Toward a Robust, Impact‐Based, Predictive Drought Metric
Water resources research(2022)
摘要
This work presents a new approach to defining drought by establishing an empirical relationship between historical droughts (and wet spells) documented in impact reports, and a broad range of observed climate features using Random Forest (RF) models. The new drought indicator quantifies the conditional probability of drought, considering multiple drought‐related climate features and their interactive effects, and can be used for forecasting with up to 3‐month lead time. The approach was tested out‐of‐sample across several random selections of training and testing datasets, and demonstrated better predictive capabilities than commonly used drought indicators (e.g., Standardised Precipitation Index and Evaporative Demand Drought Index) in a range of performance metrics. Furthermore, it showed comparable performance to the (expert elicitation‐based) US Drought Monitor (USDM), the current state‐of‐the‐art record of historical drought in the USA. As well as providing an alternative historical drought indicator to USDM, the RF approach offers additional advantages by being automated, by providing drought information at the grid‐scale, and by having forecasting capacity. While traditional drought metrics define drought as extreme anomalies in drought‐related variables, the approach presented here reveals the full suite of circumstances that lead to impactful droughts. We highlight several combinations of climate features—such as precipitation, potential evapotranspiration, soil moisture and change in water storage—that led to drought events not detected by commonly used drought metrics. The new RF drought indicator combines meteorological, hydrological, agricultural, and socioeconomic drought, providing drought information for all impacted sectors. As a proof‐of‐concept, the RF drought indicator was trained on Texan climate data and droughts.
更多查看译文
关键词
drought,machine learning,impacts data
AI 理解论文
溯源树
样例
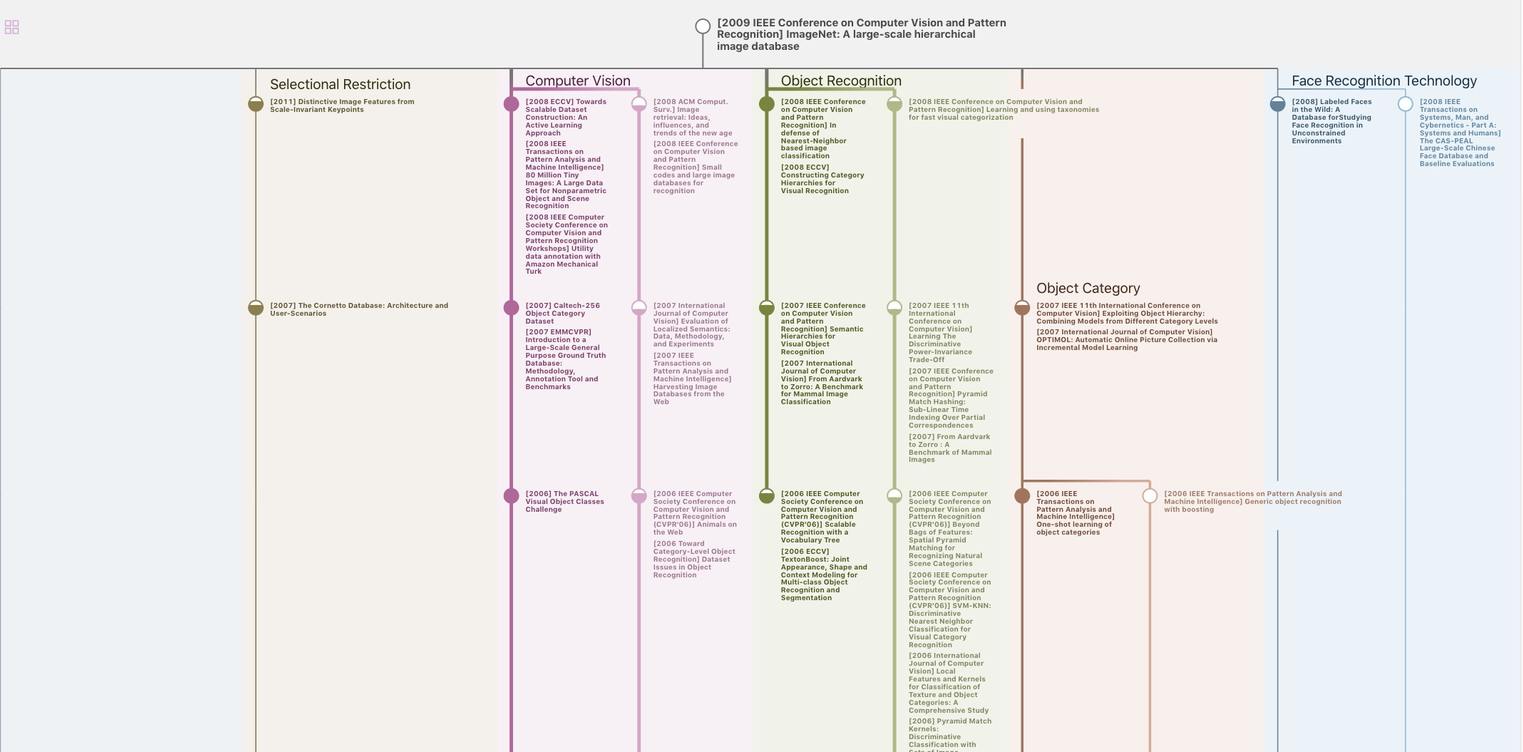
生成溯源树,研究论文发展脉络
Chat Paper
正在生成论文摘要