Predictive Market Making via Machine Learning
Operations Research Forum(2022)
摘要
Market making (MM) is an important means of providing liquidity to the stock markets. Recent research suggests that reinforcement learning (RL) can improve MM significantly in terms of returns. In the latest work on RL-based MM, the reward is a function of equity returns, calculated based on its current price, and the inventory of MM agent. As a result, the agent’s return is maximised and liquidity is provided. If the price movement is known and this information is optimally utilised, there is potential that the MM agent’s return can be further improved. Important questions are, how to predict stock price movement, and how to utilise such prediction? In this paper, we introduce the concept of predictive market marking (PMM) and present our method for PMM, which comprises a RL-based MM agent and a deep neural network (DNN)-based price predictor. A key component of PMM is the consolidated price equation (CPE), which amalgamates an equity’s present and predicted market prices into a consolidated price, which is used to generate ask and bid quotes that reflect both current price and future movement. Our PMM method is evaluated against the state-of-the-art (RL-based MM) and a traditional MM method, using ten stocks and three exchange traded funds (ETFs). Out-of-sample backtesting showed that our PMM method outperformed the two benchmark methods.
更多查看译文
关键词
Market making,Reinforcement learning,Deep neural network,Asset price prediction
AI 理解论文
溯源树
样例
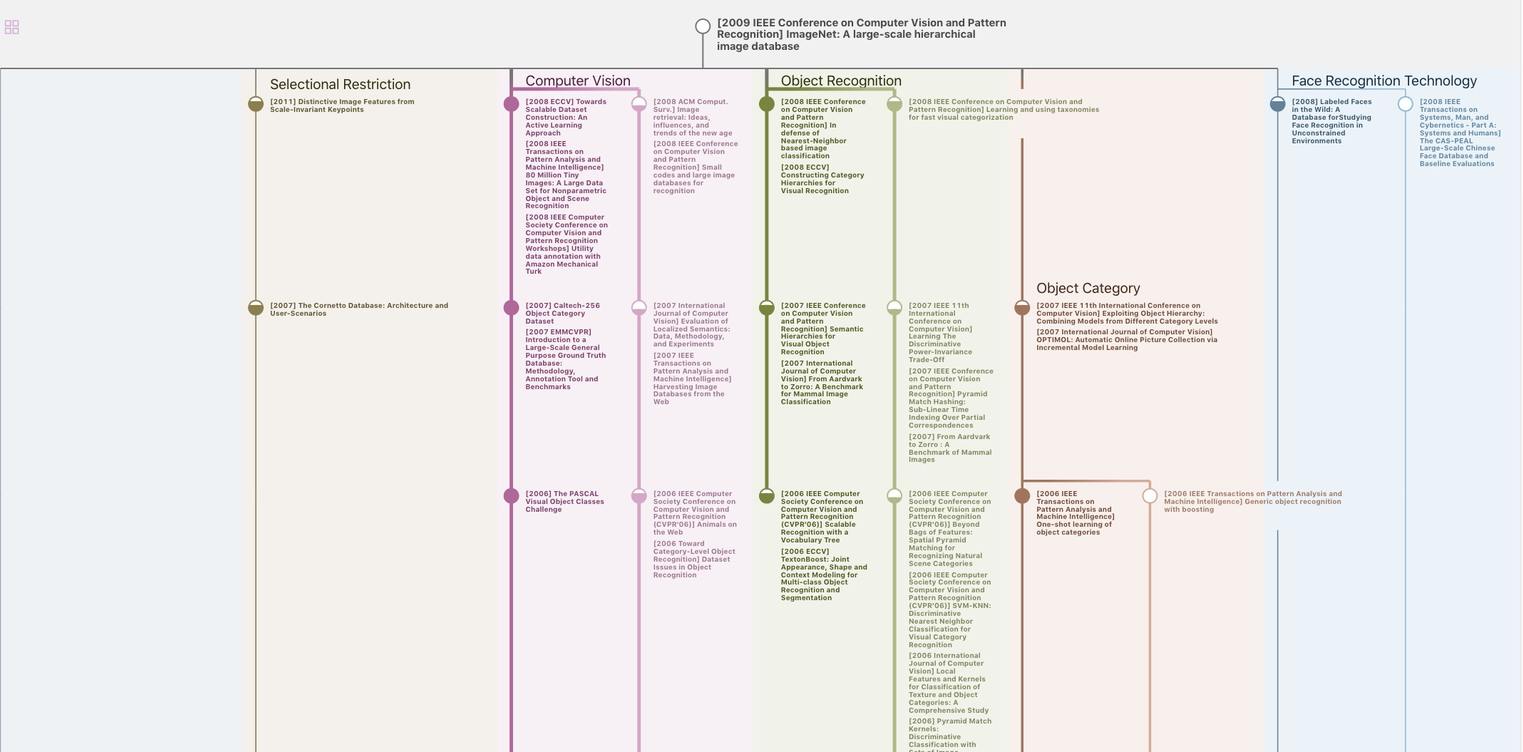
生成溯源树,研究论文发展脉络
Chat Paper
正在生成论文摘要