Local Model-Agnostic Explanations for Black-box Recommender Systems Using Interaction Graphs and Link Prediction Techniques
International journal of interactive multimedia and artificial intelligence(2023)
摘要
Explanations in recommender systems are a requirement to improve users’ trust and experience. Traditionally, explanations in recommender systems are derived from their internal data regarding ratings, item features, and user profiles. However, this information is not available in black-box recommender systems that lack sufficient data transparency. This current work proposes a local model-agnostic, explanation-by-example method for recommender systems based on knowledge graphs to leverage this knowledge requirement. It only requires information about the interactions between users and items. Through the proper transformation of these knowledge graphs into item-based and user-based structures, link prediction techniques are applied to find similarities between the nodes and to identify explanatory items for the user’s recommendation. Experimental evaluation demonstrates that these knowledge graphs are more effective than classical content-based explanation approaches but have lower information requirements, making them more suitable for black-box recommender systems.
更多查看译文
关键词
Black-box Recommender Systems,Explainable Artificial Intelligence,Graph Knowledge,Graph Representation,Link Prediction Techniques
AI 理解论文
溯源树
样例
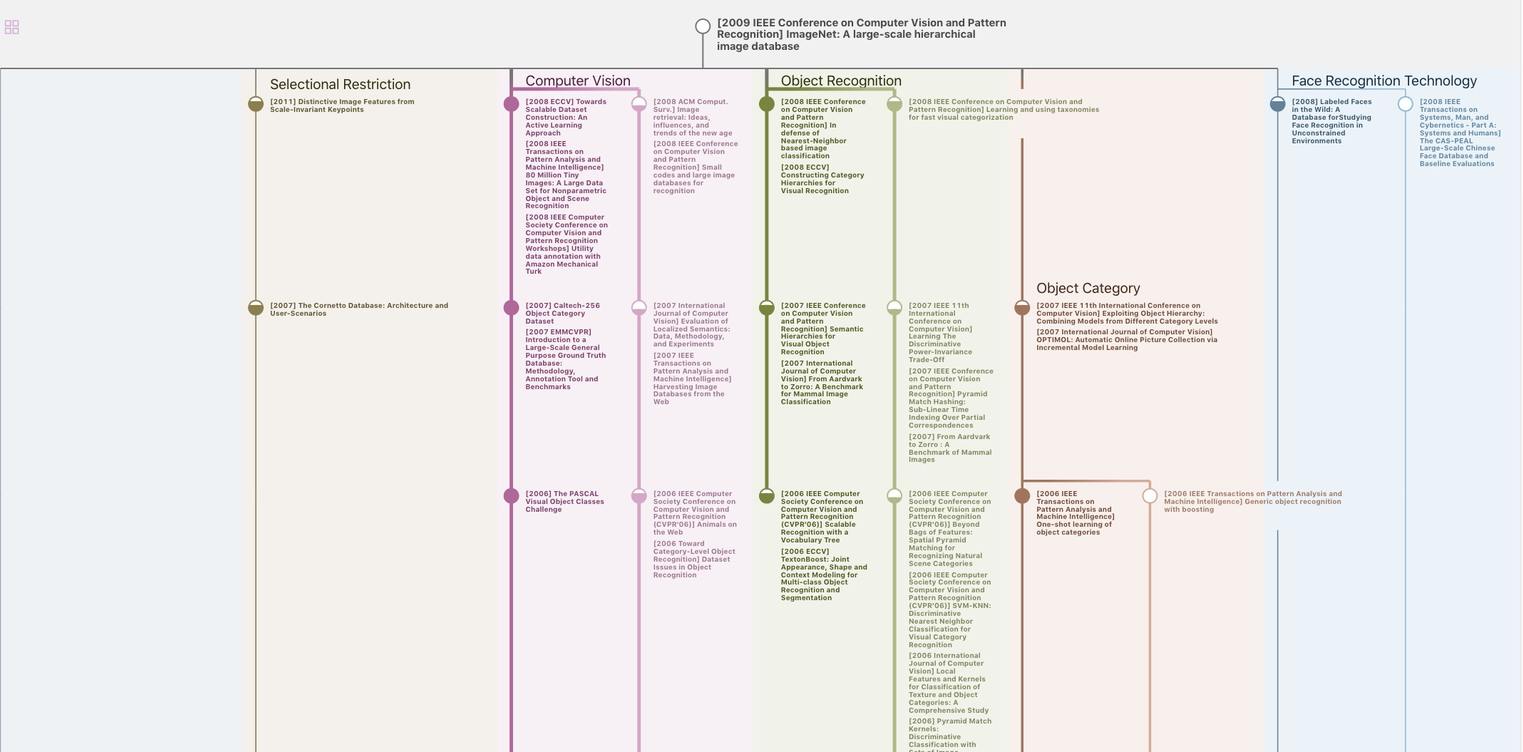
生成溯源树,研究论文发展脉络
Chat Paper
正在生成论文摘要