A Data Driven Method to Predict and Restore Missing Well Head Flow Pressure
Day 3 Wed, February 23, 2022(2022)
摘要
Abstract With the rapid development of oilfield exploration and development technology and the continuous improvement of automation and informationization, the petroleum industry has entered the era of digitalization and intelligence. Surveillance data including pressure and saturation play increasingly role of reservoir development machine learning modelling. A large amount of high-quality data can enhance the accuracy and robustness of the neural network model. However, during actual reservoir development, not all data are easily available. Data acquisition is limited to high-cost tests techniques, pressure bulid-up test, reservoir saturation test, and production logging test etc. In this study, we develop a novel workflow to forecast and restore missing well head flow pressure (WHFP) by utilizing Random Forest (RF) algorithms based on the actual dataset obtained from the middle east carbonate reservoir. To evaluate the effect of prediction pressure results, we employed predicted WHFP into production prediction neural network model. With the support of predicted WHFP, the quality and accuracy of neural network production prediction model is much improved. This study provides a low-cost and high-precision WHFP prediction and restoration method for reservoir engineers in decision making.
更多查看译文
AI 理解论文
溯源树
样例
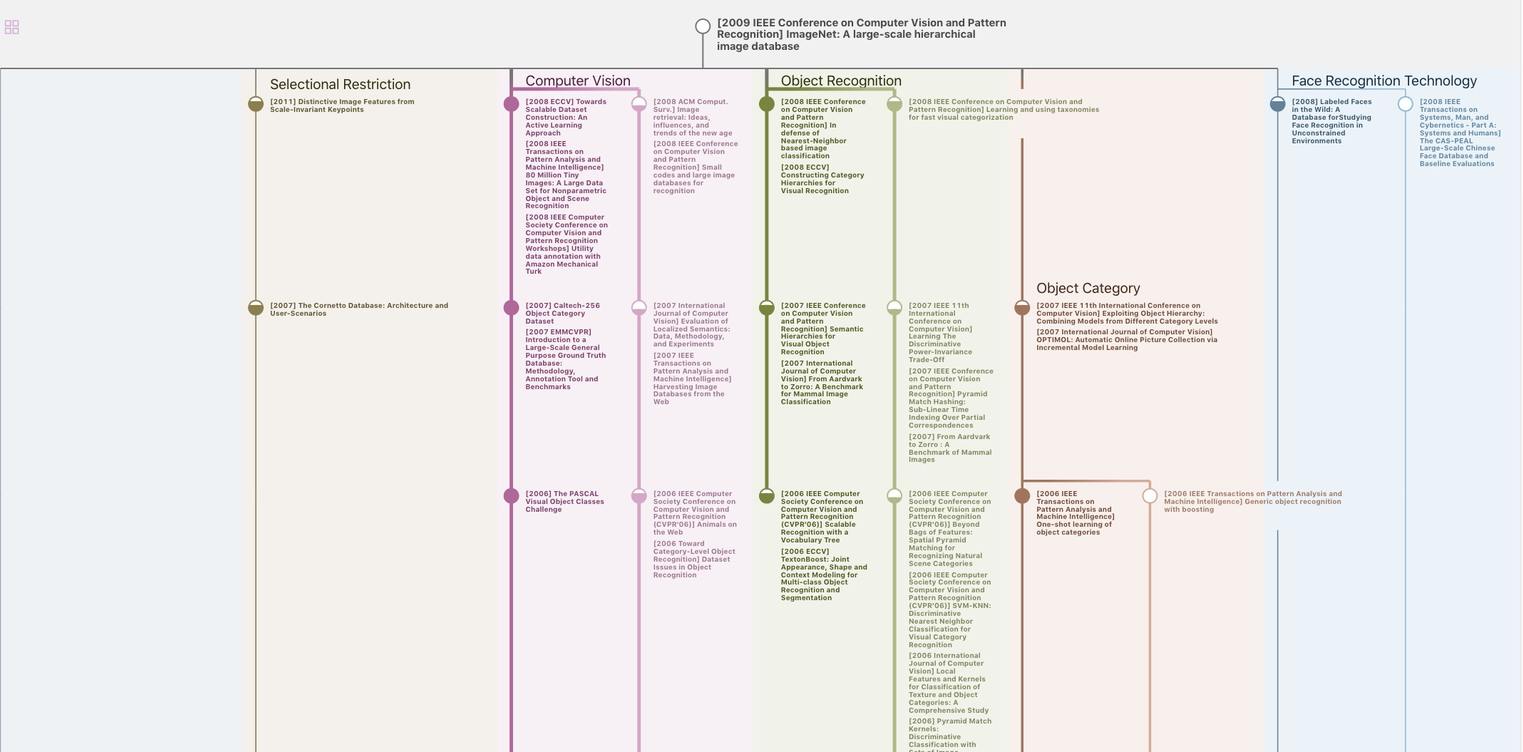
生成溯源树,研究论文发展脉络
Chat Paper
正在生成论文摘要