BlindNet backdoor: Attack on deep neural network using blind watermark
Multimedia Tools and Applications(2022)
摘要
Deep neural network (DNNs) provide excellent performance in image recognition, speech recognition, video recognition, and pattern analysis. However, DNNs are vulnerable to backdoor attacks. A backdoor attack allows a DNN to correctly recognize normal data that do not contain a specific trigger but induces it to incorrectly recognize data that do contain the trigger. An advantage of the backdoor attack is that the attacker can determine the time of attack by using a specific trigger. In this paper, we propose a blind-watermark backdoor method whose results are imperceptible to humans. Unlike existing methods, the proposed method avoids the human detectability of the backdoor sample attack by making the trigger invisible. In this method, a blind-watermarked sample is generated by inserting a trigger consisting of a specific image in a frequency band into input data by using a Fourier transform. By additionally training on the blind-watermarked sample during the training process, the target model learns to incorrectly classify any sample with the specific watermark. For testing, we used the CIFAR10 dataset and the Tensorflow machine learning library. In the experiment, when the proportion of blind-watermarked samples in the training data was 10%, the proposed method resulted in 88.9% classification accuracy by the model on the original samples and a 99.3% attack success rate via training with the blind-watermarked samples.
更多查看译文
关键词
Deep neural network,Poisoning attack,Backdoor attack,Machine learning security,Causative attack
AI 理解论文
溯源树
样例
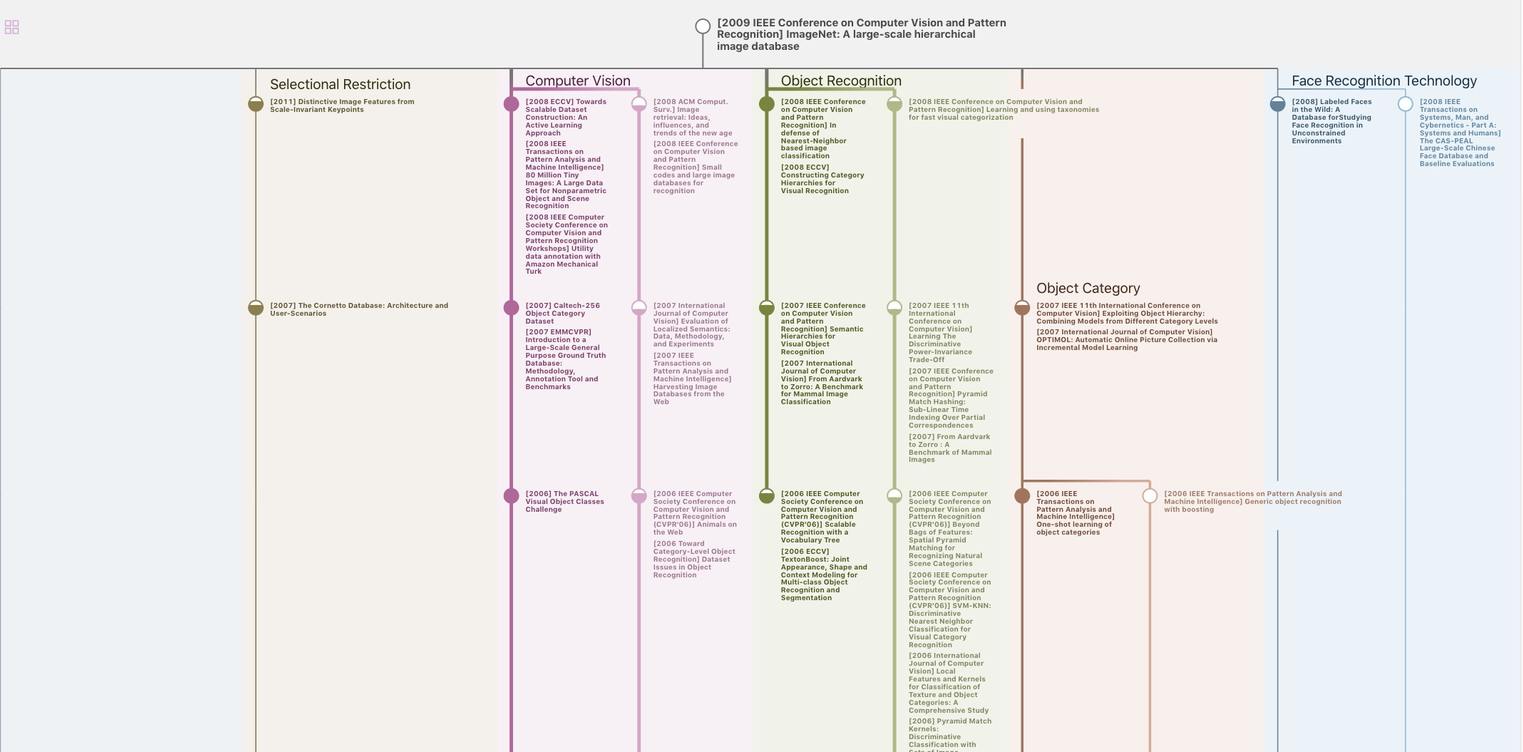
生成溯源树,研究论文发展脉络
Chat Paper
正在生成论文摘要