Data Assimilation Network for Generalizable Person Re-Identification
IEEE Transactions on Circuits and Systems for Video Technology(2022)
摘要
In this paper, a data assimilation network is proposed to tackle the challenges of domain generalization for person re-identification (ReID). Most of the existing research efforts only focus on single-dataset issues, and the trained models are difficult to generalize to unseen scenarios. This paper presents a distinctive idea to improve the generality of the model by assimilating three types of images: style-variant images, misaligned images and unlabeled images. The latter two are often ignored in the previous domain generalization ReID studies. In this paper, a non-local convolutional block attention module is designed for assimilating the misaligned images, and an attention adversary network is introduced to correct it. A progressive augmented memory is designed for assimilating the unlabeled images by progressive learning. Moreover, we propose an attention adversary difference loss for attention correction, and a labeling-guide discriminative embedding loss for progressive learning. Rather than designing a specific feature extractor that is robust to style shift as in most previous domain generalization work, we propose a data assimilation meta-learning procedure to train the proposed network, so that it learns to assimilate style-variant images. It is worth mentioning that we add an unlabeled augmented dataset to the source domain to tackle the domain generalization ReID tasks. Extensive experiments demonstrate that our approach significantly outperforms the state-of-the-art domain generalization methods.
更多查看译文
关键词
Person re-identification,data assimilation,attention correction,progressive augmented memory
AI 理解论文
溯源树
样例
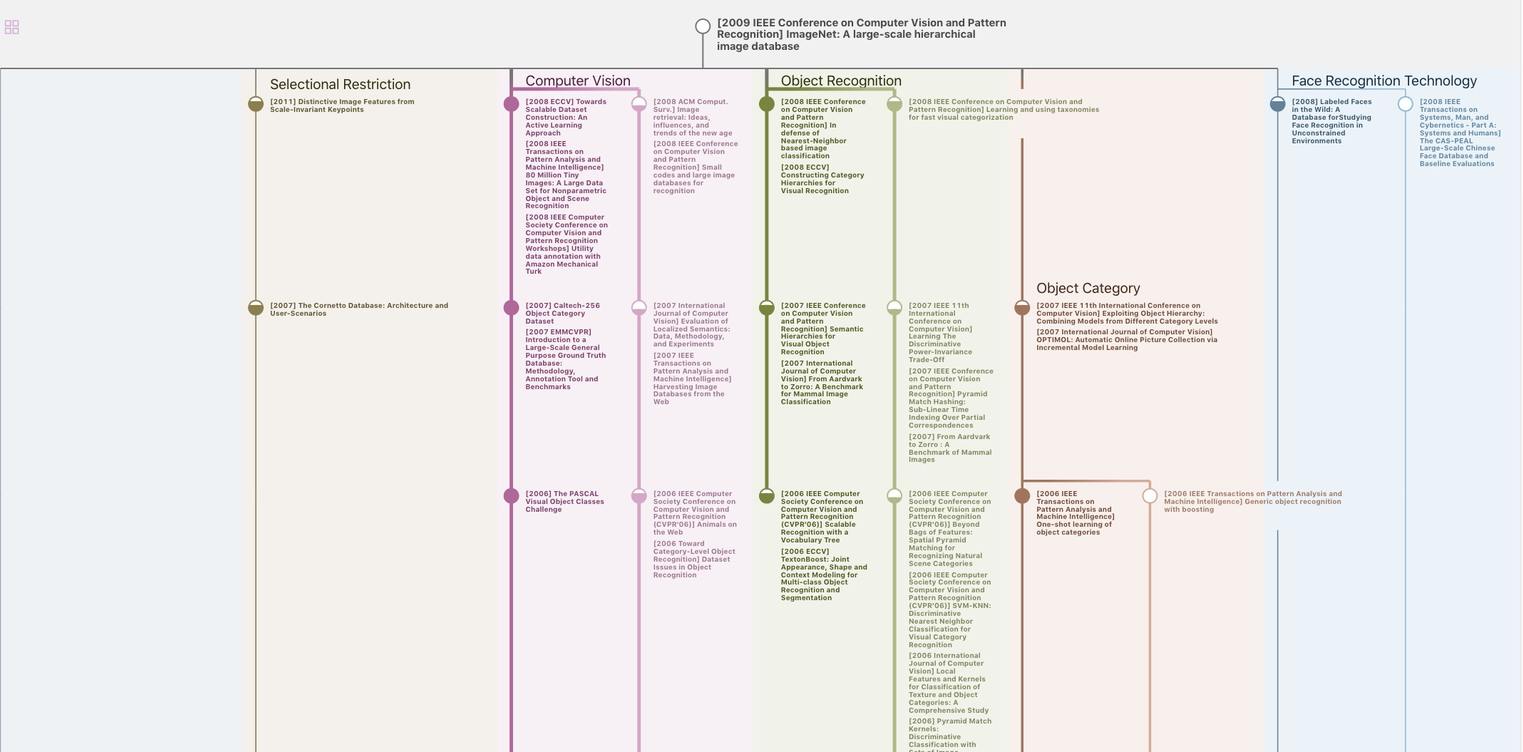
生成溯源树,研究论文发展脉络
Chat Paper
正在生成论文摘要