Learned Compression Framework With Pyramidal Features and Quality Enhancement for SAR Images
IEEE geoscience and remote sensing letters(2022)
摘要
Current image compression algorithms based on transforms can achieve ideal performance for natural images, but do not do well with synthetic aperture radar (SAR) images. We propose a learned compression framework with pyramidal features and quality enhancement to fully exploit the redundancy among image pixels and to improve the compression bitrate and reconstruction quality. Based on the variational autoencoder (VAE) architecture, pyramidal decomposition is performed at the first autoencoder to extract both global and coarse feature maps. The latent distribution is modeled by the second hyperprior autoencoder with a single-Gaussian model for more accurate and flexible entropy estimation. Universal quantization is applied to consolidate the entropy estimation accuracy of the hyperprior network. To further improve reconstruction quality, a residual dense network (RDN) is adopted to fully capture local and global features. Experimental results demonstrate that the proposed framework provides a better rate-distortion tradeoff than standard codecs such as JPEG, JPEG2000, and learning-based methods on both the Sandia and ICEYE datasets.
更多查看译文
关键词
Image coding,Feature extraction,Transform coding,Radar polarimetry,Quantization (signal),Decoding,Distortion,Image compression,pyramidal decomposition,remote sensing,residual dense network (RDN),variational autoencoder (VAE)
AI 理解论文
溯源树
样例
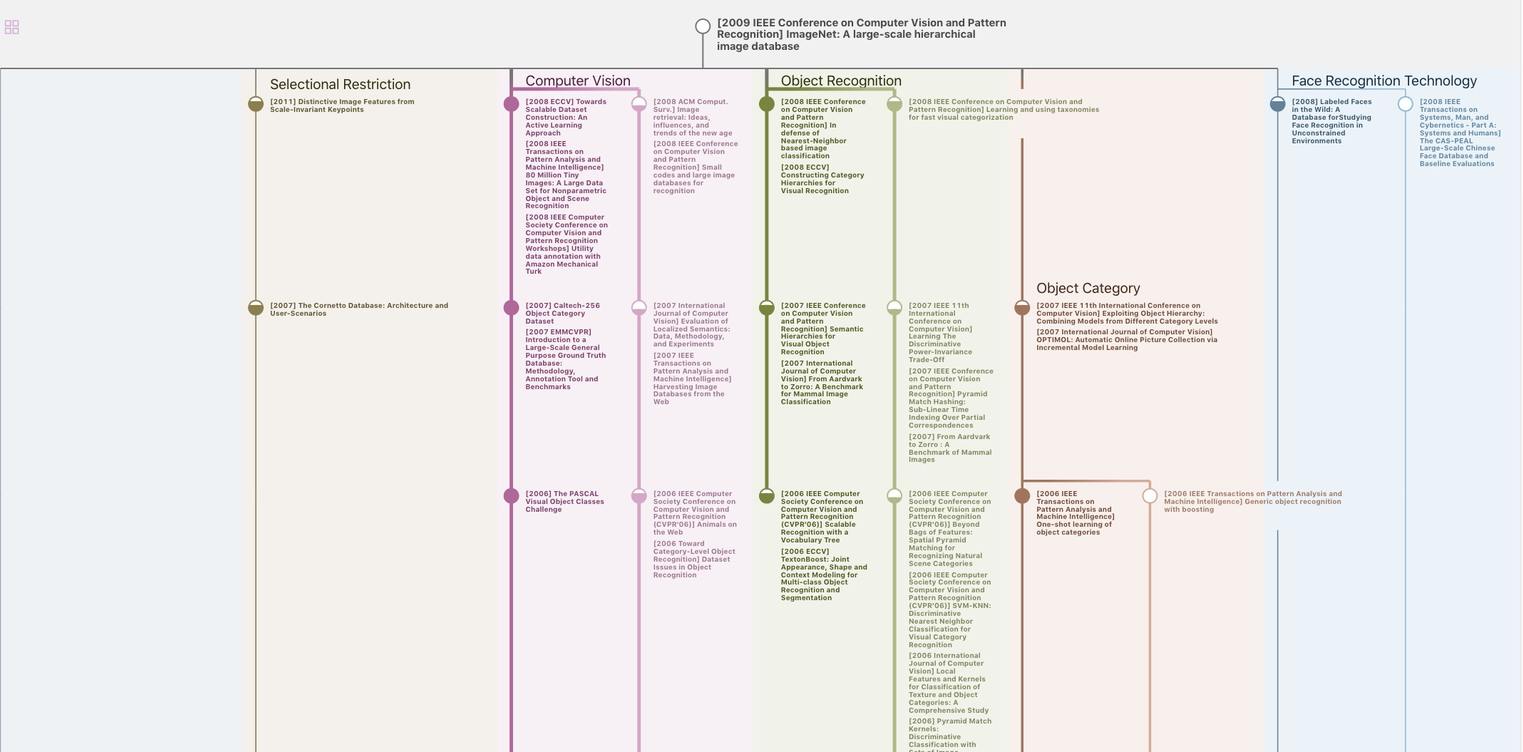
生成溯源树,研究论文发展脉络
Chat Paper
正在生成论文摘要