Data Augmentation for Intelligent Contingency Management Using Generative Adversarial Networks
AIAA SCITECH 2022 Forum(2022)
摘要
Artificial intelligence (AI)-based techniques for intelligent contingency management (ICM) require that intelligent agents learn various aspects of system dynamics to create and execute contingencies. For high assurance contingency management, agents achieve the most compelling results through supervised or semi-supervised machine learning, for which agents require large datasets to learn the dynamics of the system. Unfortunately, data collection in aerospace applications can be costly, due to both time and resources. Presented work describes a framework for data augmentation of ICM databases containing training data for machine learning models. This framework populates the database with the outputs of generative adversarial network (GAN) models that were trained on flight data. Methods for evaluating the suitability of these models based on the equations of motion, as well as other physical constraints, are discussed. The paper demonstrates the utility of this database for training intelligent agents on the NASA T2 generic transport aircraft model and experimental vertical takeoff and landing (VTOL) simulation model.
更多查看译文
AI 理解论文
溯源树
样例
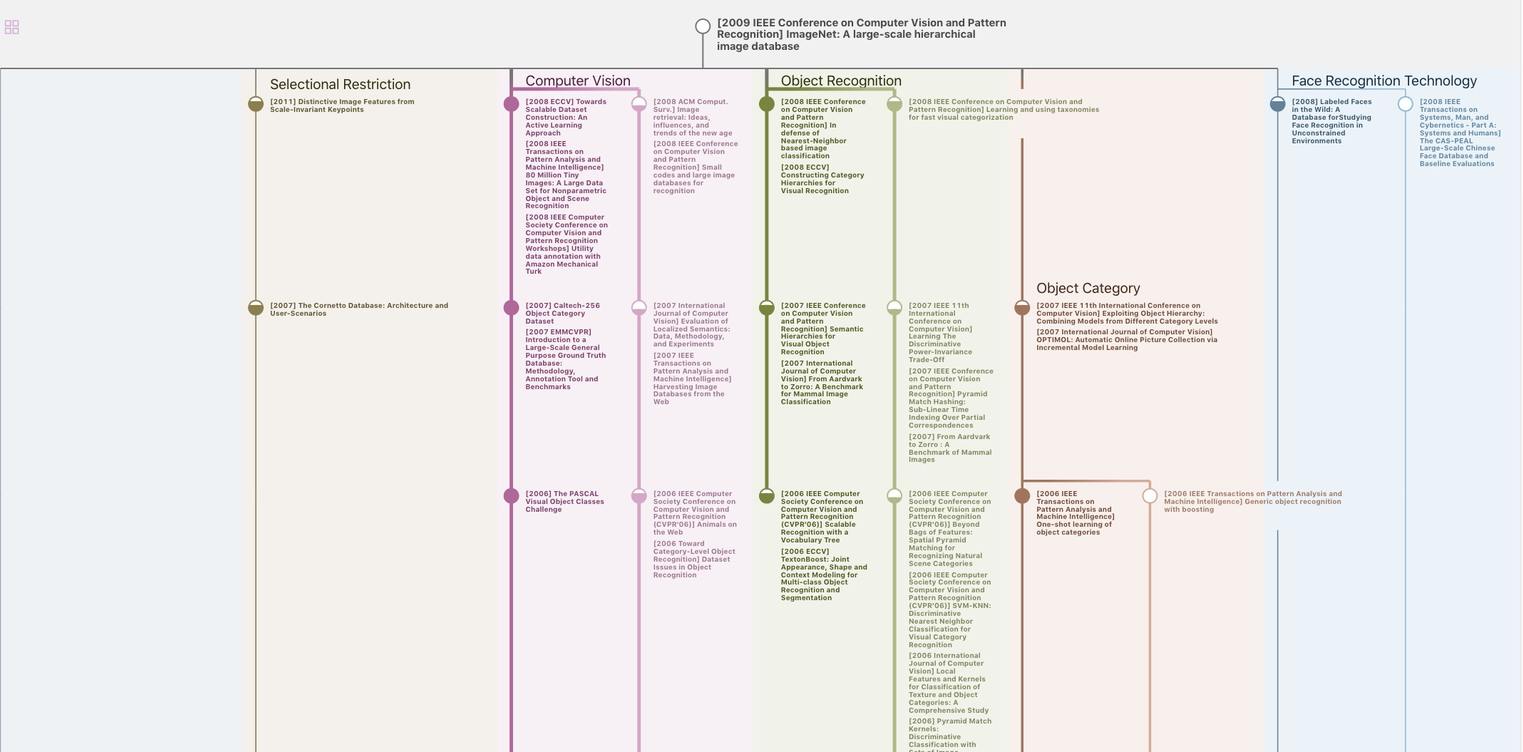
生成溯源树,研究论文发展脉络
Chat Paper
正在生成论文摘要