Hierarchical feature disentangling network for universal domain adaptation
Pattern Recognition(2022)
摘要
•We propose a novel Hierarchical Feature Disentangling Network (HFDN) for universal domain adaptation (UniDA), which is a more practical domain adaptation (DA) setting compared with close-set, open-set, and partial DA.•The proposed HFDN is the first to address the feature misalignment problem caused by both the domain gap and the category gap in UniDA.•With the disentangled features, we propose an innovated knowledge transfer approach for UniDA, which bridges the domain gap to reduce the domain-shift by domain adversarial training, and leverages information of category gap to assign larger weights for samples from the common label set.•Experiments demonstrate that our method achieves the best performance for UniDA on benchmark datasets compared with existing methods proposed under various domain adaptation assumptions.
更多查看译文
关键词
Universal domain adaptation,Feature disentanglement,Domain adversarial training,Sample reweighting
AI 理解论文
溯源树
样例
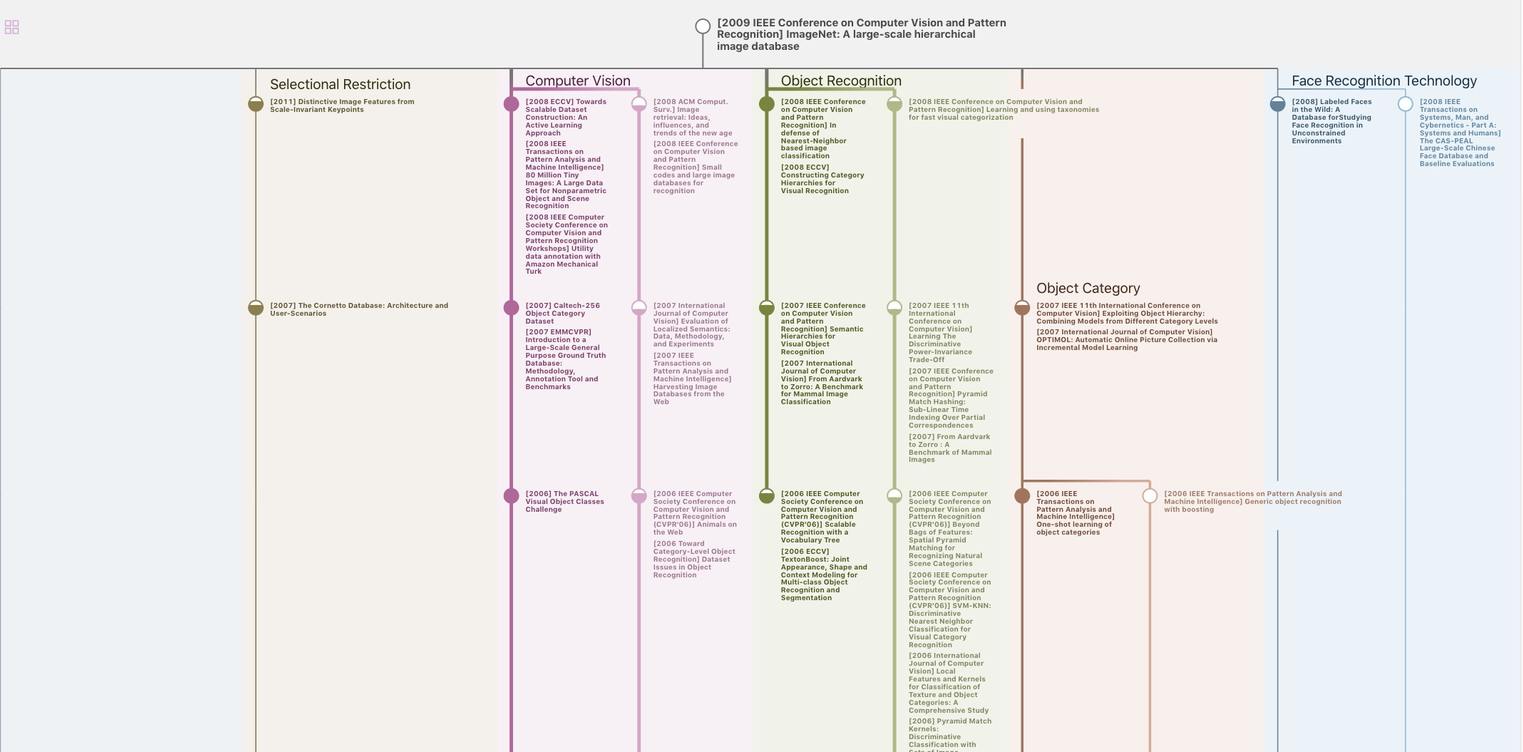
生成溯源树,研究论文发展脉络
Chat Paper
正在生成论文摘要