Integrating Zhuhai-1 Hyperspectral Imagery With Sentinel-2 Multispectral Imagery to Improve High-Resolution Impervious Surface Area Mapping
IEEE JOURNAL OF SELECTED TOPICS IN APPLIED EARTH OBSERVATIONS AND REMOTE SENSING(2022)
摘要
Mapping impervious surface area (ISA) in an accurate and timely manner is essential for a variety of fields and applications, such as urban heat islands, hydrology, waterlogging, and urban planning and management. However, the large and complex urban landscapes pose great challenges in retrieving ISA information. Spaceborne hyperspectral (HS) remote sensing imagery provides rich spectral information with short revisit cycles, making it an ideal data source for ISA extraction from complex urban scenes. Nevertheless, insufficient single-band energy, the involvement of modulation transfer function (MTF), and the low signal-to-noise ratio (SNR) of spaceborne HS imagery usually result in poor image clarity and noises, leading to inaccurate ISA extraction. To address this challenge, we propose a new deep feature fusion-based classification method to improve 10 m resolution ISA mapping by integrating Zhuhai-1 HS imagery with Sentinel-2 multispectral (MS) imagery. We extract deep features that include spectral and spatial features, respectively, from MS and HS imagery via a 2-D convolutional neural network (CNN), aiming to increase feature diversity and improve the model's recognition capability. The Sentinel-2 imagery is used to enhance the spatial information of the Zhuhai-1 HS image, improving the urban ISA retrieval by reducing the impact of noises. By combining the deep spatial features and deep spectral features, we obtain joint spatial-spectral features, leading to high classification accuracy and robustness. We test the proposed method in two highly urbanized study areas that cover Foshan city and Wuhan city, China. The results reveal that the proposed method obtains an overall accuracy of 96.72% and 96.75% in the two study areas, 18.78% and 8.66% higher than classification results with only HS imagery as input. The final ISA extraction overall accuracy is 95.42% and 95.50% in the two study areas, the highest among the comparison methods.
更多查看译文
关键词
Feature extraction,Convolutional neural networks,Signal to noise ratio,Spatial resolution,Support vector machines,Hyperspectral imaging,Fuses,Convolutional neural network (CNN),feature fusion,impervious surface area (ISA) mapping,sentinel-2 imagery,Zhuhai-1 spaceborne hyperspectral (HS) imagery
AI 理解论文
溯源树
样例
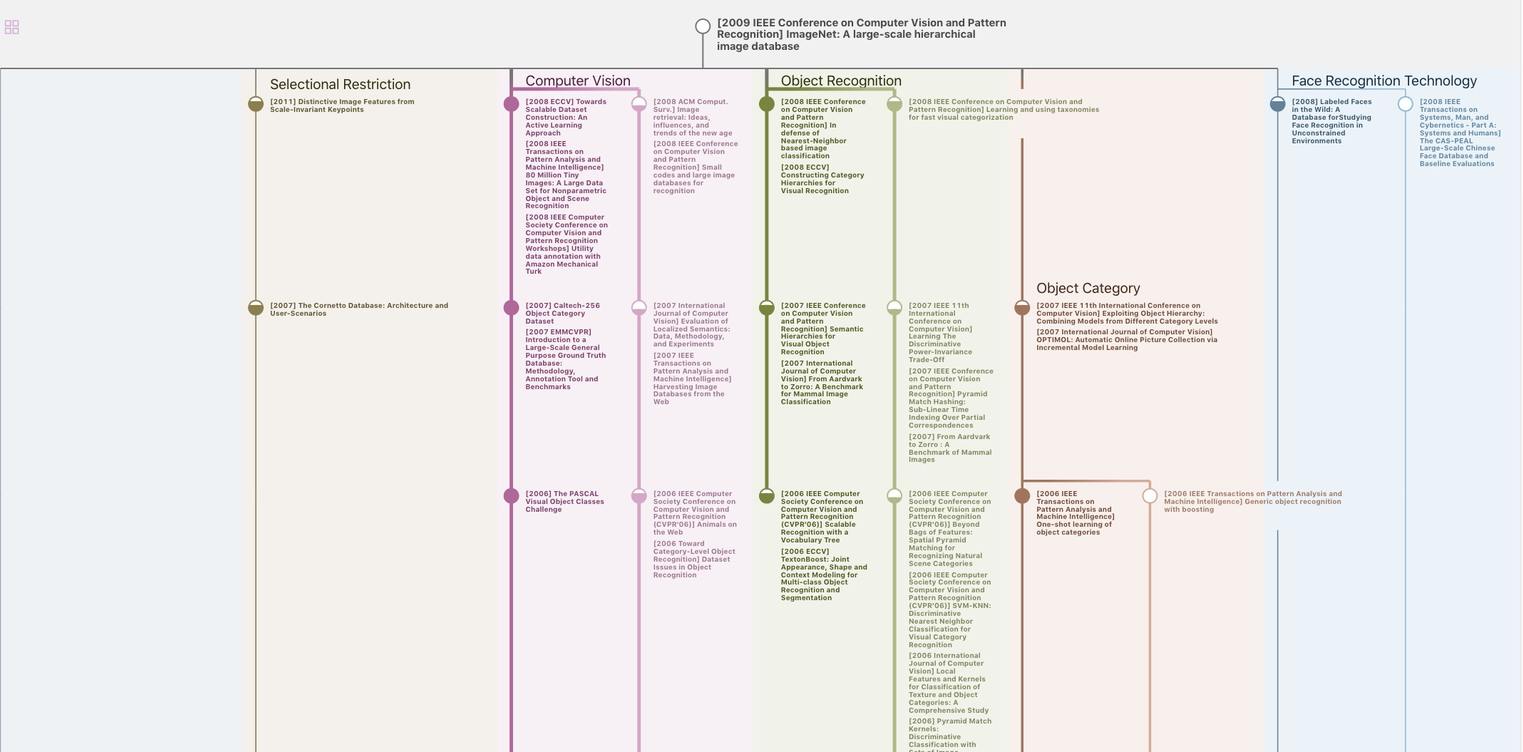
生成溯源树,研究论文发展脉络
Chat Paper
正在生成论文摘要