A metric for quantifying nonlinearity in k-dimensional complex-valued functions
JOURNAL OF DEFENSE MODELING AND SIMULATION-APPLICATIONS METHODOLOGY TECHNOLOGY-JDMS(2024)
摘要
Modeling and simulation is a proven cost-efficient means for studying the behavioral dynamics of modern systems of systems. Our research is focused on evaluating the ability of neural networks to approximate multivariate, nonlinear, complex-valued functions. In order to evaluate the accuracy and performance of neural network approximations as a function of nonlinearity (NL), it is required to quantify the amount of NL present in the complex-valued function. In this paper, we introduce a metric for quantifying NL in multi-dimensional complex-valued functions. The metric is an extension of a real-valued NL metric into the k-dimensional complex domain. The metric is flexible as it uses discrete input-output data pairs instead of requiring closed-form continuous representations for calculating the NL of a function. The metric is calculated by generating a best-fit, least-squares solution (LSS) linear k-dimensional hyperplane for the function; calculating the L2 norm of the difference between the hyperplane and the function being evaluated; and scaling the result to yield a value between zero and one. The metric is easy to understand, generalizable to multiple dimensions, and has the added benefit that it does not require a closed-form continuous representation of the function being evaluated.
更多查看译文
关键词
Nonlinearity metric,complex-valued functions,modeling,simulation
AI 理解论文
溯源树
样例
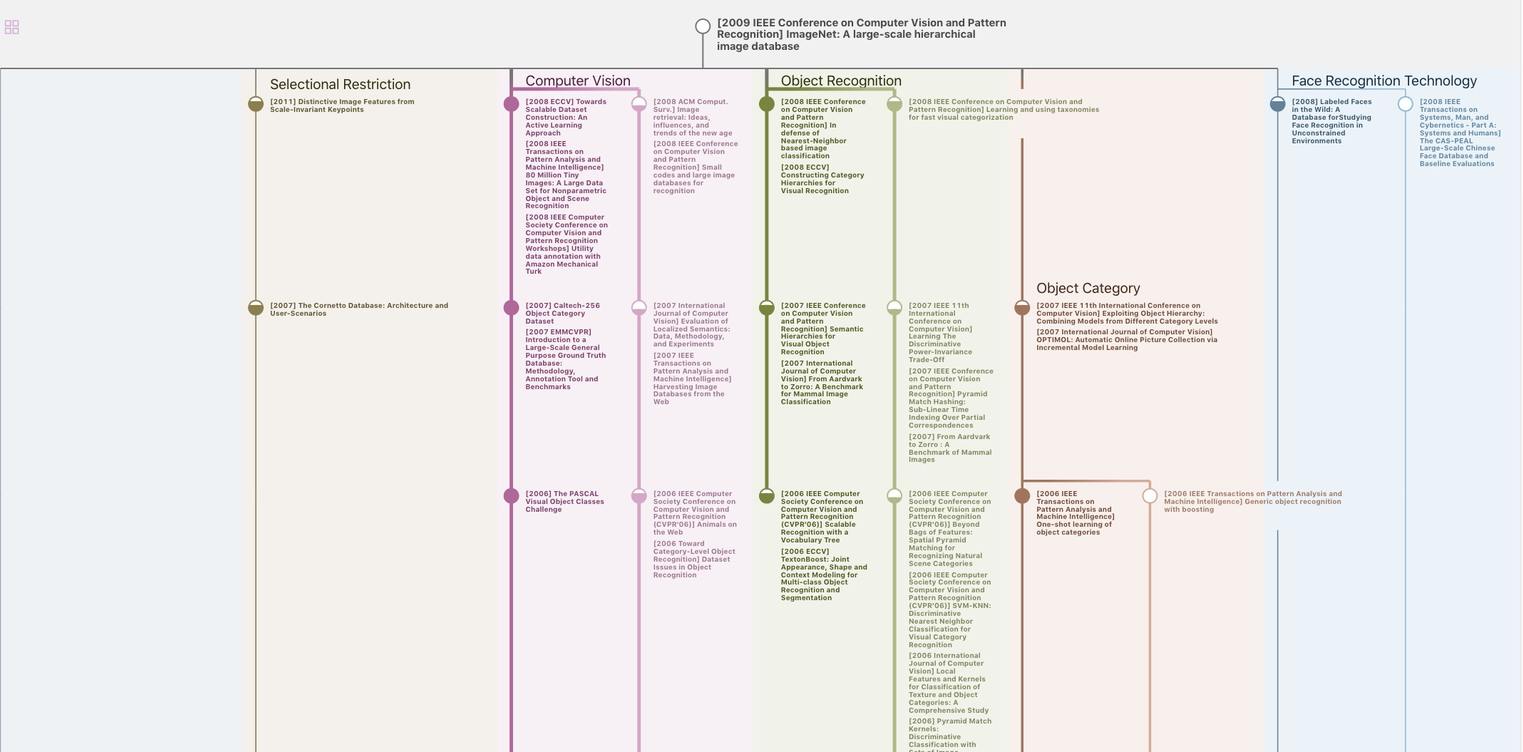
生成溯源树,研究论文发展脉络
Chat Paper
正在生成论文摘要