Enhanced Efficient YOLOv3-tiny for Object Detection.
CSAI(2021)
摘要
Lightweight object detection models have great application prospects in resource-restricted scenarios such as mobile and embedded devices, and have been a hot topic in the computer vision community. However, most existing lightweight object detection methods show poor detection accuracy. In this study, we put forward a lightweight objection detection model named Enhanced-YOLOv3-tiny to improve the detection accuracy and reduce the model complexity at the same time. In Enhanced-YOLOv3-tiny, we propose a new backbone named GhostDarkNet based on DarkNet53 and Ghost Module for decreasing the model parameters, which helps to get more representative features compared with YOLOv3-tiny. Furthermore, we put forward a new Multiscale Head, which adds three more heads and includes Ghost Module in each head to fuse multi-scale features. Experiments on the Priority Research Application dataset from the real scenes in driving show that the proposed Enhanced-YOLOv3-tiny outperforms the state-of-the-art YOLOv3-tiny by 8.4% improvement in AP metric and decreases parameters from 8.8M to 3.9M, demonstrating the application potentials of our proposed method in resource-constrained scenarios.
更多查看译文
AI 理解论文
溯源树
样例
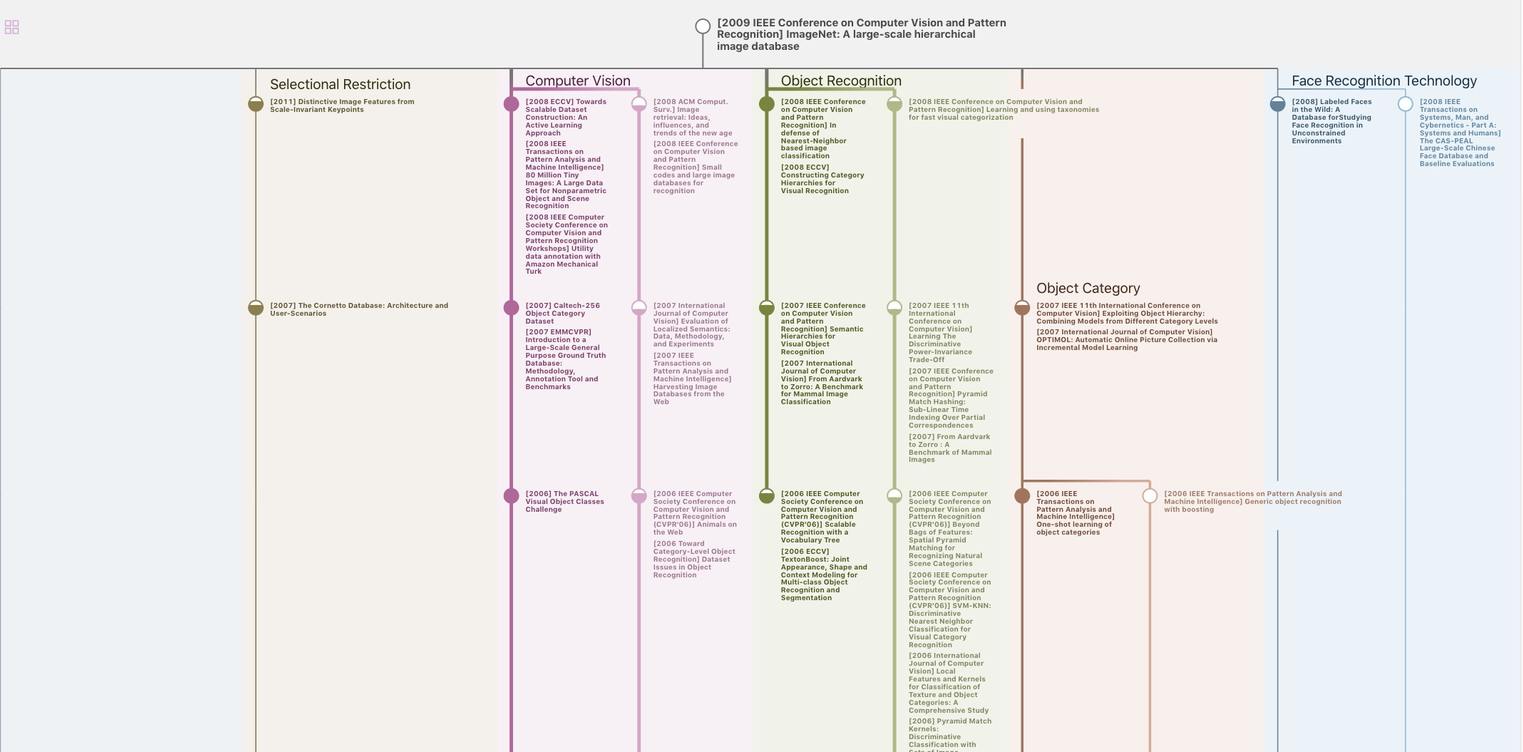
生成溯源树,研究论文发展脉络
Chat Paper
正在生成论文摘要