Diversifying Content Generation for Commonsense Reasoning with Mixture of Knowledge Graph Experts
FINDINGS OF THE ASSOCIATION FOR COMPUTATIONAL LINGUISTICS (ACL 2022)(2022)
摘要
Generative commonsense reasoning (GCR) in natural language is to reason about the commonsense while generating coherent text. Recent years have seen a surge of interest in improving the generation quality of commonsense reasoning tasks. Nevertheless, these approaches have seldom investigated diversity in the GCR tasks, which aims to generate alternative explanations for a real-world situation or predict all possible outcomes. Diversifying GCR is challenging as it expects to generate multiple outputs that are not only semantically different but also grounded in commonsense knowledge. In this paper, we propose MoKGE, a novel method that diversifies the generative reasoning by a mixture of expert (MoE) strategy on commonsense knowledge graphs (KG). A set of knowledge experts seek diverse reasoning on KG to encourage various generation outputs. Empirical experiments demonstrated that MoKGE can significantly improve the diversity while achieving on par performance on accuracy on two GCR benchmarks, based on both automatic and human evaluations.
更多查看译文
关键词
commonsense reasoning,knowledge graph experts,content generation
AI 理解论文
溯源树
样例
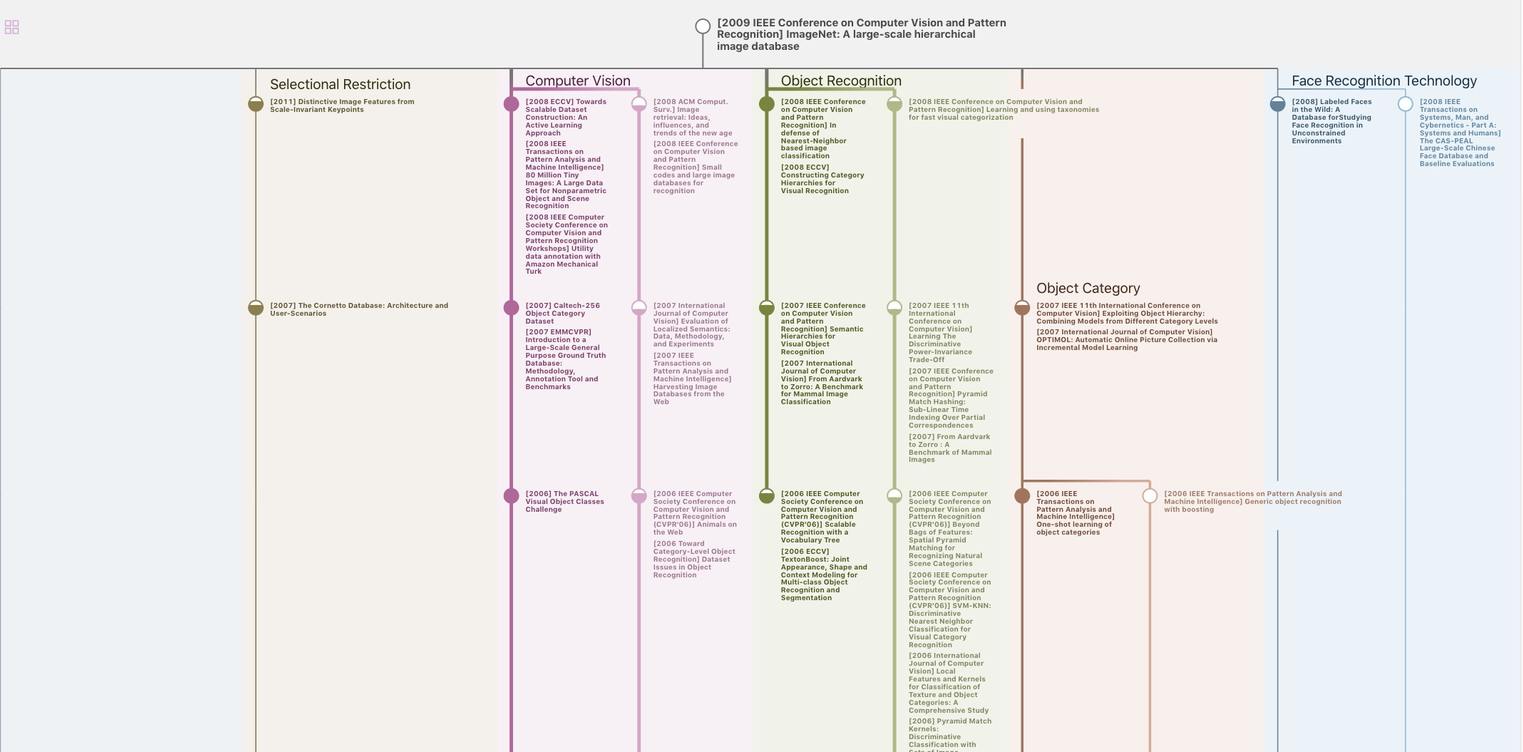
生成溯源树,研究论文发展脉络
Chat Paper
正在生成论文摘要