A Deep Learning Method Based on Bidirectional Wavenet for Voltage Sag State Estimation Via Limited Monitors in Power System
SSRN Electronic Journal(2021)
摘要
Voltage sag state estimation on the basis of a limited number of installed monitors is essential to dividing the responsibility for the voltage sag and taking corresponding measurements for improvement in voltage quality. Therefore, a deep learning methodology via bidirectional WaveNet for the voltage sag state estimation is proposed in this paper. The presented method can simultaneously estimate voltage sag state at non-monitored buses via limited monitors. Especially, the proposed deep learning method using the bidirectional WaveNet is designed to explore the long-term and long-range temporal dependencies in both the forward and backward directions. In this way, only by using original measured voltages through monitors, high accuracy for voltage sag state estimation can be achieved without restructured or redesign of the raw monitored data. An excellent advantage of the presented algorithm is that it can be implemented without system parameters or operating conditions or any other prior information. The presented methodology was verified by the IEEE 30-bus benchmark system. The experimental results illustrated that the accuracy of the voltage sag state estimation results was over 99.83%. Furthermore, a comparison among different models, including the bidirectional GRU-based model, one-way WaveNet-based model, and bidirectional WaveNet-based model, was also conducted. The results illustrated that the proposed bidirectional WaveNet-based model achieved the highest accuracy and quickest convergence speed.
更多查看译文
关键词
power quality, voltage sag, state estimation, deep learning, bidirectional WaveNet
AI 理解论文
溯源树
样例
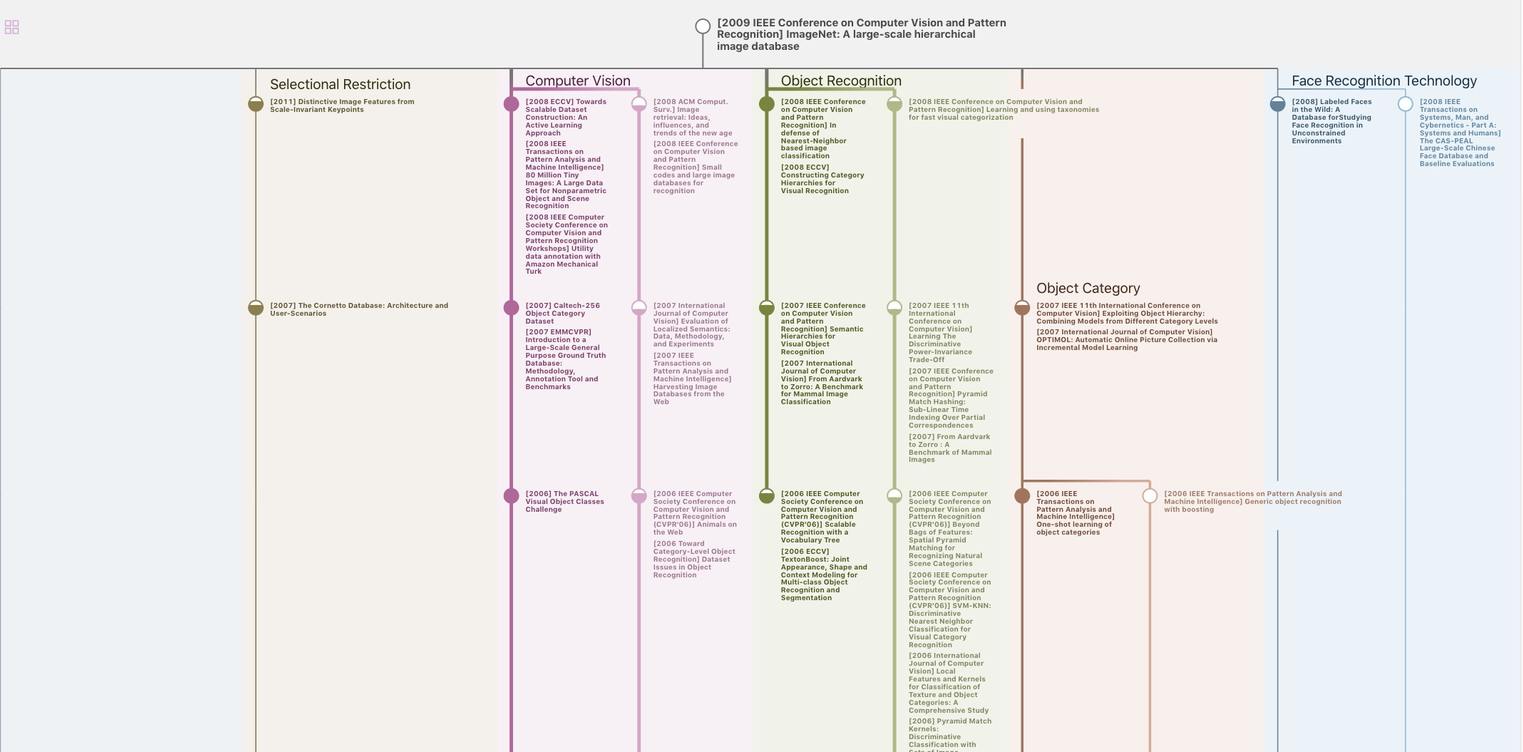
生成溯源树,研究论文发展脉络
Chat Paper
正在生成论文摘要