Surrogate-based optimization based on the probability of feasibility
Structural and Multidisciplinary Optimization(2021)
摘要
Surrogate models can be used to evaluate computationally expensive functions in optimization of nonlinear problems. The models are able to identify areas of interest in a multidimensional design space driven by methods of searching and evaluating new locations in a sparse design space. The use of constraints based on surrogates complicates the optimization problem as the uncertainty within the design space influences both the objective and constraint functions. While some optimization methods consider the mean prediction for the constraints, several methods have been reported on ways to include the uncertainty into the constraints. One way is to use a probability of feasibility to account for the mean prediction and its uncertainty. In this work, this approach is explored to evaluate its feasibility and compared to other alternate methods documented in the literature. Computationally efficient and reliable results were obtained for the mathematical test functions evaluated. The same advantageous trend could not be observed for more complex engineering applications since convergence was not reached within the prescribed computational cost by either this new approach or the established one. Nevertheless, more feasible designs were generated when the probability of feasibility was included in the objective function. For an airfoil drag minimization problem with aerodynamic and geometric constraints, improvements of ∼ 70
更多查看译文
关键词
Surrogate-based optimization, Adaptive sampling, Aerodynamic shape optimization, Kriging
AI 理解论文
溯源树
样例
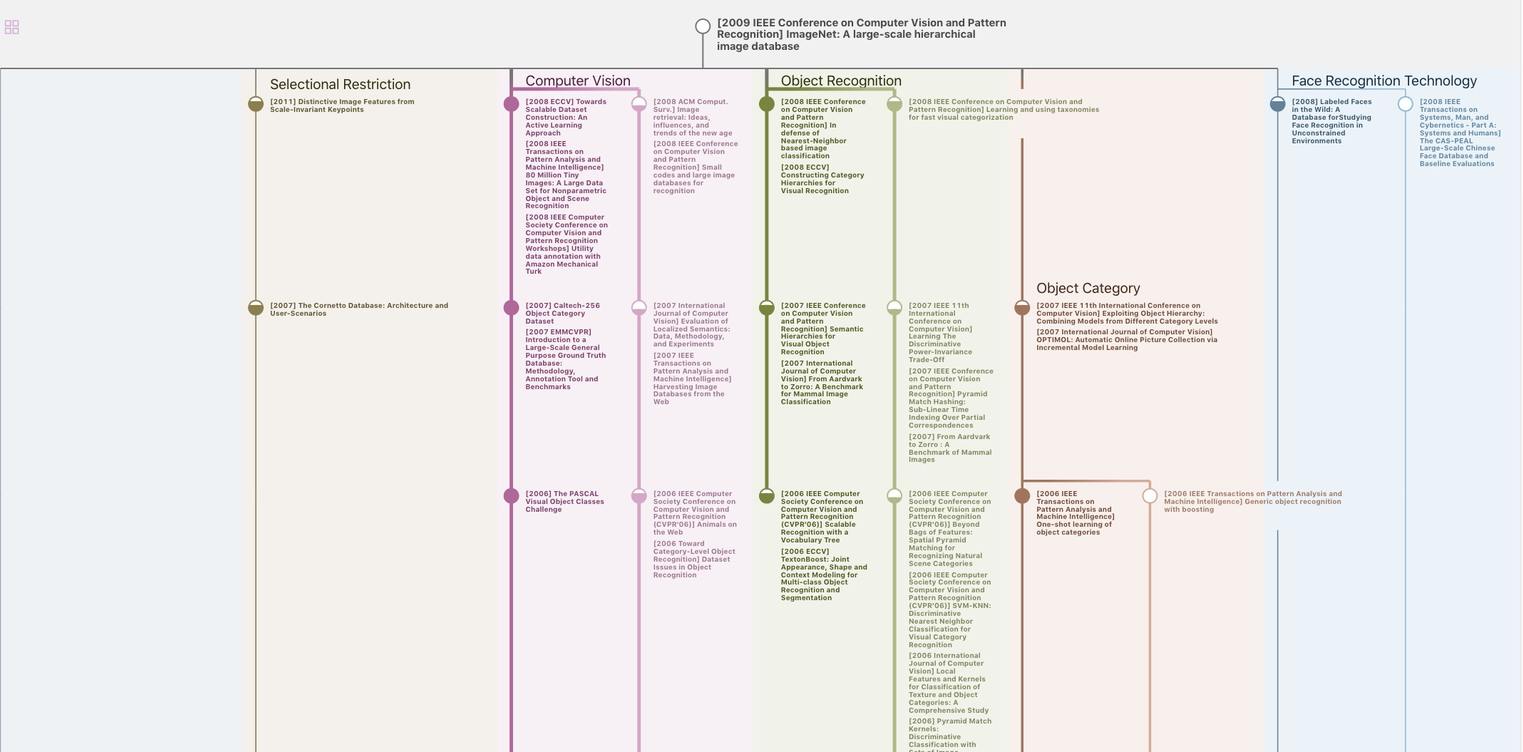
生成溯源树,研究论文发展脉络
Chat Paper
正在生成论文摘要