Optimized visually meaningful image embedding strategy based on compressive sensing and 2D DWT-SVD
Multimedia Tools and Applications(2022)
摘要
This paper presents an optimized embedding strategy for visually meaningful embedded image based on compressive sensing (CS), two dimensional discrete wavelet transform (2D DWT) and singular value decomposition (SVD). The embedding strategy consists of four main processes: keys generation; compress the original signal; bit-level image encryption; information hiding. Firstly, the Mahalanobis distance between the original image and the host image is used as the parameter of the SHA-512, and then iterate two chaotic systems with hash values to generate key streams. Then, the components of the plain image are sparsely represented by the optimal direction method, and liner measurement these sparse matrices according to the theory of CS, which can achieve compressing and encrypting simultaneously. Thirdly, the bit-level XOR operation is performed on the compressed sensing image by shuffle and diffusion simultaneously. Finally, decomposing the encrypted image and the carrier image by 2D DWT, and modifying sub-bands of the host image with encrypted sub-bands based on SVD, one can obtain a visually meaningful embedded image, which peak signal-to-noise ratio (PSNR) is 0.08% higher than some traditional optimal algorithms. In particular, the size of the plain image is larger than the host image, which requires stronger embedding capacity. In order to enhance the ability to the defense against plaintext attacks, key streams of two chaotic systems are highly correlated with original images and host images. Moreover, the method of compression and encryption can greatly reduce storage space and process time. Simulation results demonstrate the security and effectiveness of the proposed algorithm.
更多查看译文
关键词
Compressive sensing, Singular value decomposition, Chaotic system, Two dimensional discrete wavelet transform, Visually meaningful embedded image
AI 理解论文
溯源树
样例
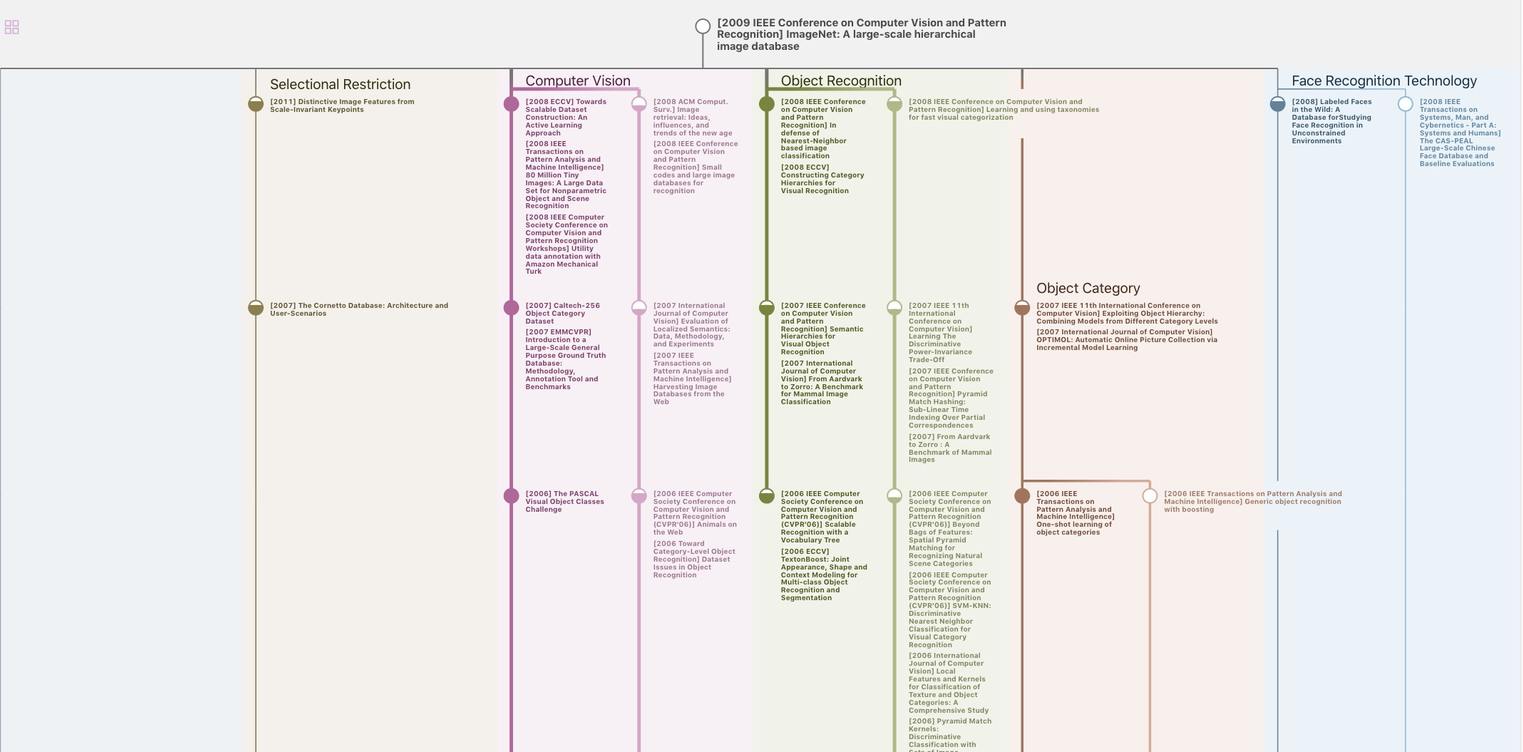
生成溯源树,研究论文发展脉络
Chat Paper
正在生成论文摘要