Impacts of Pollution Heterogeneity on Population Exposure in Dense Urban Areas Using Ultra-Fine Resolution Air Quality Data
Journal of Environmental Sciences/Journal of environmental sciences(2023)
摘要
Traditional air quality data have a spatial resolution of 1 km or above, making it challenging to resolve detailed air pollution exposure in complex urban areas. Combining urban morphology, dynamic traffic emission, regional and local meteorology, physicochemical transformations in air quality models using big data fusion technology, an ultra-fine resolution modeling system was developed to provide air quality data down to street level. Based on one-year ultra-fine resolution data, this study investigated the effects of pollution heterogeneity on the individual and population exposure to particulate matter (PM2.5 and PM10), nitrogen dioxide (NO2), and ozone (O3) in Hong Kong, one of the most densely populated and urbanized cities. Sharp fine-scale variabilities in air pollution were revealed within individual city blocks. Using traditional 1 km average to represent individual exposure resulted in a positively skewed deviation of up to 200% for high-end exposure individuals. Citizens were disproportionally affected by air pollution, with annual pollutant concentrations varied by factors of 2 to 5 among 452 District Council Constituency Areas (DCCAs) in Hong Kong, indicating great environmental inequities among the population. Unfavorable city planning resulted in a positive spatial coincidence between pollution and population, which increased public exposure to air pollutants by as large as 46% among districts in Hong Kong. Our results highlight the importance of ultra-fine pollutant data in quantifying the heterogeneity in pollution exposure in the dense urban area and the critical role of smart urban planning in reducing exposure inequities.
更多查看译文
关键词
Particulate matter,Nitrogen dioxide,Ozone,Pollution heterogeneity,Urban area
AI 理解论文
溯源树
样例
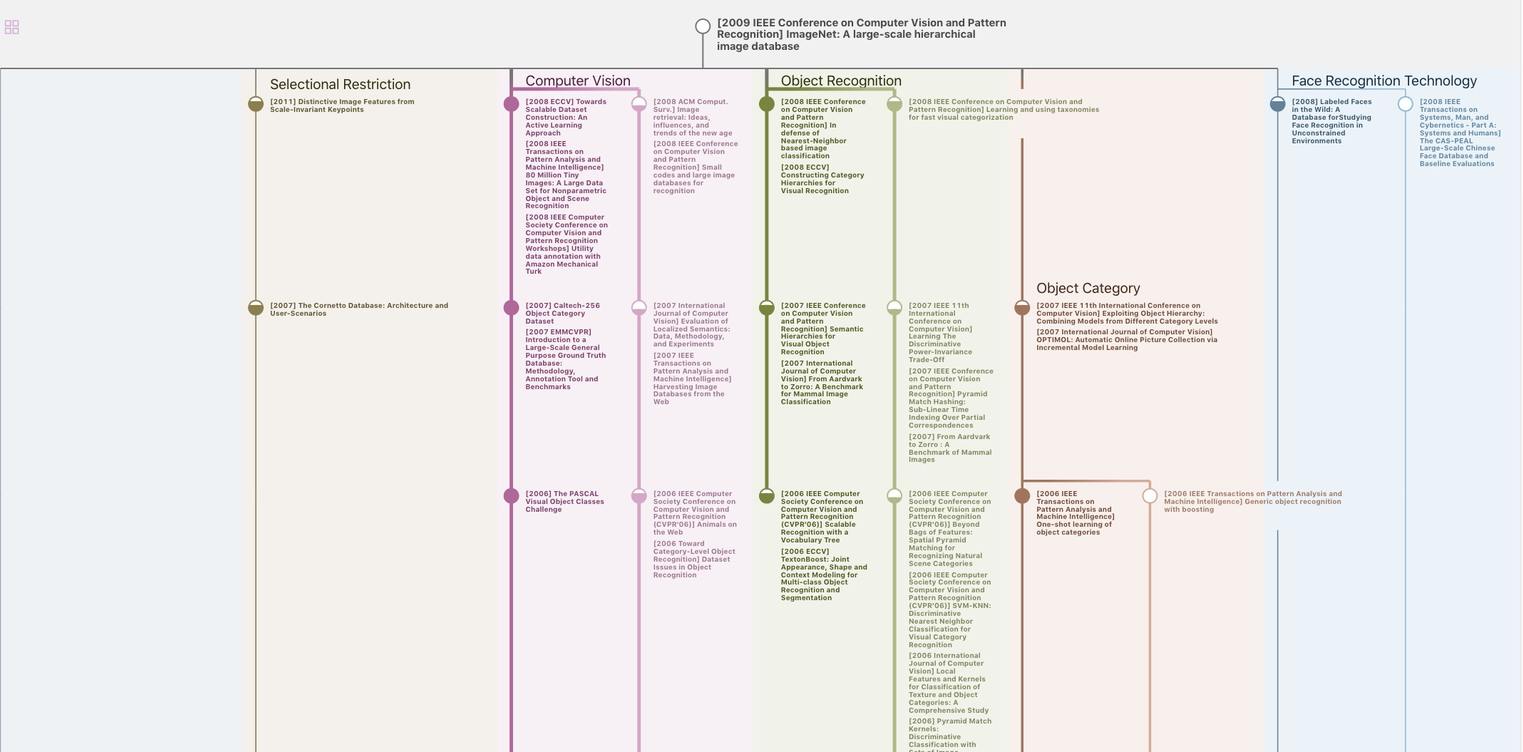
生成溯源树,研究论文发展脉络
Chat Paper
正在生成论文摘要