Mining Willing-to-Pay Behavior Patterns from Payment Datasets
ACM transactions on intelligent systems and technology(2022)
摘要
The customer base is the most valuable resource to E-commerce companies. A comprehensive understanding of customers’ preferences and behavior is crucial to developing good marketing strategies, in order to achieve optimal customer lifetime values (CLVs). For example, by exploring customer behavior patterns, given a marketing plan with a limited budget, a set of potential customers is able to be identified to maximize profit. In other words, personalized campaigns at the right time and in the right place can be treated as the last stage of consumption. Moreover, effective future purchase estimation and recommendation help guide the customer to the up-selling stage. The proposed willing-to-pay prediction model (W2P) exploits the transaction data to predict customer payment behavior based on a probabilistic graphical model, which provides semantic explanation of the estimated results and deals with the sparsity of payment data from each customer. Existing work in this domain ranks the customers by their probabilities of purchase in different conditions. However, the customer with the highest purchase probability does not necessarily spend the most. Therefore, we propose a CLV maximization algorithm based on the prediction results. In addition, we improve the model by behavioral segmentation wherein we group the customers by payment behaviors to reduce the size of the offline models and enhance the accuracy for low-frequency customers. The experiment results show that our model outperforms the state-of-the-art methods in purchase behavior prediction.
更多查看译文
关键词
Financial technology,customer lifetime value prediction,data sparsity
AI 理解论文
溯源树
样例
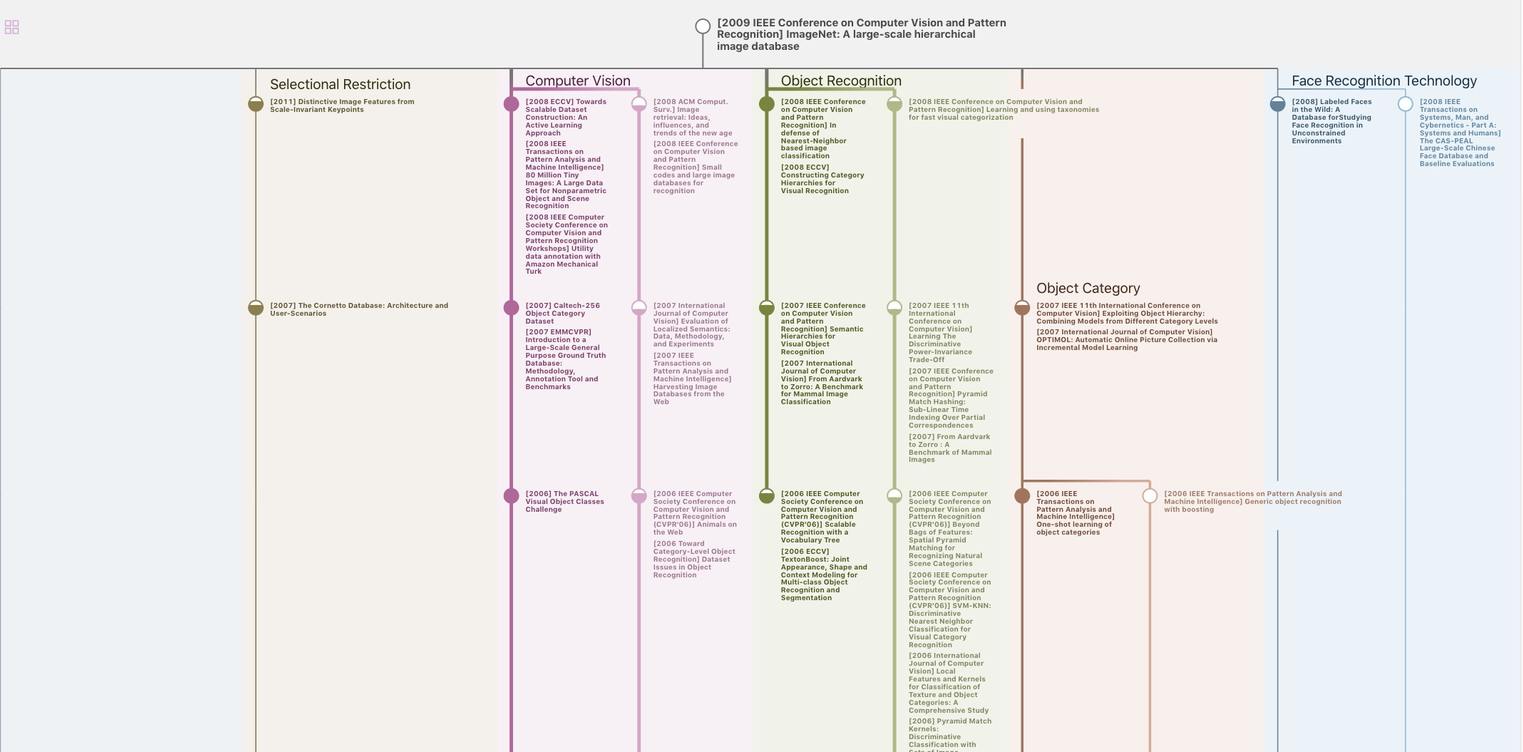
生成溯源树,研究论文发展脉络
Chat Paper
正在生成论文摘要