Class metric regularized deep belief network with sparse representation for fault diagnosis
INTERNATIONAL JOURNAL OF INTELLIGENT SYSTEMS(2022)
摘要
This paper proposes a joint class metric and sparse representation regularized deep belief network (J-DBN) method for intelligent fault diagnosis of the rotary equipment. In this novel method, the joint class metric and sparse representation regularized DBN is considered as a pretraining method to extract data features. It combines advantages of both class metric and sparse representation, which can optimize the distance of features in the same class and penalize the distance of features in different classes, and generate sparse features. Specifically, a new metric matrix is constructed to avoid using the same structural parameters for the local structure of each sample. The J-DBN-based fault diagnosis is implemented by the pretraining learning method, which contributes to better classification capabilities. Finally, gearbox and bearing fault diagnosis experiments are conducted to validate the effectiveness and the superiority of the proposed method. The results show that the ability of the J-DBN method to extract features is significantly enhanced, and the clustering of features of the same data is more obvious; furthermore, the proposed method has higher diagnostic accuracy than other fault diagnosis methods.
更多查看译文
关键词
class metric,deep belief network,fault diagnosis,feature extraction,sparse representation
AI 理解论文
溯源树
样例
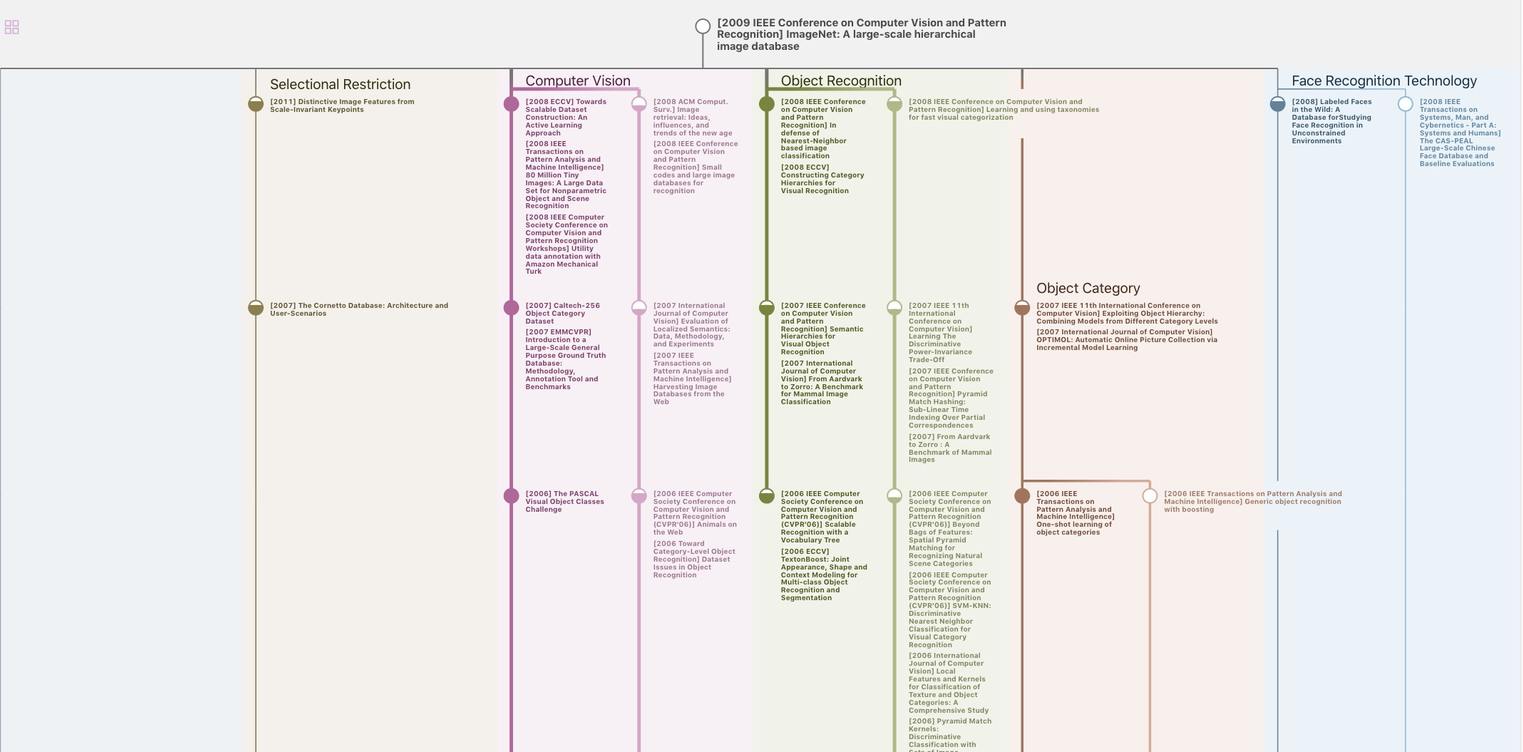
生成溯源树,研究论文发展脉络
Chat Paper
正在生成论文摘要