A controllable deep transfer learning network with multiple domain adaptation for battery state-of-charge estimation
APPLIED ENERGY(2022)
摘要
Deep learning models have been drawing significant attention in the literature of state-of-charge (SOC) estimation because of their capabilities to capture non-trivial temporal patterns. However, most of such models ignore cell-to-cell variations or focus on short-term estimations that are not practical for battery cells with limited charging-discharging history. We propose a Controllable Deep Transfer Learning (CDTL) network for short and long-term SOC estimations at early stages of degradation. The CDTL utilizes shared knowledge between the target cells of interest and historical source cells with rich SOC data using controllable Multiple Domain Adaptation (MDA). Specifically, the CDTL consists of two long-short term memory (LSTM) networks, the source LSTM, and the target LSTM. The source LSTM is trained on SOC data from historical battery cells. The target LSTM is then trained using limited available SOC data from the target cell and the transferred knowledge from the source LSTM using controllable MDA with adaptive regularization. The contributions of the CDTL are two-folded. First, it reduces the likelihood of negative transfer learning using controllable MDA with adaptive regularization, which enhances the target LSTM generalizability for long-term SOC estimation. Second, the CDTL offers theoretical guarantees on the controllability and convergence of transferred knowledge from the source cell to target cell. The experimental results demonstrate that the proposed CDTL outperforms existing deep and transfer learning benchmarks with 1) a maximum improvement of 60% in root-mean-squared error (RMSE) for battery cells with the same chemistry, 2) an average improvement of 50% in RMSE across different battery chemistries, and 3) about 39% reduction in computational time.
更多查看译文
关键词
Lithium-ion batteries, Deep learning model, Transfer learning, Multiple domain adaptation, State-of-charge
AI 理解论文
溯源树
样例
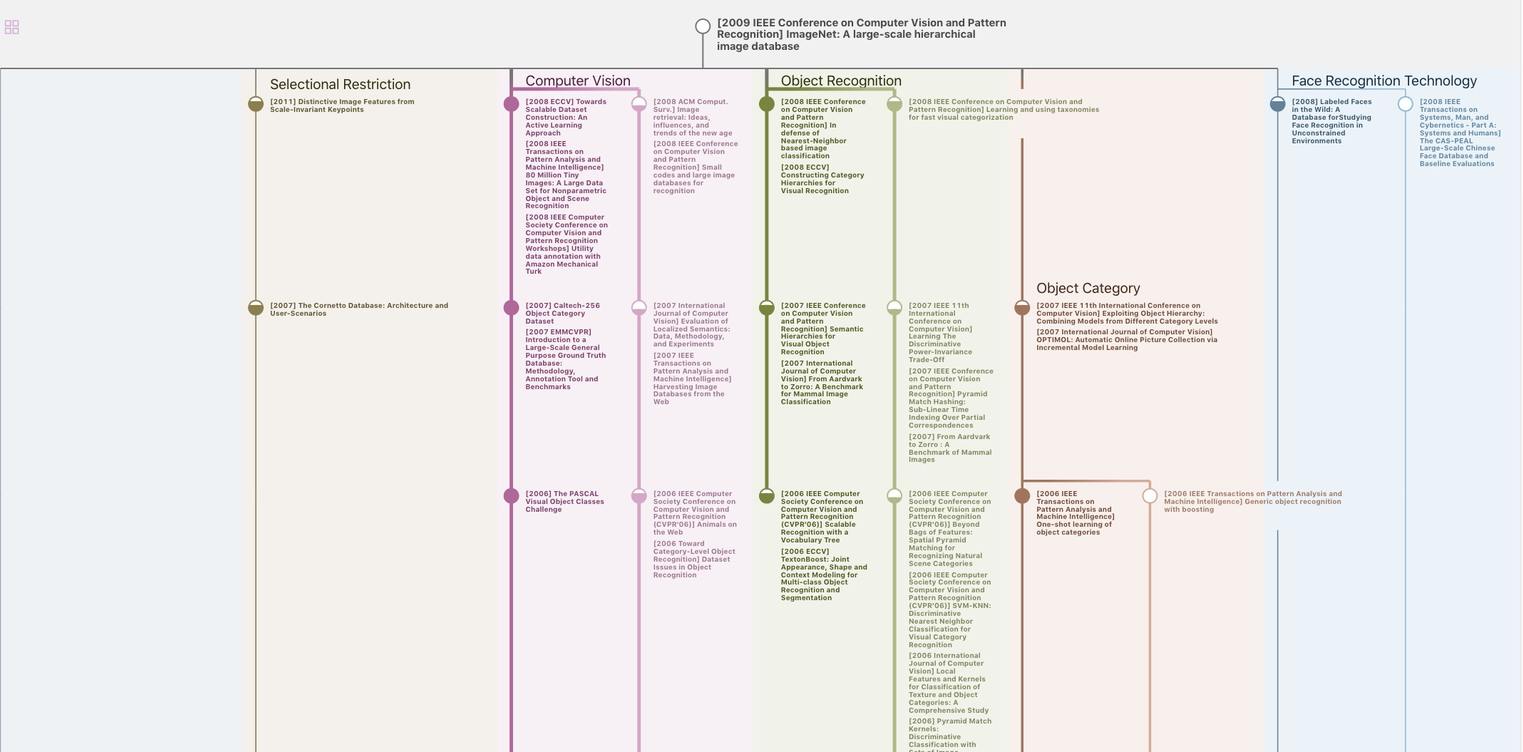
生成溯源树,研究论文发展脉络
Chat Paper
正在生成论文摘要