A semi-supervised autoencoder for autism disease diagnosis
Neurocomputing(2022)
摘要
Autism spectrum disorder (ASD) is a neurological developmental disorder that typically causes impaired communication and compromised social interactions. The current clinical assessment of ASD is typically based on behavioral observations and lack of the understanding of the neurological mechanism and the progression of the brain development. The functional magnetic resonance imaging (fMRI) data is one of the commonly-used imaging modalities for understanding human brain mechanisms as well as the diagnosis and treatment of brain disorders such as ASD. In this paper, we proposed a semi-supervised autoencoder (AE) for autism diagnosis using functional connectivity (FC) pattern obtained from resting-state fMRI. An unsupervised autoencoder in combination with the supervised classification networks enables semi-supervised learning in which an autoencoder for learning hidden features and a neural network based classifier are trained together. Compared to train the autoencoder and classifier in separate phases, the proposed semi-supervised learning essentially helps tune the latent feature representation learning towards the goal of classification, and thus leads to improvements in autism diagnosis performance. The proposed model is evaluated by using cross-validation methods on ABIDE I database. Experimental results demonstrate that the proposed model achieves improved classification performance, and that the proposed semi-supervised learning framework can integrate unlabelled fMRI data for better feature learning and improved classification accuracy.
更多查看译文
关键词
Artificial intelligence,Semi-supervised learning,Autoencoders,fMRI,Brain disorders diagnosis
AI 理解论文
溯源树
样例
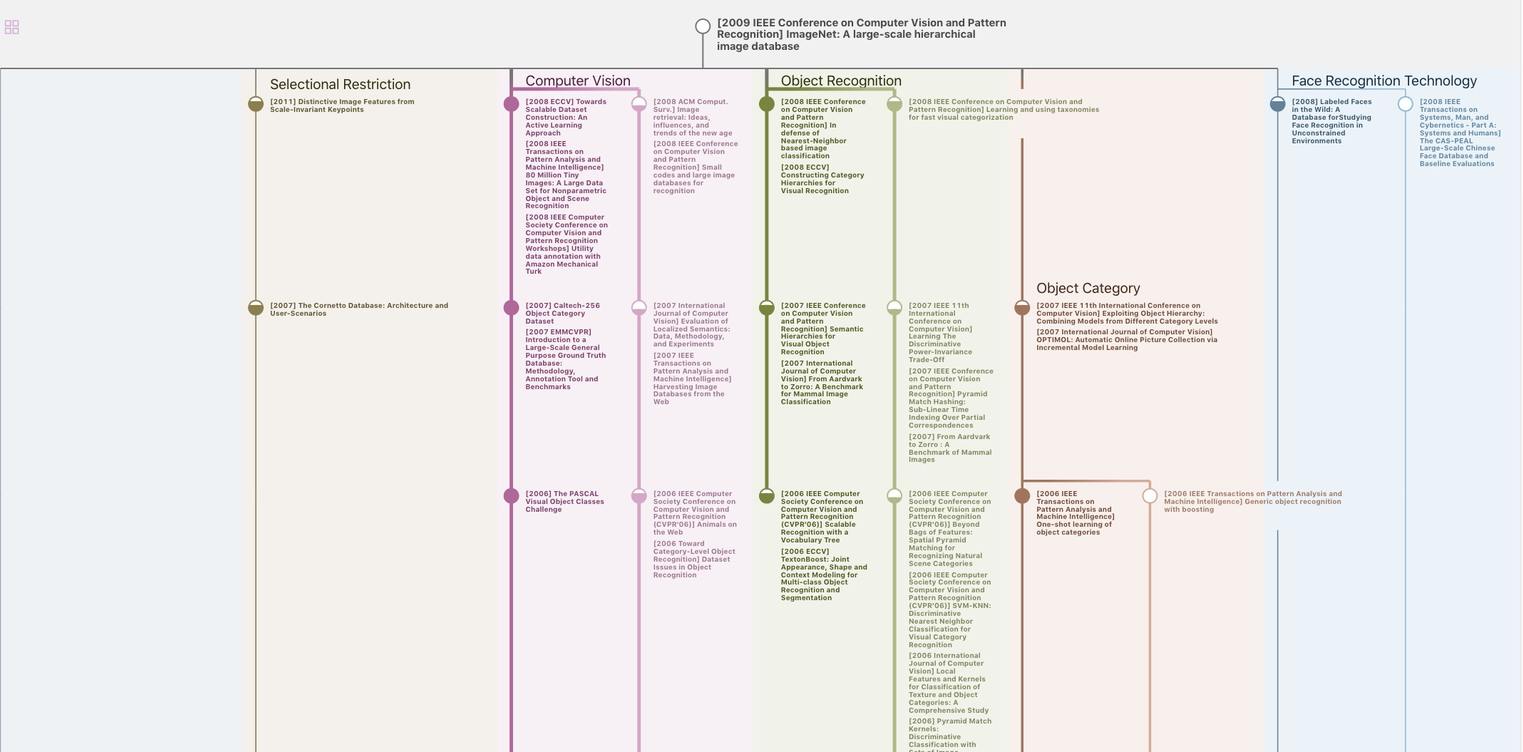
生成溯源树,研究论文发展脉络
Chat Paper
正在生成论文摘要