Interpreting Deep Neural Networks for Medical Imaging Using Concept Graphs
AI for Disease Surveillance and Pandemic IntelligenceStudies in Computational Intelligence(2022)
摘要
The black-box nature of deep learning models prevents them from being completely trusted in domains like biomedicine. Most explainability techniques do not capture the concept-based reasoning that human beings follow. In this work, we attempt to understand the behavior of trained models that perform image processing tasks in the medical domain by building a graphical representation of the concepts they learn. Extracting such a graphical representation of the model’s behavior on an abstract, higher conceptual level would help us to unhigher conceptual level would help us to unravel the steps taken by the model for predictions. We show the application of our proposed implementation on two biomedical problems brain tumor segmentation and fundus image classification. We provide an alternative graphical representation of the model by formulating a concept level graph as discussed above, and find active inference trails in the model. We work with radiologists and ophthalmologists to understand the obtained inference trails from a medical perspective and show that medically relevant concept trails are obtained which highlight the hierarchy of the decision-making process followed by the model. Our framework is available at https://github.com/koriavinash1/
更多查看译文
关键词
deep neural networks,medical imaging,graphs,neural networks
AI 理解论文
溯源树
样例
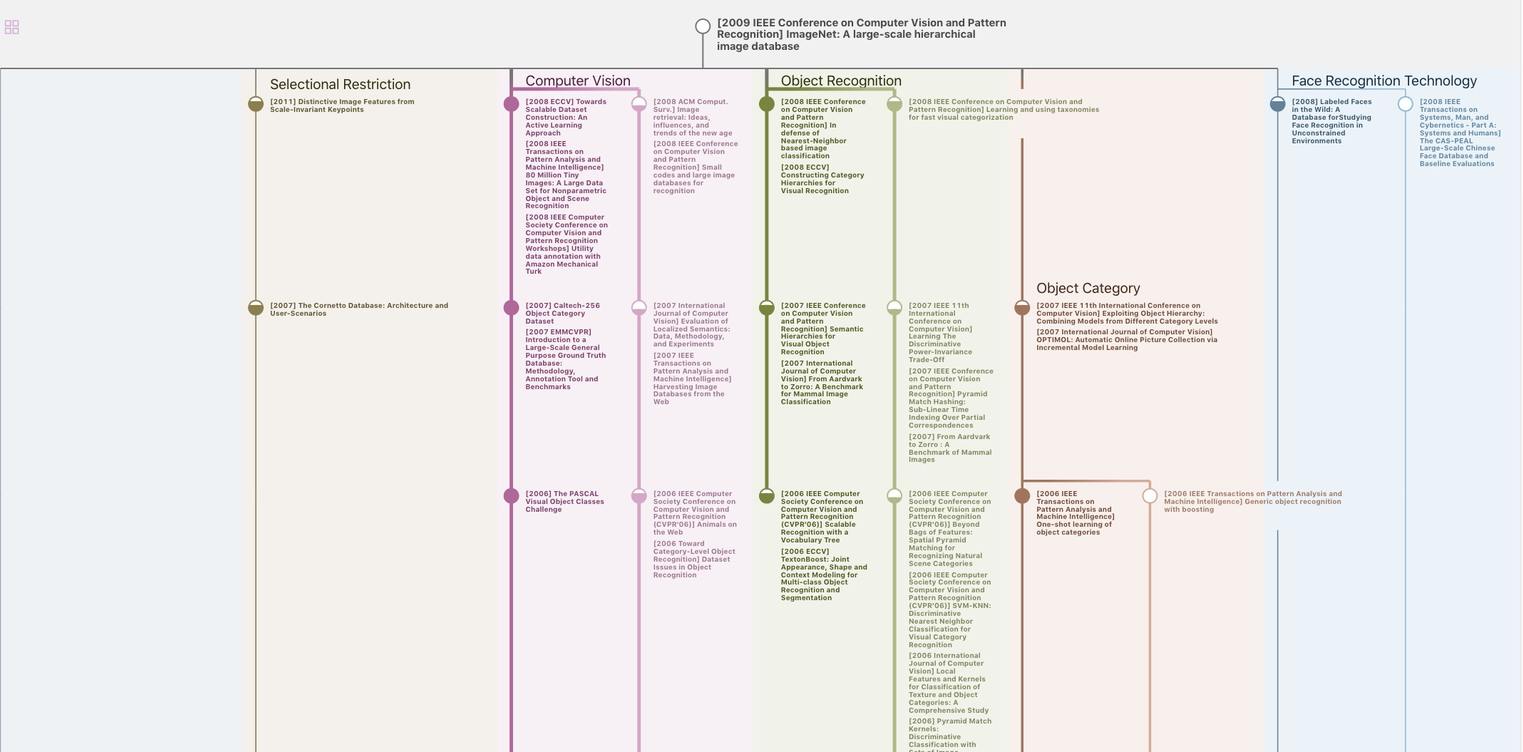
生成溯源树,研究论文发展脉络
Chat Paper
正在生成论文摘要