Optimized biodiesel production from c. inophyllum bio-oil using kriging and ann predictive models
Thermal Science(2022)
摘要
This work aimed at optimizing the two-stage transesterification efficiency of the production of C. inophyllum biodiesel using artificial neural network and Kriging predictive models. Response surface methodology was used to develop the central rotatable composite design of 27 trial experimental runs with variations in the input process parameters like methanol to oil molar ratio, potassium hydroxide catalyst loading, and reaction time. A multi-layered non-linear regressive artificial neural network model with feed-forward propagation and a numerical surrogate Kriging model was used to predict the C. inophyllum biodiesel yield. The efficacy of the developed model was verified using analysis of variance by comparing its coefficient of determination and the mean relative percentage deviation values. The optimized C. inophyllum biodiesel as 98.1% is derived with 0.94 v/v of methanol to oil molar ratio, 0.98 wt.% of potassium hydroxide catalyst loading, and 80 minutes reaction time with 70 degrees C constant reaction temperature as predicted by Kriging model. The optimized parameters were also verified experimentally.
更多查看译文
关键词
transesterification,reaction time,Molar ratio,biodiesel yield,optimization
AI 理解论文
溯源树
样例
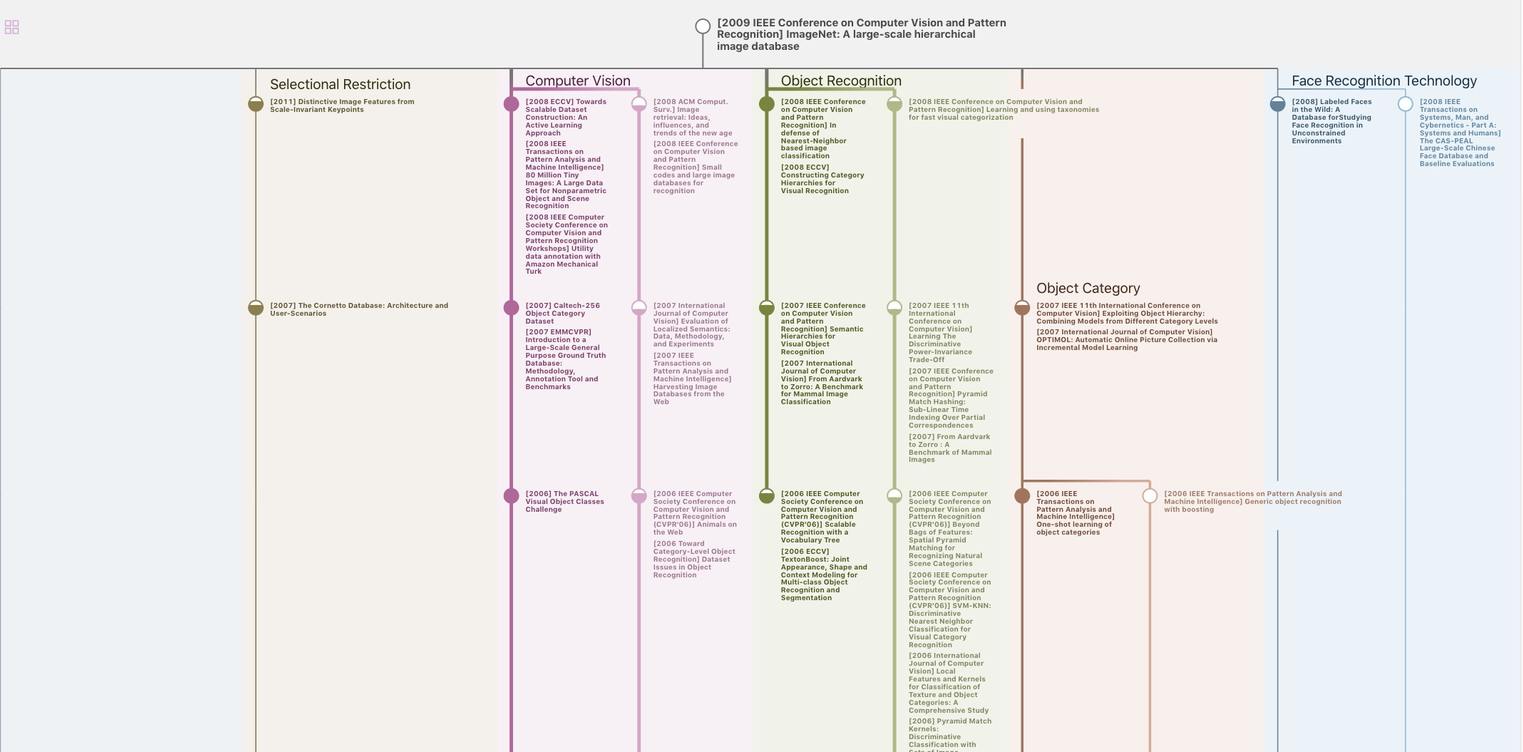
生成溯源树,研究论文发展脉络
Chat Paper
正在生成论文摘要