Implementation of MARS Metrics and MARS Charts for Evaluating Classifier Exclusivity: the Comparative Uniqueness of Binary Classifier Predictions
Software impacts(2022)
摘要
Traditionally, performance metrics such as accuracy, precision, recall, F-score, and ROC curve/ Area-Under-Curve (AUC) values have been used to evaluate and understand binary classifier capabilities. However, modern high-performance classifier models frequently have equivalent classification performance according to traditional metrics. We propose a novel approach – the MARS classifier evaluation method – to evaluate classifier exclusivity, using MARS ShineThrough and MARS Occlusion scores. Specifically, the MARS method vividly illustrates the extent to which classifiers spot distinct target-class observations, that other classifiers miss. In this paper, we describe the software artifact utilized to calculate MARS metrics for comparative uniqueness and MARS charts for the visualization of these calculations.
更多查看译文
关键词
Classifier performance evaluation,Classifier selection optimization
AI 理解论文
溯源树
样例
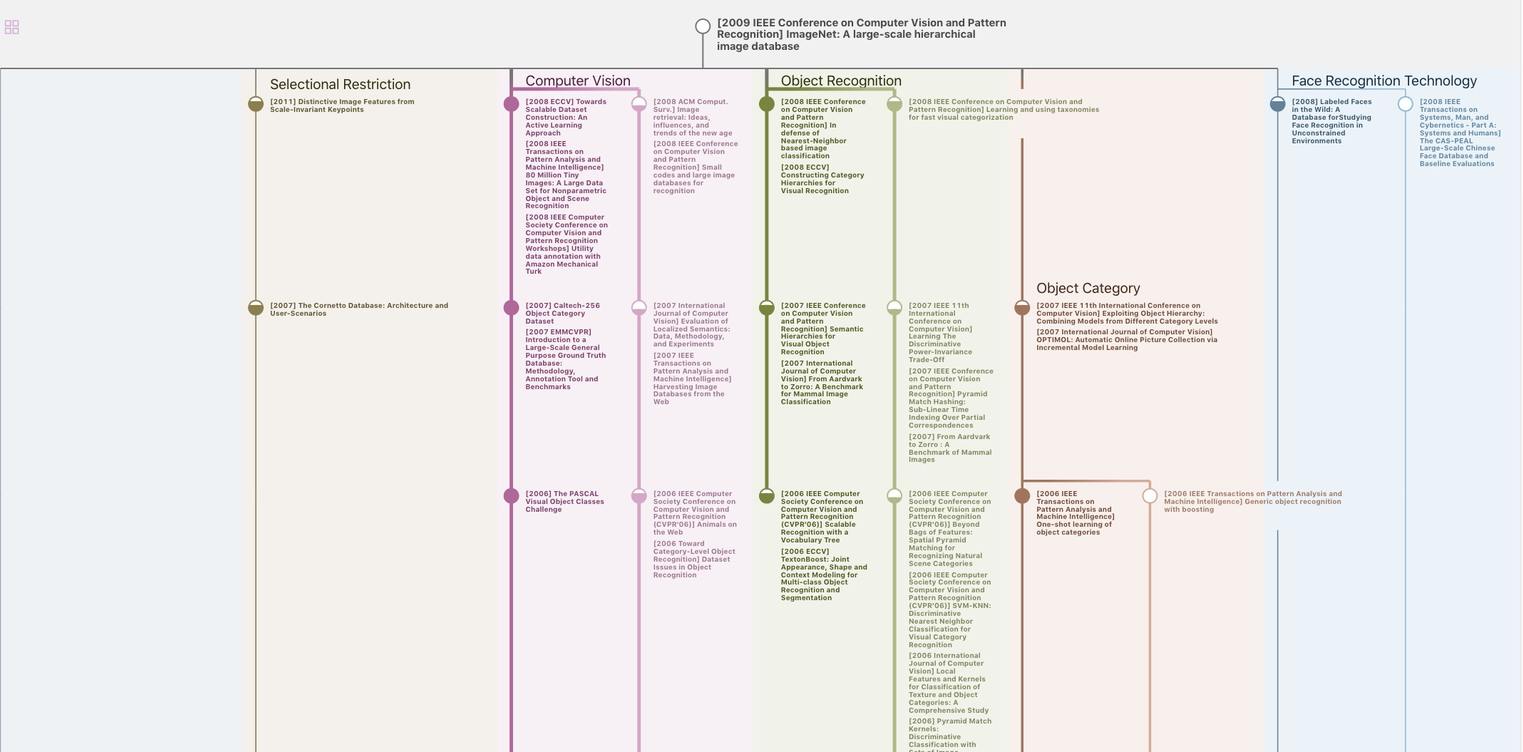
生成溯源树,研究论文发展脉络
Chat Paper
正在生成论文摘要