BigEye: Detection and Summarization of Key Global Events From Distributed Crowdsensed Data
IEEE Internet of Things Journal(2022)
摘要
Social media postings using smartphones (referred to as crowd-sensed data) can often facilitate real-time detection of key physical events in applications like disaster recovery or in smart cities. These postings also often contain visual content (e.g., images) that can be used to obtain zoomed-in views of such events. These crowd-sensed data are likely to be of large volume and distributed across a plurality of producers (e.g., cloudlets). Blindly transferring this large volume of raw data from the producers to a consumer will induce information overload and consume very high bandwidth. The problem is exacerbated in scenarios with limited bandwidth (e.g., after a disaster). In this article, we design
BigEye
, a novel framework that only transfers very limited data from the distributed producers to a central summarizer, and yet supports: 1) highly accurate detection and 2) concise visual summarization of key events of global interest. In realizing
BigEye
, we address several challenges including: 1) identifying events that have the highest global interest via the transfer of appropriate limited metadata from the producers to the summarizer; 2) reconciling metadata that could be inconsistent across the producers; and 3) the timely retrieval of visual summaries of the key events given bandwidth constraints. We show that
BigEye
achieves the same accuracy in detecting key events, as a system, where all data are available centrally while transferring only 1% of the raw data volume. Compared to the baseline approaches,
BigEye
’s parallelized transfer of visual content reduces the average delay by 67%.
更多查看译文
关键词
Crowd sensing,data summarization,distributed event detection,resource constrained networks,smart cities,social sensing,visual summaries
AI 理解论文
溯源树
样例
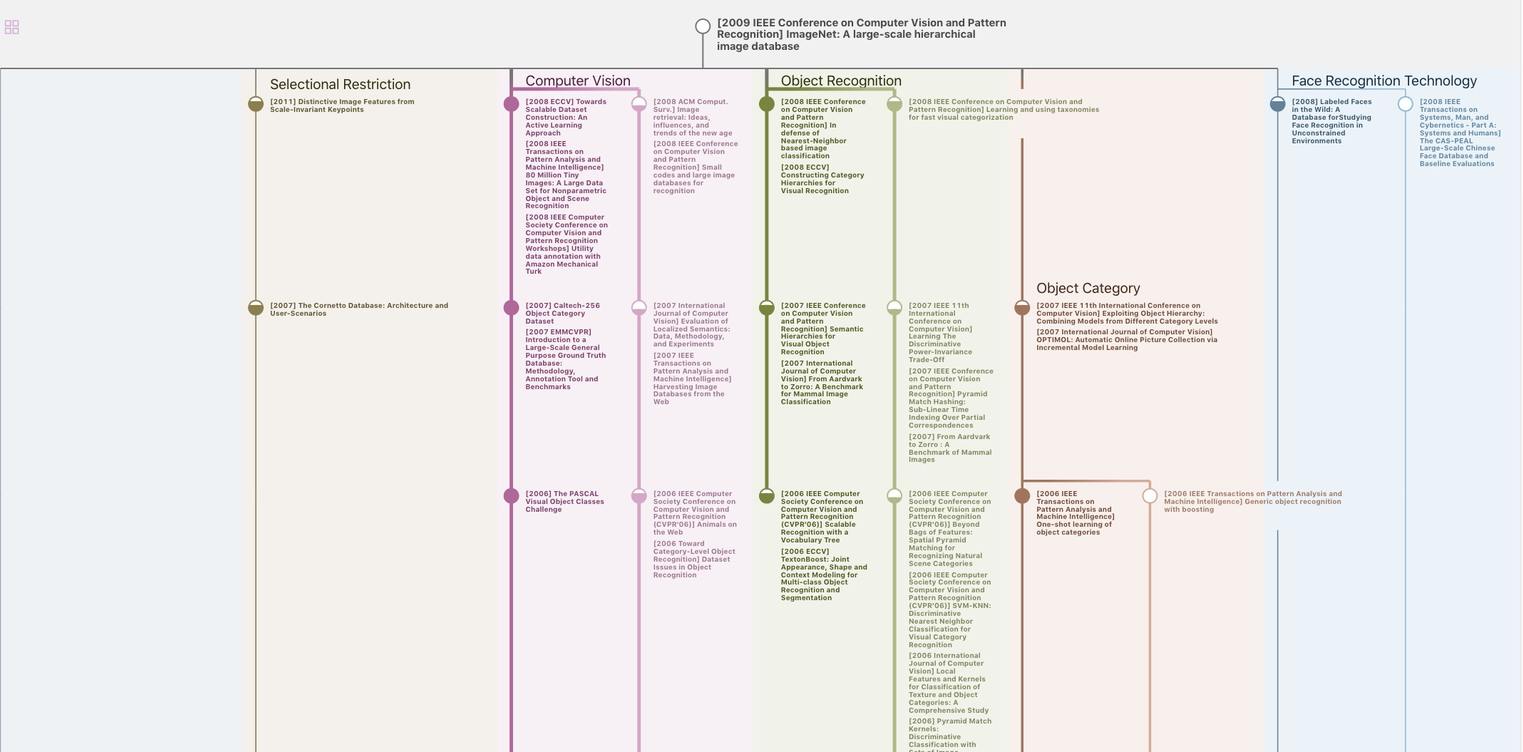
生成溯源树,研究论文发展脉络
Chat Paper
正在生成论文摘要