Detection and characterization of microseismic events from fiber-optic DAS data using deep learning
arxiv(2022)
摘要
Microseismic analysis is a valuable tool for fracture characterization in the earth's subsurface. As distributed acoustic sensing (DAS) fibers are deployed at depth inside wells, they hold vast potential for high-resolution microseismic analysis. However, the accurate detection of microseismic signals in continuous DAS data is challenging and time-consuming. We design, train, and deploy a deep learning model to detect microseismic events in DAS data automatically. We create a curated dataset of nearly 7,000 manually-selected events and an equal number of background noise examples. We optimize the deep learning model's network architecture together with its training hyperparameters by Bayesian optimization. The trained model achieves an accuracy of 98.6% on our benchmark dataset and even detects low-amplitude events missed during manual labeling. Our methodology detects more than 100,000 events allowing the reconstruction of spatio-temporal fracture development far more accurately and efficiently than would have been feasible by traditional methods.
更多查看译文
关键词
microseismic events,deep learning,das data,fiber-optic
AI 理解论文
溯源树
样例
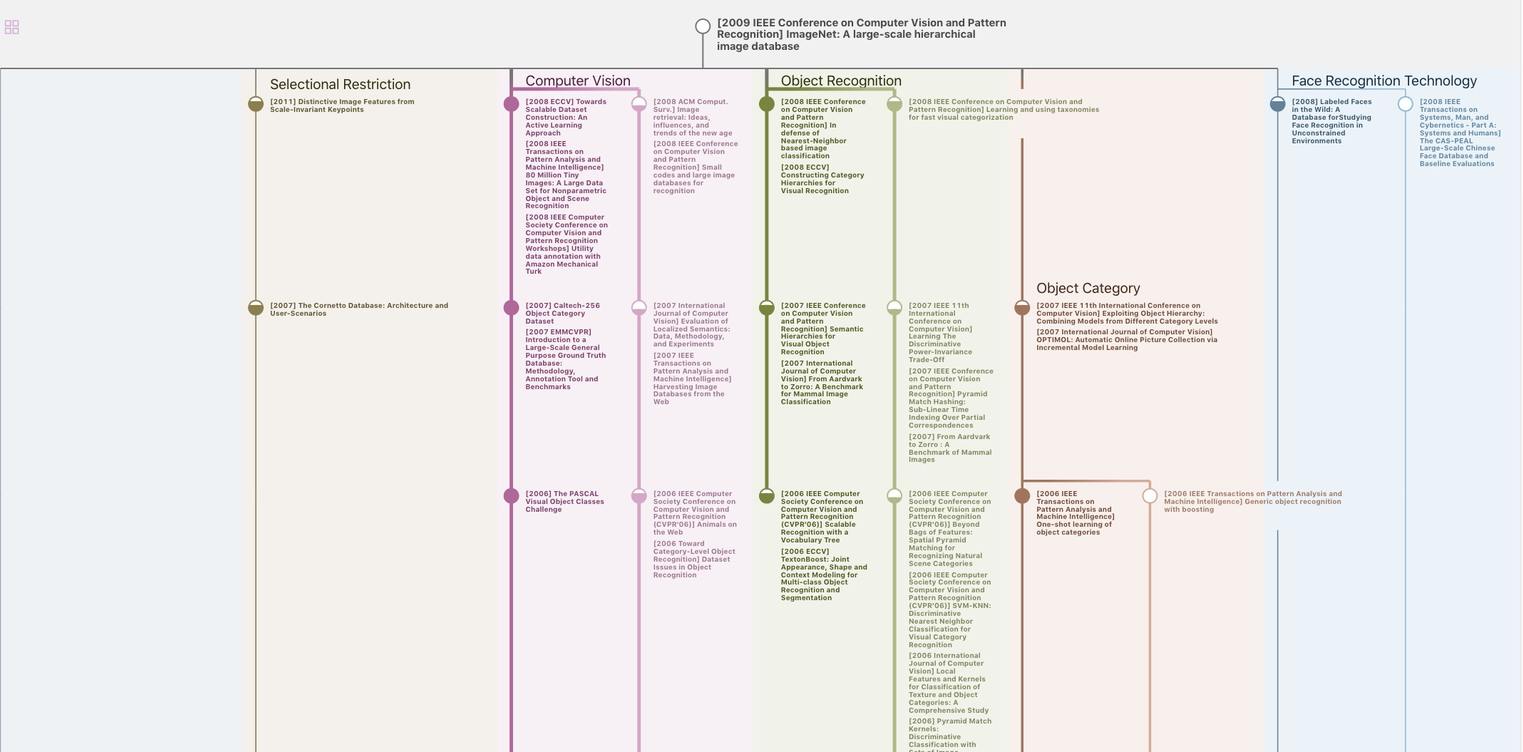
生成溯源树,研究论文发展脉络
Chat Paper
正在生成论文摘要