A Data-Driven Self-Optimization Solution for Inter-Frequency Mobility Parameters in Emerging Networks
IEEE Transactions on Cognitive Communications and Networking(2022)
摘要
Densification and multi-band operation means inter-frequency handovers can become a bottleneck for mobile user experience in emerging cellular networks. The challenge is aggravated by the fact that there does not exist a method to optimize key inter-frequency handover parameters namely A5 time-to-trigger, A5-threshold1 and A5-threshold2. This paper presents a first study to analyze and optimize the three A5 parameters for jointly maximizing three key performance indicators that reflect mobile user experience: handover success rate (HOSR), reference signal received power (RSRP), and signal-to-interference-plus-noise-ratio (SINR). As analytical modeling cannot capture the system-level complexity, we exploit a data-driven approach. To minimize the training data generation time, we exploit shapley additive explanations (SHAP) sensitivity analysis. The insights from SHAP analysis allow the selective collection of the training data thereby enabling the easier implementation of the proposed solution in a real network. We show that joint RSRP, SINR and HOSR optimization problem is non-convex and solve it using genetic algorithm (GA). We then propose an intelligent mutation scheme for GA, which makes the solution 5x times faster than the legacy GA and 21x faster than the brute force search. This paper thus presents first solution to implement computationally efficient closed-loop self-optimization of inter-frequency mobility parameters.
更多查看译文
关键词
Mobility Management,Handover Optimization,5G,6G,Machine Learning
AI 理解论文
溯源树
样例
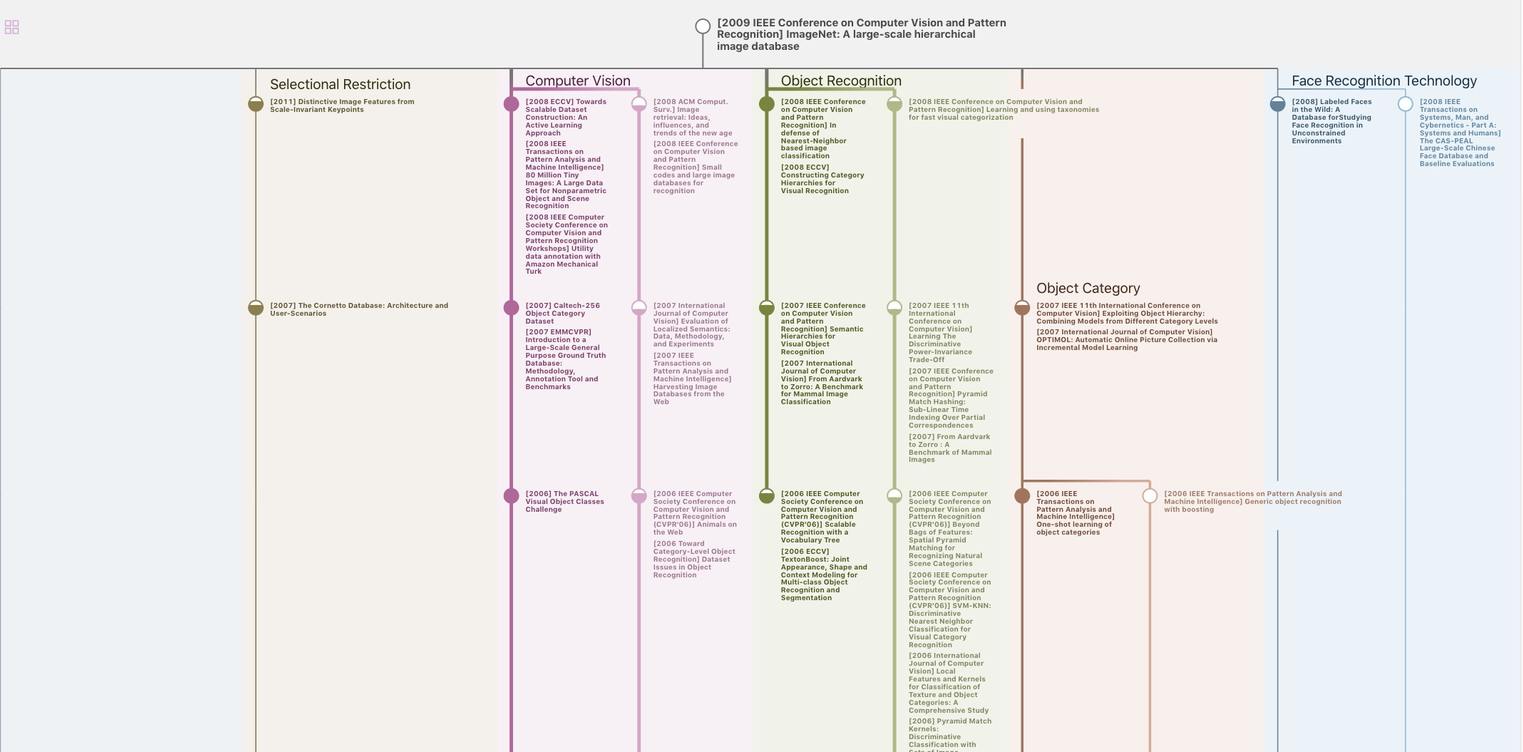
生成溯源树,研究论文发展脉络
Chat Paper
正在生成论文摘要