An Improved Infrared and Visible Image Fusion Using an Adaptive Contrast Enhancement Method and Deep Learning Network with Transfer Learning
Remote sensing(2022)
摘要
Deep learning (DL) has achieved significant attention in the field of infrared (IR) and visible (VI) image fusion, and several attempts have been made to enhance the quality of the final fused image. It produces better results than conventional methods; however, the captured image cannot acquire useful information due to environments with poor lighting, fog, dense smoke, haze, and the noise generated by sensors. This paper proposes an adaptive fuzzy-based preprocessing enhancement method that automatically enhances the contrast of images with adaptive parameter calculation. The enhanced images are then decomposed into base and detail layers by anisotropic diffusion-based edge-preserving filters that remove noise while smoothing the edges. The detailed parts are fed into four convolutional layers of the VGG-19 network through transfer learning to extract features maps. These features maps are fused by multiple fusion strategies to obtain the final fused detailed layer. The base parts are fused by the PCA method to preserve the energy information. Experimental results reveal that our proposed method achieves state-of-the-art performance compared with existing fusion methods in a subjective evaluation through the visual experience of experts and statistical tests. Moreover, the objective assessment parameters are conducted by various parameters (FMI, SSIMa, API, EN, QFAB, and NFAB) which were used in the comparison method. The proposed method achieves 0.2651 to 0.3951, 0.5827 to 0.8469, 56.3710 to 71.9081, 4.0117 to 7.9907, and 0.6538 to 0.8727 gain for FMI, SSIMa, API, EN, and QFAB, respectively. At the same time, the proposed method has more noise reduction (0.3049 to 0.0021) that further justifies the efficacy of the proposed method than conventional methods.
更多查看译文
关键词
deep learning,image fusion,computer vision,pattern recognition,machine learning
AI 理解论文
溯源树
样例
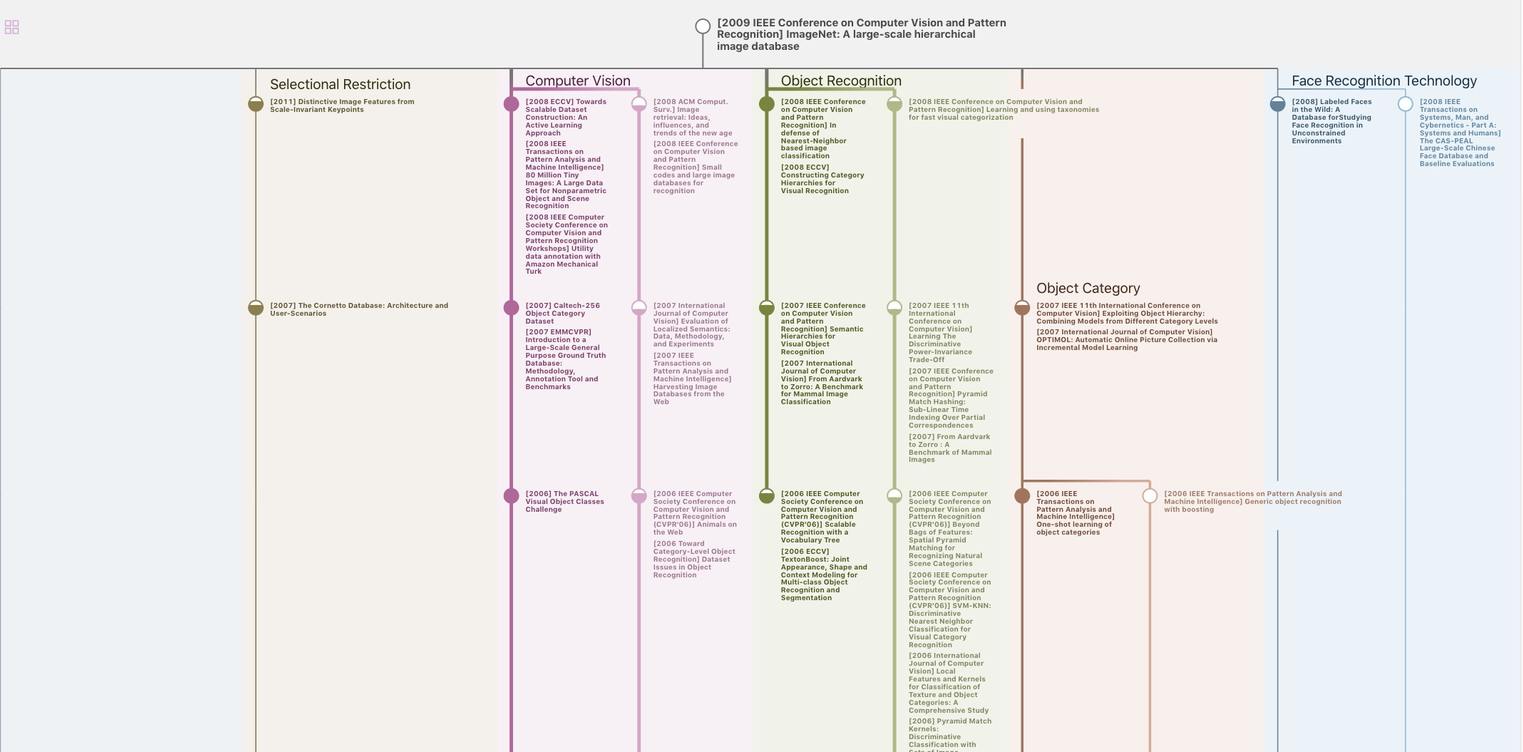
生成溯源树,研究论文发展脉络
Chat Paper
正在生成论文摘要