Deep Learning for Multi‐Timescales Pacific Decadal Oscillation Forecasting
Geophysical Research Letters(2022)
摘要
Variations in the Pacific decadal oscillation (PDO) can influence marine ecosystems and regional climate phenomena. Accurate and long-term forecasts of the PDO are therefore crucial for marine governance. This paper presents a novel seasonal gated recurrent unit (SGRU) model, based on deep learning, for forecasting the PDO at multiple time scales. The model first decomposes the complex and nonlinear PDO index time series into three separate components, each retaining a distinct pattern of PDO. Next, a three-pathway GRU model is constructed to model and forecast the PDO index. A test applying the SGRU model to the period from 1979 to 2020 demonstrates its superiority over eight state-of-the-art models in PDO forecasting. Additionally, the SGRU model can flexibly produce high-performance forecasts at multiple time scales. In view of that physical and dynamical models rely on clear evolutionary mechanisms, the SGRU model overcomes the complexities of these models. Plain Language Summary We apply deep learning methods, for the first time, to directly forecast the Pacific decadal oscillation (PDO) index at both monthly and annual time scales. Using a data-driven seasonal gated recurrent unit (SGRU) model, we forecast the PDO index flexibly, without relying on information about the dynamics of the evolution of the PDO. In a comparative test using a data set for the years 1979-2020, the performance of the SGRU model is far superior to that of the state-of-art methods in consecutive forecasting for lead times of 6 months and 3 years.
更多查看译文
AI 理解论文
溯源树
样例
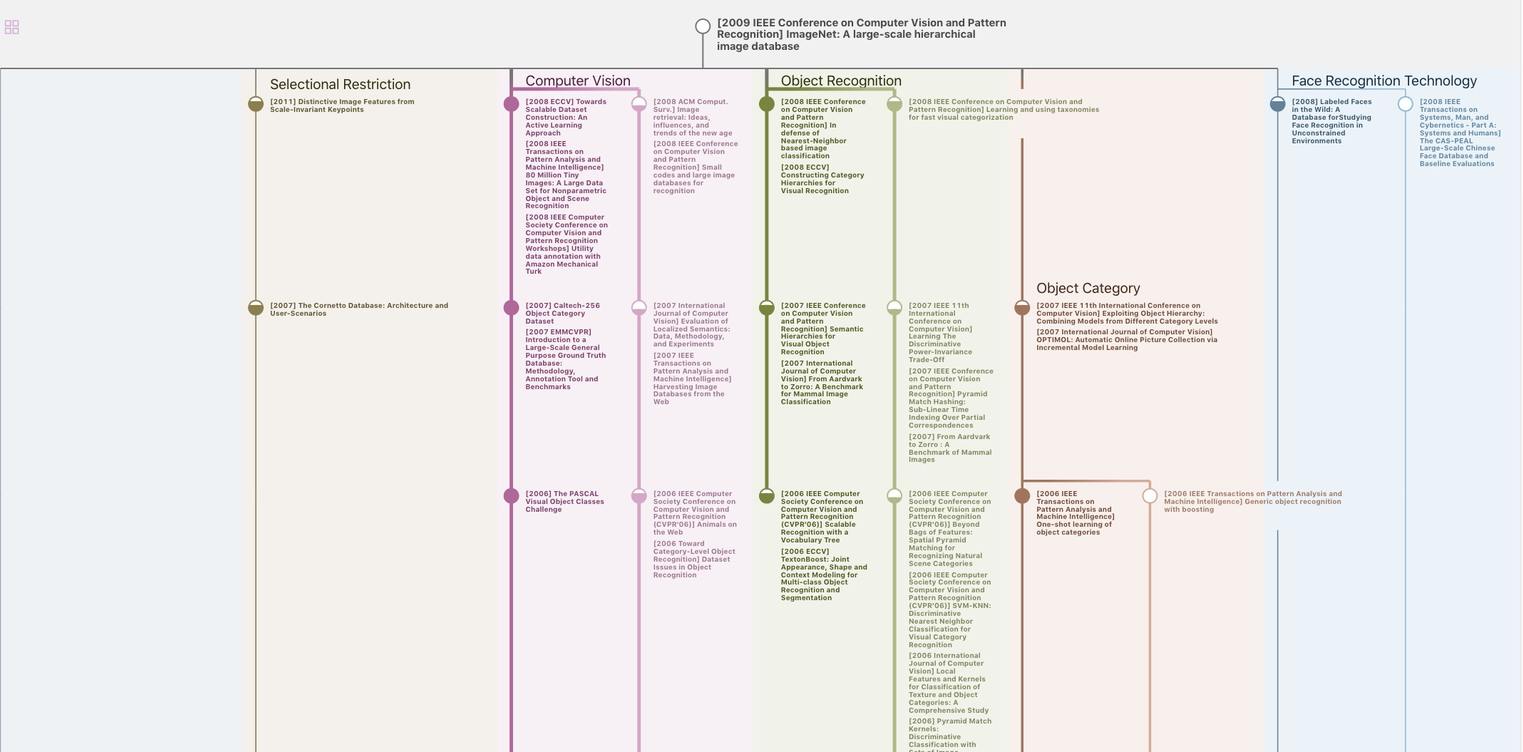
生成溯源树,研究论文发展脉络
Chat Paper
正在生成论文摘要