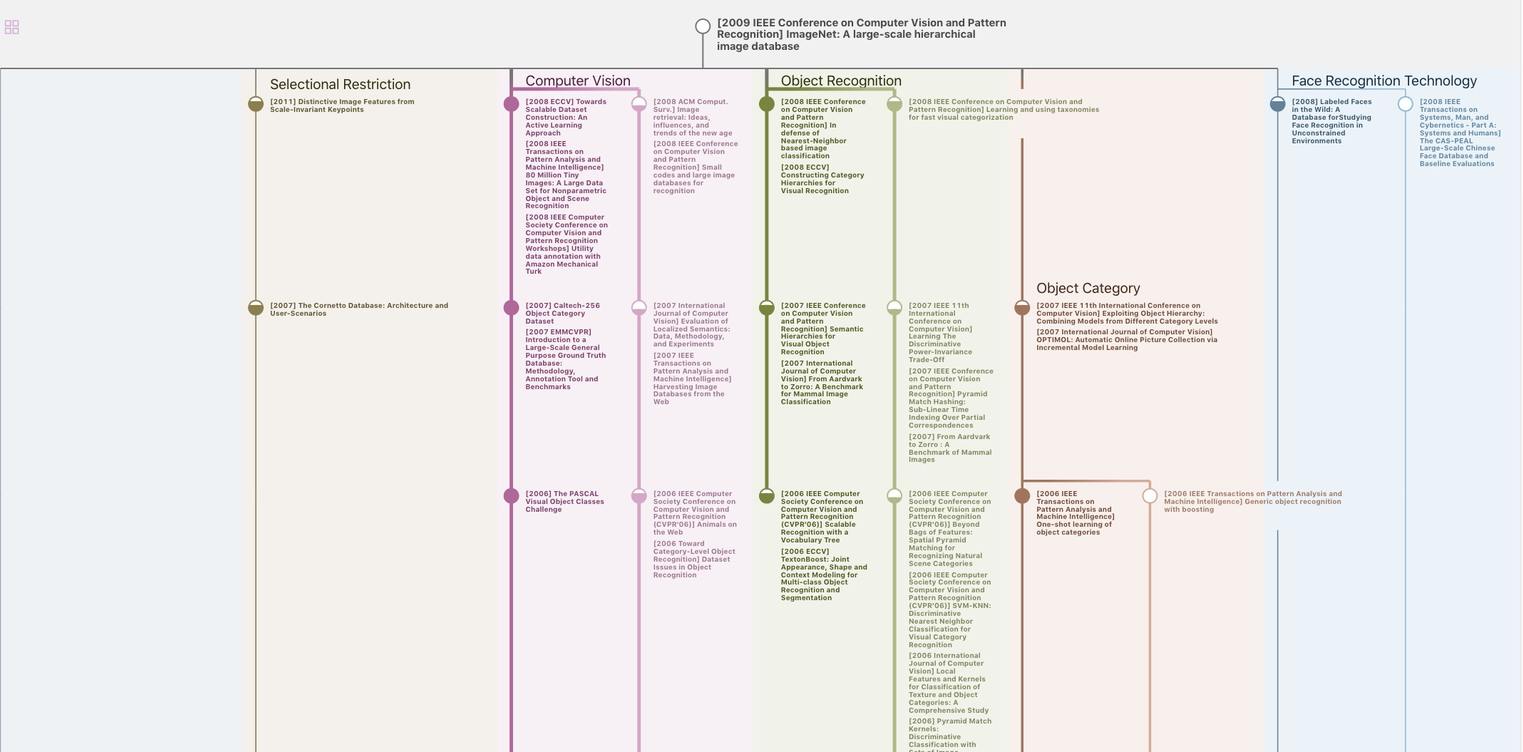
生成溯源树,研究论文发展脉络
Chat Paper
正在生成论文摘要