Underground Object Classification Using Deep 3-D Convolutional Networks and Multiple Mirror Encoding for GPR Data
IEEE geoscience and remote sensing letters(2022)
摘要
Ground-penetrating radar (GPR) is an effective tool for underground object detection, but its data interpretation remains a great challenge. In this letter, we propose a novel underground object classification algorithm using deep 3-D convolutional networks (C3D) and multiple mirror encoding (MME) for 3-D GPR data. Although deep learning technique has been applied to interpret the GPR data, most of the existing methods are based on GPR B-scans and have a relatively low accuracy since the reflections from various subsurface targets present similar hyperbolic patterns in B-scans. To improve the classification accuracy, we use 3-D GPR data as training data for C3D to capture the spatio-temporal features between parallel B-scans. Since 3-D GPR data including single object has different sizes in consideration of actual sizes of objects, they are rearranged by the MME method to enhance spatio-temporal features, as well as to satisfy the requirement of the network input. Experimental results demonstrate that the proposed method outperforms the state-of-the-art B-scan-based methods.
更多查看译文
关键词
Three-dimensional displays,Feature extraction,Buried object detection,Plastic products,Mirrors,Convolution,Reflection,3-D convolutional networks (C3D),3-D data,ground-penetrating radar (GPR),multiple mirror encoding (MME),underground object classification
AI 理解论文
溯源树
样例
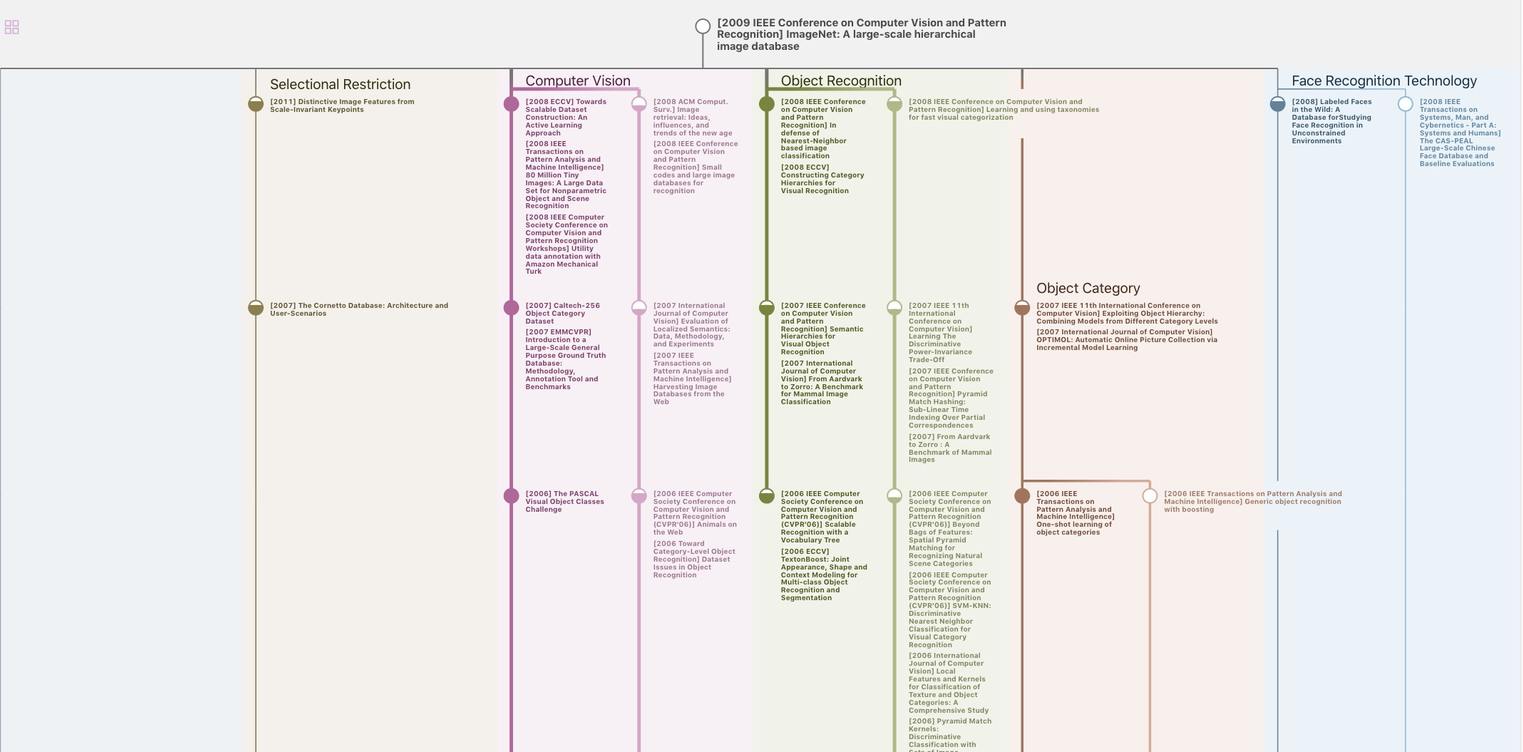
生成溯源树,研究论文发展脉络
Chat Paper
正在生成论文摘要