Fully Unsupervised Person Re-Identification by Enhancing Cluster Samples
2021 the 7th International Conference on Communication and Information Processing (ICCIP)(2021)
摘要
Fully unsupervised person re-identification aims to train a discriminative model with unlabeled person images. Most existing methods first generate pseudo labels by clustering image features (convolutional features) and then fine-tune the convolutional neural network (CNN) with pseudo labels. However, these methods are greatly limited by the quality of the pseudo labels. In this paper, a cluster sample enhancement method is introduced to increase the reliability of pseudo-label samples to facilitate the CNN training. Specifically, when generating pseudo labels, only the samples with high-confidence pseudo-label predictions are selected. In addition, to enhance the selected samples for training, two different image transformations are adopted and coupled with specific-design loss functions to boost the model performance. Experiments demonstrate the effectiveness of the proposed method. Concretely, the proposed method achieves 87.1% rank-1 and 70.2% mAP accuracy on Market-1501.
更多查看译文
关键词
cluster samples,re-identification
AI 理解论文
溯源树
样例
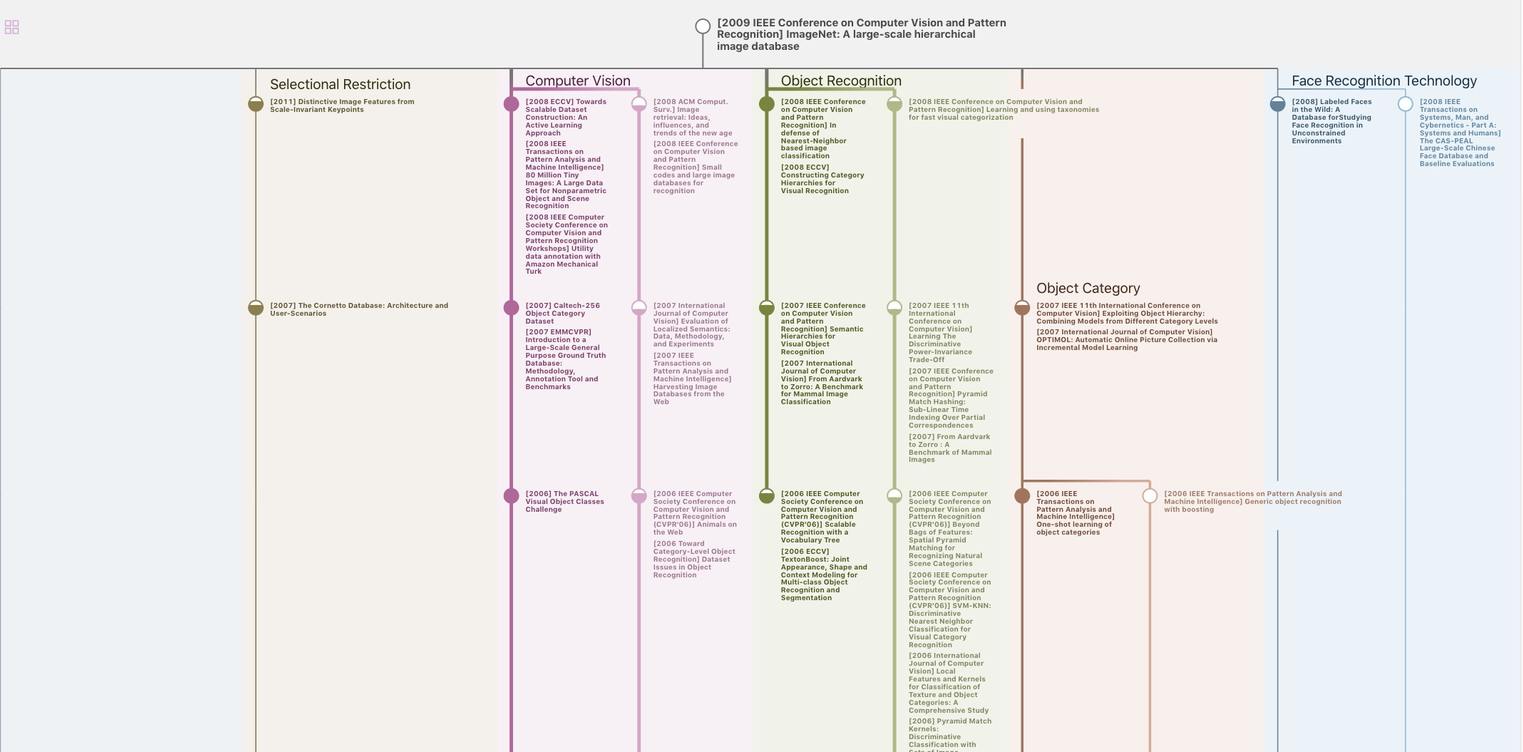
生成溯源树,研究论文发展脉络
Chat Paper
正在生成论文摘要