Machine Learning Assisted Synthesis of Lithium-Ion Batteries Cathode Materials
SSRN Electronic Journal(2021)
摘要
Optimizing synthesis parameters is crucial in fabricating an ideal cathode material; however, the design space is too vast to be fully explored using an Edisonian approach. Here, by clustering eleven domain-expert-derived-descriptors from literature, we use an inverse design surrogate model to build up the experimental parameters-property relationship. Without struggling with the trial-and-error method, the model enables design variables prediction that serves as an effective strategy for cathode retrosynthesis. More importantly, not only did we overcome the data scarcity problem, but the machine learning model has guided us to achieve cathode with high discharge capacity and Coulombic efficiency of 209.5 mAh/g and 86%, respectively. This work demonstrates an inverse design-to-device pipeline with unprecedented potential to accelerate the discovery of high-energy-density cathodes.
更多查看译文
关键词
Lithium-ion batteries,NCM cathode,Inverse design,Machine learning,Design-to-device pipeline
AI 理解论文
溯源树
样例
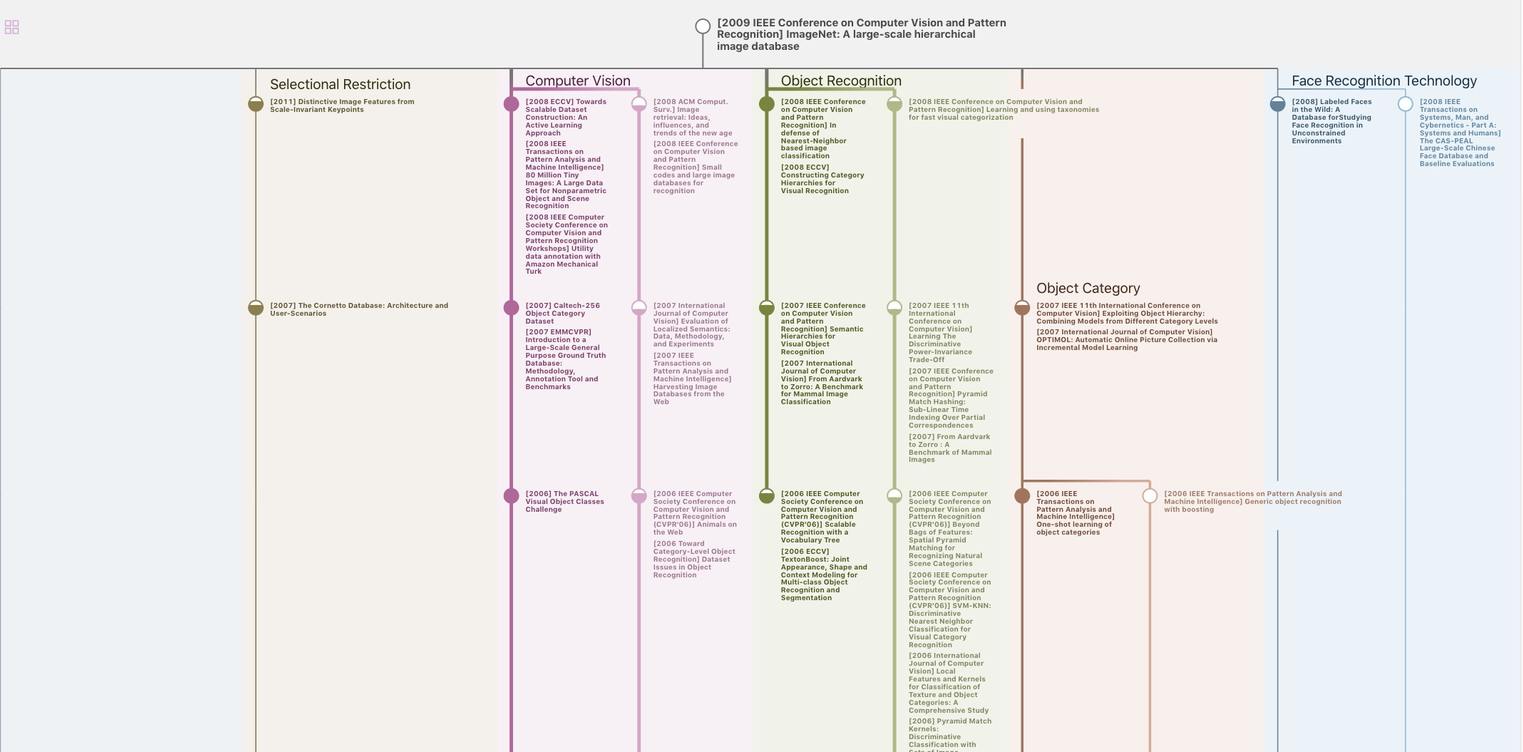
生成溯源树,研究论文发展脉络
Chat Paper
正在生成论文摘要