Efficient Depth Fusion Transformer for Aerial Image Semantic Segmentation
REMOTE SENSING(2022)
摘要
Taking depth into consideration has been proven to improve the performance of semantic segmentation through providing additional geometry information. Most existing works adopt a two-stream network, extracting features from color images and depth images separately using two branches of the same structure, which suffer from high memory and computation costs. We find that depth features acquired by simple downsampling can also play a complementary part in the semantic segmentation task, sometimes even better than the two-stream scheme with the same two branches. In this paper, a novel and efficient depth fusion transformer network for aerial image segmentation is proposed. The presented network utilizes patch merging to downsample depth input and a depth-aware self-attention (DSA) module is designed to mitigate the gap caused by difference between two branches and two modalities. Concretely, the DSA fuses depth features and color features by computing depth similarity and impact on self-attention map calculated by color feature. Extensive experiments on the ISPRS 2D semantic segmentation dataset validate the efficiency and effectiveness of our method. With nearly half the parameters of traditional two-stream scheme, our method acquires 83.82% mIoU on Vaihingen dataset outperforming other state-of-the-art methods and 87.43% mIoU on Potsdam dataset comparable to the state-of-the-art.
更多查看译文
关键词
semantic segmentation,self-attention,depth fusion,transformer
AI 理解论文
溯源树
样例
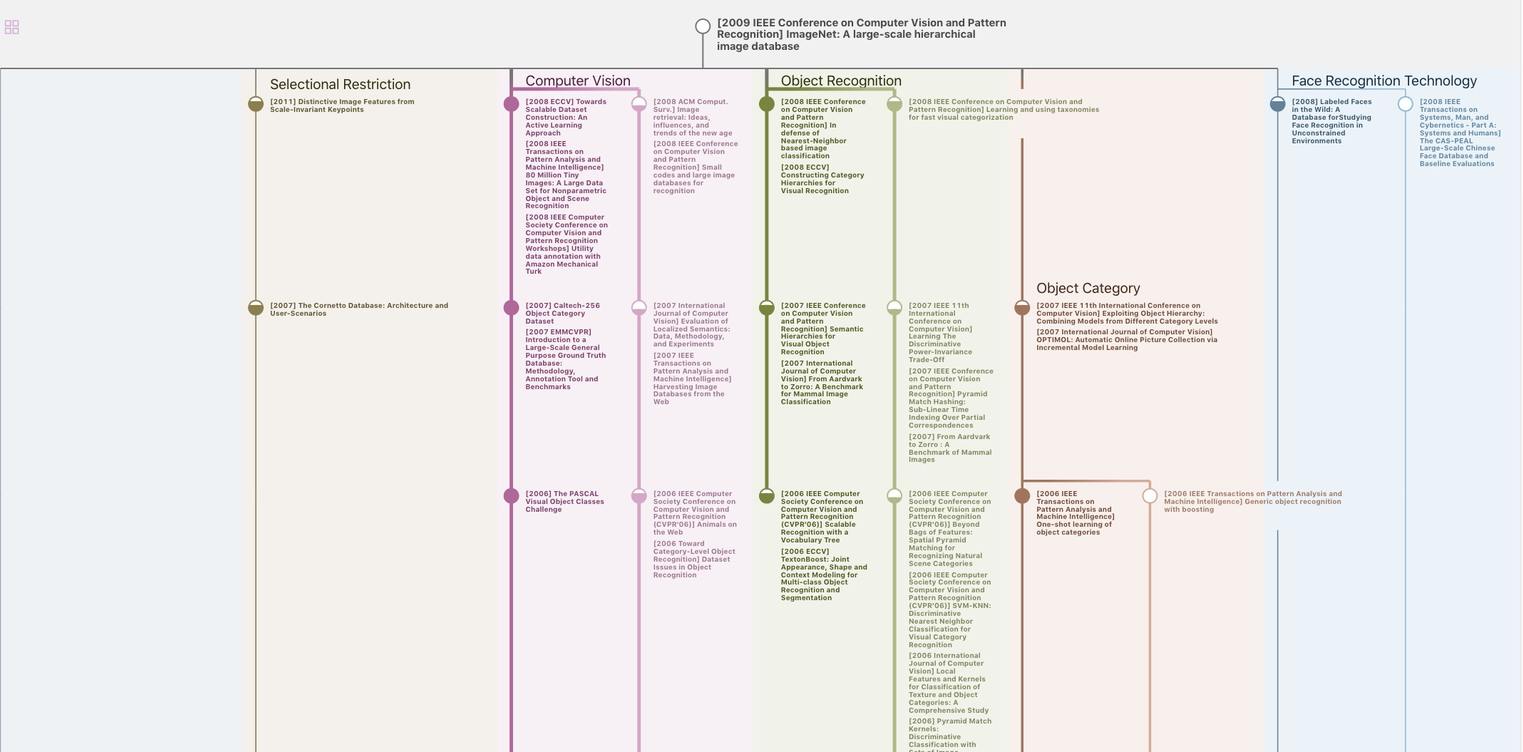
生成溯源树,研究论文发展脉络
Chat Paper
正在生成论文摘要