Multi-omics fusion for prediction of response to neoadjuvant therapy in breast cancer with external validation
Cancer Research(2022)
摘要
Abstract Predicting pathological completer response (pCR) to neoadjuvant chemotherapy (NAC) in breast cancer is crucial to stratify patients and individualize treatments early. Radiological information from Magnitude resonance imaging (MRI) could reflect the whole tumor content from a macroscopic scale and pathological information from biopsy whole slide images (WSIs) contain essential information about the tumor cells' living condition from a microscopic scale. However, few studies focused on predicting pCR to NAC in breast cancer by fusing radiological and pathological information. We recruited 318 patients with primary invasive breast cancer between January 2015 and December 2019 from three hospitals. The patients from Guangdong Provincial People’s Hospital (n=175) were selected as the primary cohort (PC). Others (n=143) collected from Foshan First People's Hospital (n=115) and Shantou Central Hospital (n=28) were used as external validation cohort (VC). All patients were with axial T2-weighted MRI (T2WI), diffusion-weighted imaging (DWI, b value=0 and 1000s/mm2 or 800s/mm2), and digitalized biopsy WSIs. Quantitive radiomic features were extracted from T2WI and apparent diffusion coefficients (ADC) maps derived from DWI. For pathomic features, we first tiled the WSIs with the size of 512×512 (0.25 mpp) and 256×256 (0.25 mpp) from two scales. Then we selected the tiles with the region of cells by our pretrained deep learning model. The output of the last convolution layer of the deep learning model was extracted as the patch-level features. The patch-level features were aggregated into patient-level features using bag of words (BoW) methods. We firstly investigated the predictive value of mono-omic using T2WI features, ADC features, and pathomic features separately using Light Gradient Boosting Machine (LGBM) in the PC with the reference of VC. Then we combined the T2WI, ADC, and pathomic features as multi-omics features and constructed a pCR prediction model using LGBM. Moreover, We combined multi-omics features with clinical characteristics including estrogen receptor (ER) status, progesterone receptor (PR) status, Ki67 index, and human epidermal growth factor 2 (HER2) status, tumor stage (cT), and lymph node stage (cN) for better performance. The area under the curve (AUC) of the receiver operating characteristic (ROC) curve was selected as the evaluation metric. In the mono-omic results, the model showed the lowest AUC (PC: 0.787, VC: 0.675) in the pathomic features and the highest AUC (PC: 0.989, VC: 0.725) in the T2WI modality. After fusing the three modality features as multi-omics features, the prediction performance increased compared to all mono-omic performances (PC: 0.833, VC: 0.745). Also, by adding clinical characteristics, the best performance was achieved with an AUC of 0.861 in the PC and 0.833 in the VC. The detailed AUC for each modality was shown in Table 1. Our study revealed that the pCR predictive performance of multi-omics features was better compared to mono-omic features. Although T2WI features in our study were better than the multi-omics features for pCR prediction in the PC, it suffered the overfitting problem in the VC. The multi-omics features could reflect the tumor properties from both macroscopic and microscopic scales, which could generalize the model and showed good predictive performance in all cohorts. Moreover, the predictive performance could further be boosted by combining clinical characteristics. Table 1.The AUC of the prediction of pathological complete response in the PC and VCModalityPrimary cohort(n=175)Validation cohort (n=143)T2WI0.9890.725ADC0.8560.692WSI0.7870.675Fusion0.8330.745Fusion+Clinical characteristics0.8610.833 Citation Format: Bao Li, Teng Zhu, Zhenyu Liu, Kun Wang, Jie Tian. Multi-omics fusion for prediction of response to neoadjuvant therapy in breast cancer with external validation [abstract]. In: Proceedings of the 2021 San Antonio Breast Cancer Symposium; 2021 Dec 7-10; San Antonio, TX. Philadelphia (PA): AACR; Cancer Res 2022;82(4 Suppl):Abstract nr P2-12-11.
更多查看译文
AI 理解论文
溯源树
样例
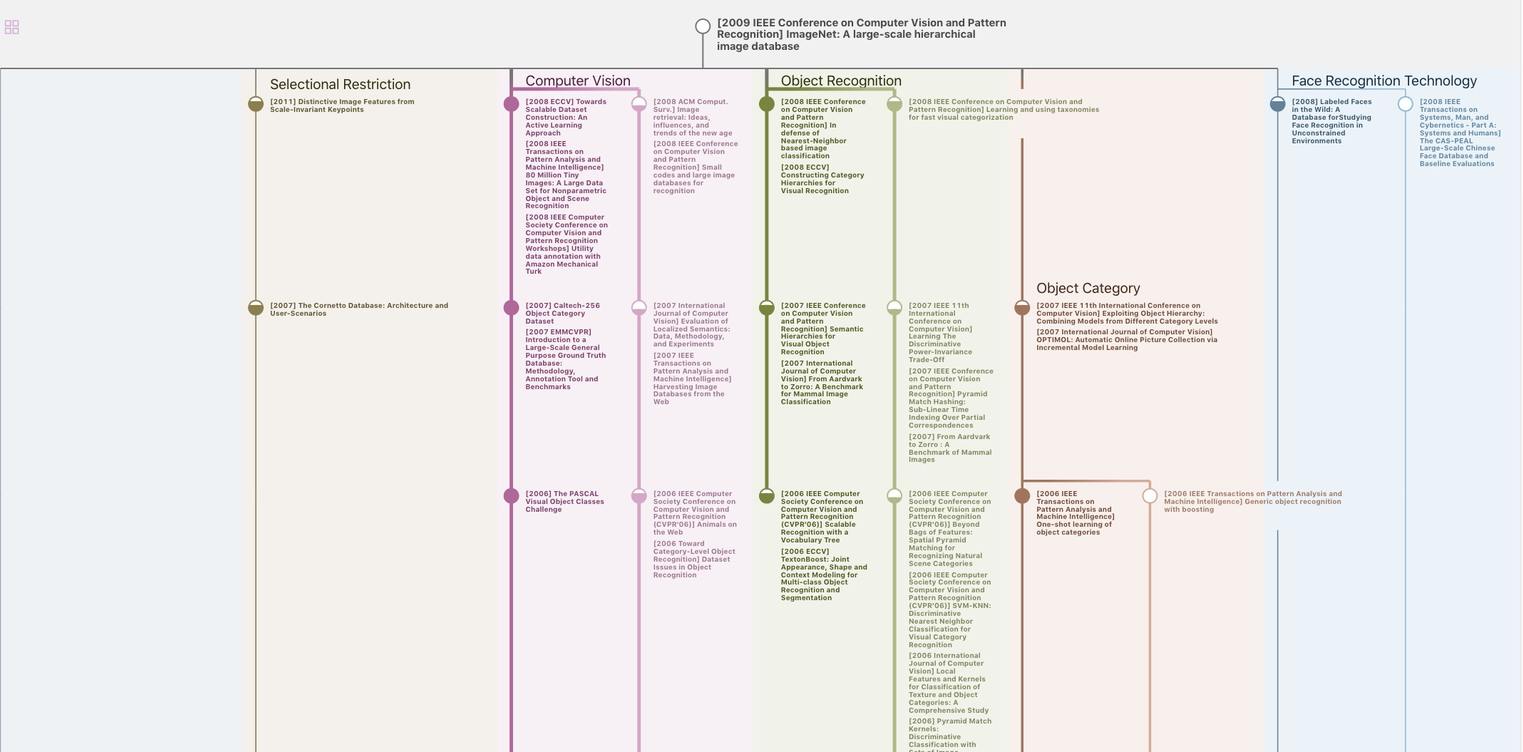
生成溯源树,研究论文发展脉络
Chat Paper
正在生成论文摘要