HashFormer: Vision Transformer Based Deep Hashing for Image Retrieval
IEEE SIGNAL PROCESSING LETTERS(2022)
摘要
Deep image hashing aims to map an input image to compact binary codes by deep neural network, to enable efficient image retrieval across large-scale dataset. Due to the explosive growth of modern data, deep hashing has gained growing attention from research community. Recently, convolutional neural networks like ResNet have dominated in deep hashing. Nevertheless, motivated by the recent advancements of vision transformers, we propose a pure transformer-based framework, called as HashFormer, to tackle the deep hashing task. Specifically, we utilize vision transformer (ViT) as our backbone, and treat binary codes as the intermediate representations for our surrogate task, i.e., image classification. In addition, we observe that the binary codes suitable for classification are sub-optimal for retrieval. To mitigate this problem, we present a novel average precision loss, which enables us to directly optimize the retrieval accuracy. To the best of our knowledge, our work is one of the pioneer works to address deep hashing learning problems without convolutional neural networks (CNNs). We perform comprehensive experiments on three widely-studied datasets: CIFAR-10, NUSWIDE and ImageNet. The proposed method demonstrates promising results against existing state-of-the-art works, validating the advantages and merits of our HashFormer.
更多查看译文
关键词
Transformers,Binary codes,Task analysis,Training,Image retrieval,Feature extraction,Databases,Binary embedding,image retrieval
AI 理解论文
溯源树
样例
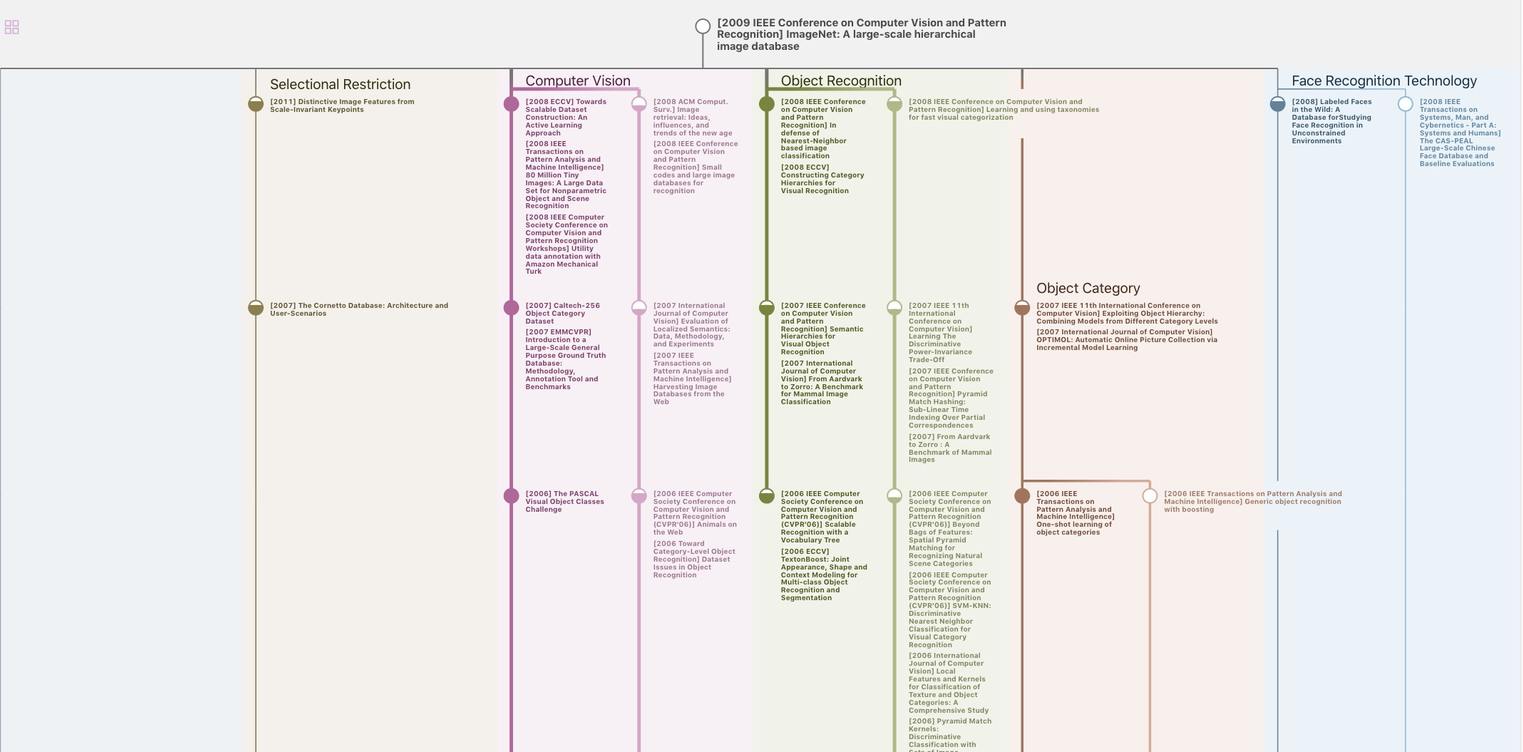
生成溯源树,研究论文发展脉络
Chat Paper
正在生成论文摘要