Hybrid descriptor and optimized CNN with transfer learning for face recognition
Multimedia Tools and Applications(2022)
摘要
This work focuses on the development of optimized algorithms applied in the field of Face Recognition (FR). The strategy adopted represents the contribution of hybrid face descriptors followed by the selection of optimal characteristics for significantly improving systems performance. The hybrid descriptors use the combination of several pieces of information and their optimization. Indeed, these are two hybrid structures developed and implemented. The first supports the combination of several classic descriptors Gabor filter with Histogram Oriented Gradient (HOG), Local Phase Quantization (LPQ) or Principal Component Analysis (PCA) for the facial features extraction. The second structure relies on Deep Learning (Transfer Learning (TL)) by relying on the Convolutional Neural Networks ( CNN) named AlexNet without its last layers and this in order to extract the most relevant face characteristics. The Particle Swarm Optimization (PSO) algorithm optimizes these characteristics extracted from these two algorithms. The first structure is followed by a data reduction step based on Linear Discriminant Analysis (LDA). The classification is carried out by the cosine distance measurement with the data normalization. A two-part data division algorithm, one part for training and one part for testing, will follow the second structure and a “Softmax” single-layer classifier is added to its output. The experimentation is conducted on existing dataset: Labeled Faces in the Wild (LFW), as well as on databases (ORL, AR and Thermal Tufts Face) and good performance is obtained.
更多查看译文
关键词
Descriptor,Hybrid,Optimization,Deep learning,Transfer learning,Thermal face
AI 理解论文
溯源树
样例
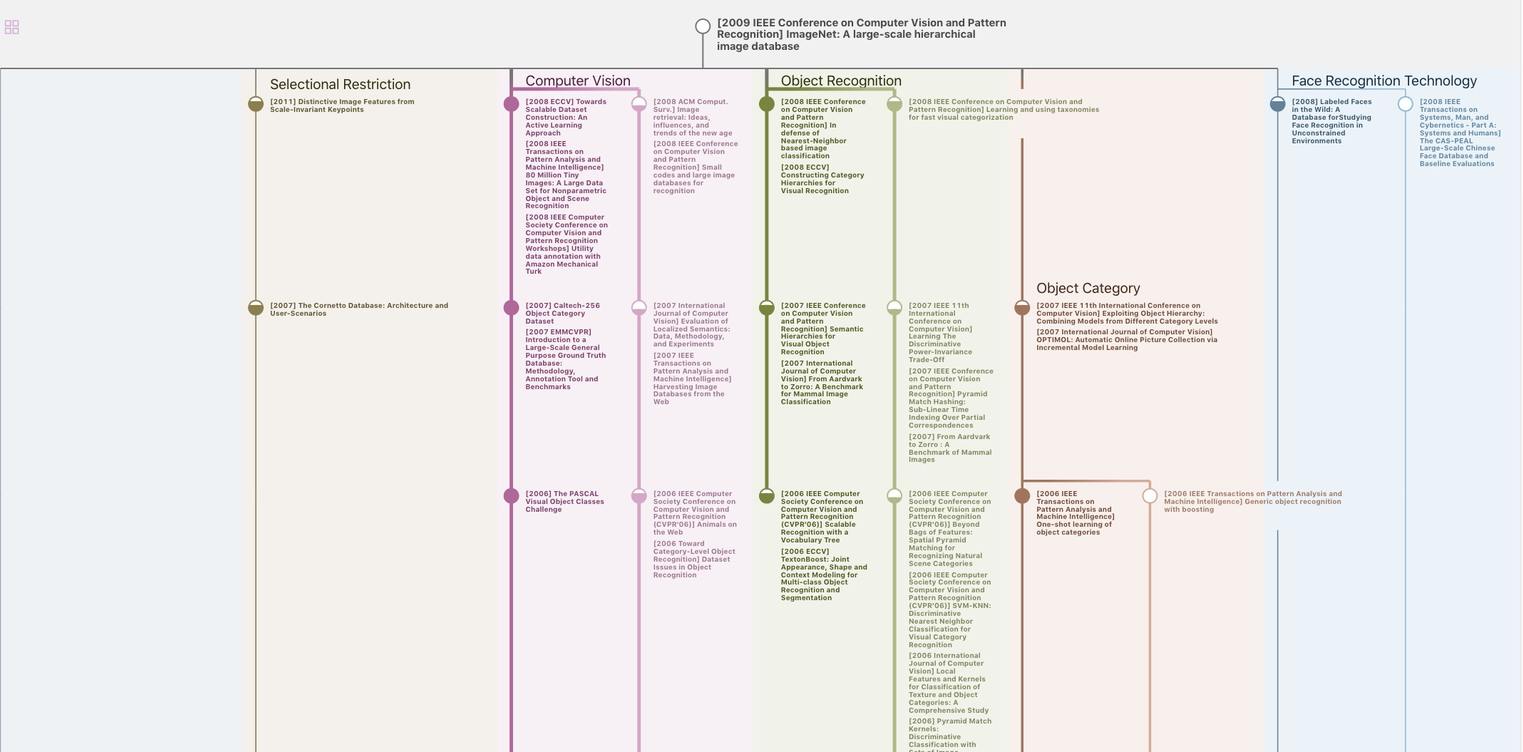
生成溯源树,研究论文发展脉络
Chat Paper
正在生成论文摘要