Adaptive Gaussian Process with PCA for Prediction of Complex Dispersion Relations for Periodic Structures
European journal of mechanics A, Solids/European journal of mechanics, A, Solids(2022)
摘要
The complex dispersion relation is important for understanding the wave propagation in periodic structures. However, its calculation is much more expensive compared to calculation of the real dispersion relation, due to the need to solve the dispersion equation at large number of frequencies. For uncertainty quantification in the dispersion relations, the computational challenge is even higher. This paper proposes an adaptive Gaussian process (AGP) model to efficiently predict the complex dispersion relations for periodic structures with different properties. Instead of directly building GP for the dispersion relation, which is challenging due to discontinuity in the dispersion relation, we first reformulate the problem as predicting the coefficients of the dispersion equation at selected frequencies, where these coefficients are continuous and smooth functions of the properties of periodic structures. Then GP model is trained to predict these coefficients, based on which the dispersion equation is then analytically solved to establish the complex dispersion relation. Second, Principal component analysis (PCA) is used to reduce the dimension of these coefficients to facilitate efficient training of GP model. Third, an adaptive procedure is integrated to iteratively add training data that can most effectively improve the GP model accuracy. Results verify the great efficiency and accuracy of the proposed approach for both undamped and damped periodic structures. The proposed approach has great promise in improving efficiency for uncertainty quantification, sensitivity analysis, and design optimization of periodic structures.
更多查看译文
关键词
Complex dispersion relations,Periodic structures,Adaptive Gaussian process,Surrogate model,Uncertainty quantification,Principal component analysis
AI 理解论文
溯源树
样例
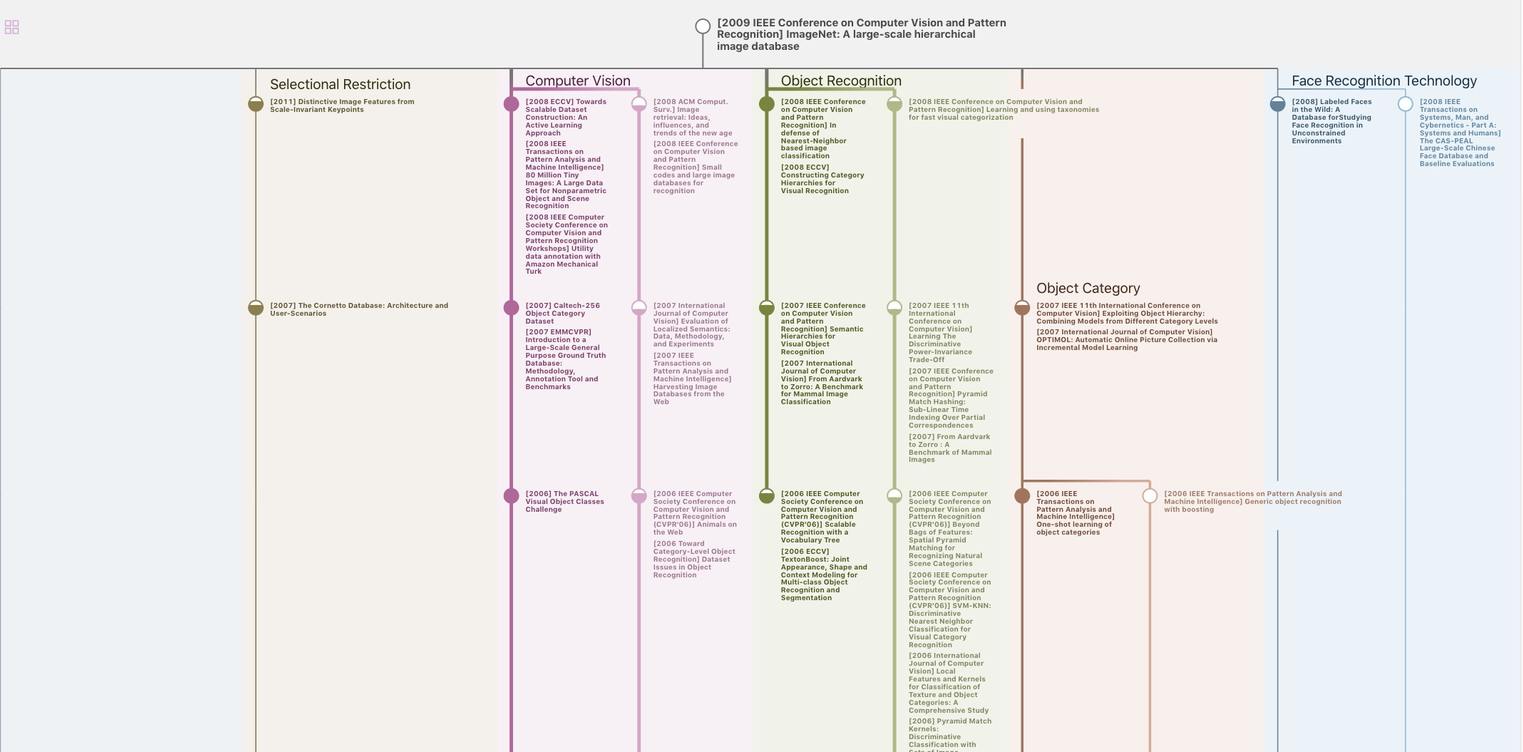
生成溯源树,研究论文发展脉络
Chat Paper
正在生成论文摘要