Automatic and accurate segmentation of peripherally inserted central catheter (PICC) from chest X-rays using multi-stage attention-guided learning
Neurocomputing(2022)
摘要
Segmentation of Peripherally Inserted Central Catheter (PICC) from chest X-rays (CXR) is the first step towards automatic PICC position confirmation. PICC is a relatively small and thin tube that occupies only a small proportion on the entire CXR, while the resolution of CXR is generally quite high. To address such large-scene-thin-object segmentation problem, in this paper we propose an automatic multi-stage attention-guided framework (MAG-Net) for PICC segmentation and tip positioning, which contains a coarse stage for rough locating and a fine stage for precise segmenting. A coarse network with pyramid pooling for larger receptive field is presented to generate an initial segmentation mask. After that, for small object refinement, an adaptive spatial weighting encoder is introduced to achieve a differentiable hard attention, and a position self-attention module is utilized to enhance the relationship between spatial pixels and maintain the integrity of target. Also, a pixelshuffling decoder is designed to restore the resolution without inserting irrelevant pixels. For the experiments, we have collected a CXR image dataset of PICC, where we manually labelled the chest contours, PICC segmentation masks, and tip positions. We propose and evaluate our overall architectures by cross-validation on our PICC dataset. Extensive experiments demonstrate that the efficiency of our approach generates precise and reliable results and superior performance compared to other state-of-the-art networks. Compared to the baseline network (U-Net), MAG-Net achieves a 6% improvement in DSC (2% improvement in regional DSC), a 8% improvement in Recall (9% improvement in regional Recall), a 2% reduction in UR (9% reduction in regional UR) and a 5% improvement in IOU (2% improvement in regional IOU).The results show that our coarse-to-fine framework is effective to improve the segmentation accuracy by making good use of both the local and global contextual information. Our code is made publicly available at: https://github.com/zjutwly/muti-stage-PICC-segmentation.
更多查看译文
关键词
PICC segmentation,Multi-stage,Attention guided learning,Chest X-rays
AI 理解论文
溯源树
样例
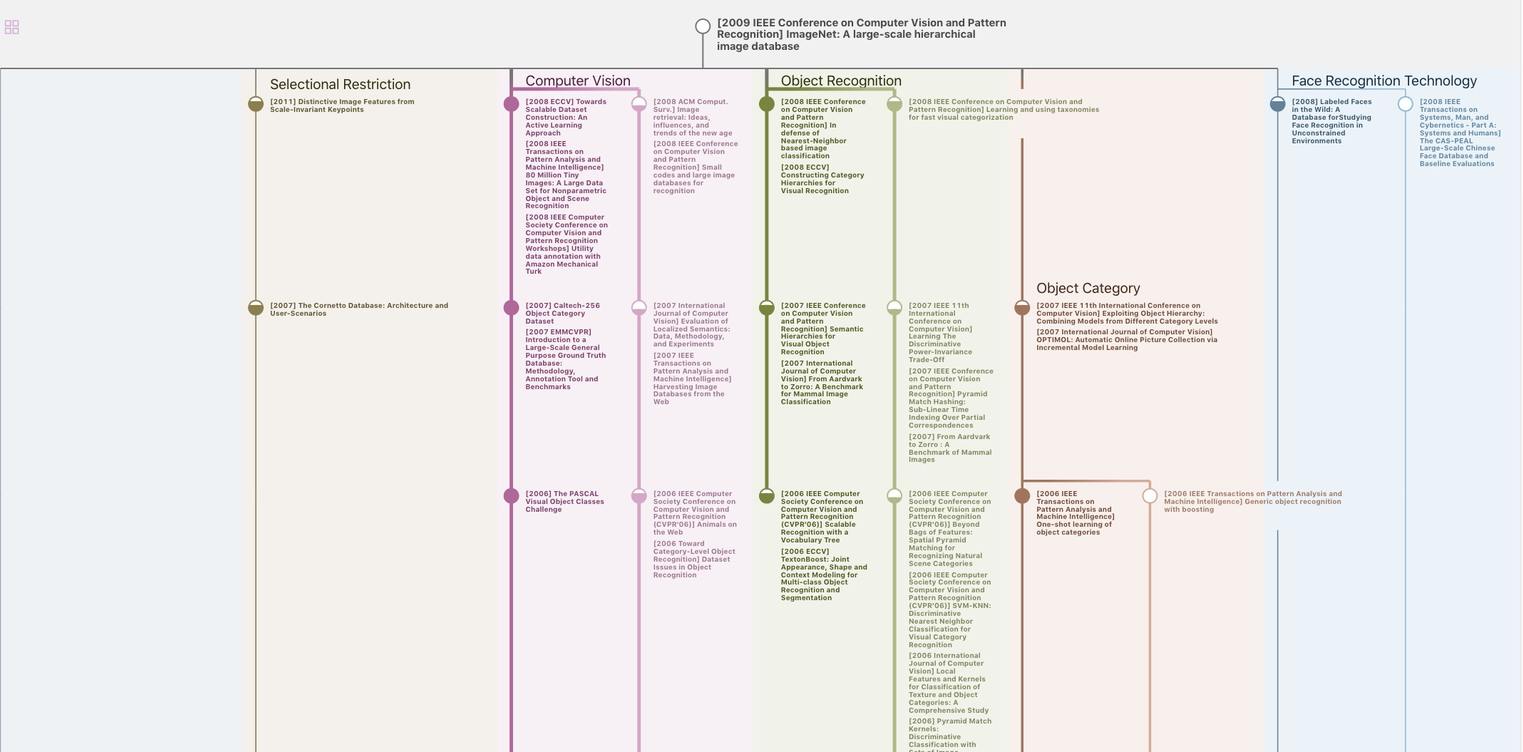
生成溯源树,研究论文发展脉络
Chat Paper
正在生成论文摘要