MulRNN: An Enhanced Technique of Multi-Stage-Attention Network for Complex Time Series Prediction
2021 4th International Conference on Algorithms, Computing and Artificial Intelligence(2021)
摘要
Multivariate time series prediction is still playing an important role in many fields such as finance, transportation and energy. It is still a challenging work to make long-term prediction of multivariate time series. The existing traditional methods based on statistics have a good prediction effect for the periodic data, but they can not capture the complex dependence between multiple time series and the prediction of long term. In this paper, we propose a new deep learning framework for complex sequence prediction based on multi-stage attentions, especially dynamic and non-periodic time series. MulRNN (Multi-Stage-Attention-RNN) uses convolutional neural network (CNN) and recurrent neural network (RNN) to extract short-term locally dependent patterns between variables, and introduces attention mechanisms to discover long-term patterns of time series. In addition, we use the traditional autoregressive model to solve the scale insensitivity problem of the neural network. Finally, we validate our proposed model on financial and energy datasets, and our method (MulRNN) achieves significant performance improvements over several advanced baseline methods in terms of time series prediction.
更多查看译文
AI 理解论文
溯源树
样例
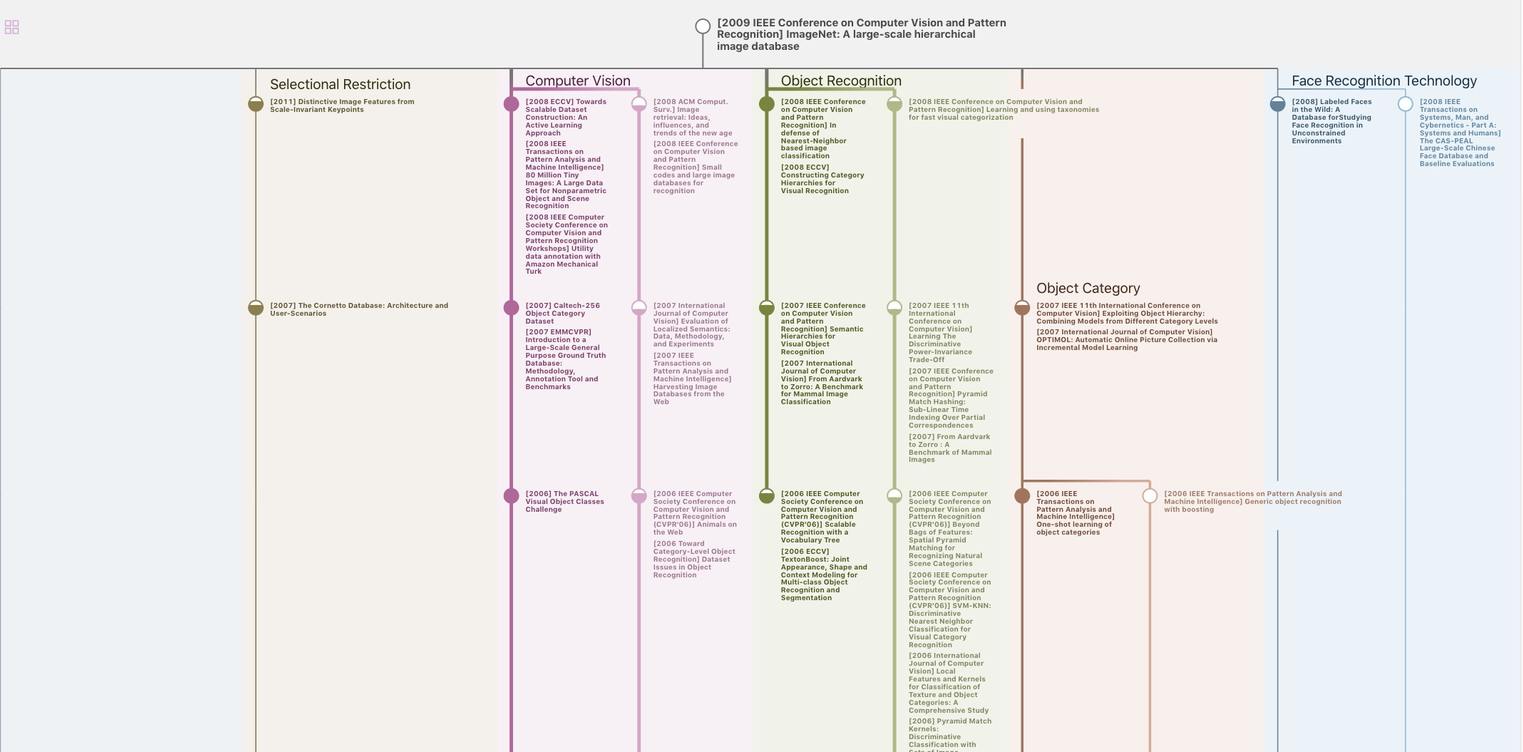
生成溯源树,研究论文发展脉络
Chat Paper
正在生成论文摘要